From bench to bot: How to use AI tools to convert notes into a draft
ChatGPT can capitalize on the highly ordered nature of scientific writing to streamline your writing process.
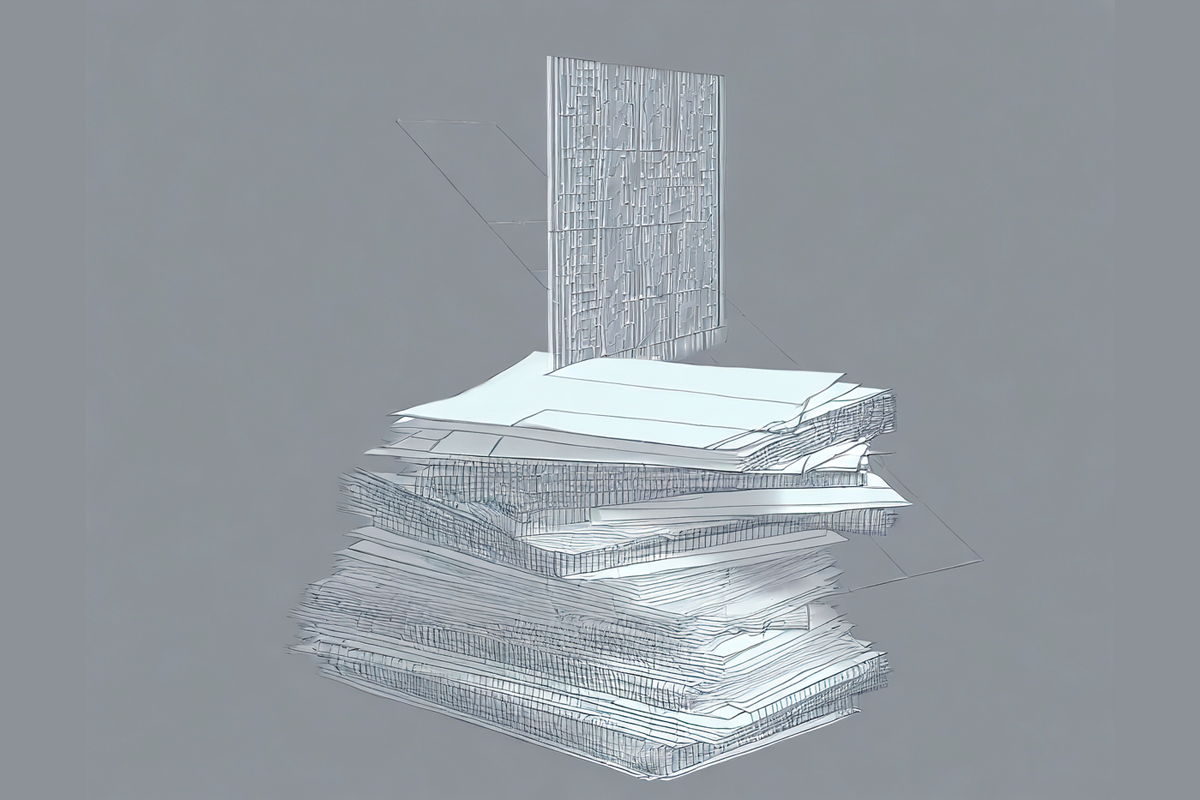
All writing is structured, but scientists have it easy in that scientific documents follow highly predictable structures. This is evident not only at the macro level — Introduction, Methods, Results and Discussion — but also at the micro level. Consider the following excerpt from the Results section of a scientific paper:
To confirm that the Hebbian term is essential for latent predictive learning (LPL) to prevent representational collapse, we simulated learning without the Hebbian term (compare equation (1)). We observed that the neuron’s activity collapses to zero firing rate as expected (Fig. 2c). Conversely, learning with the Hebbian term but without the predictive term did not result in collapse. Therefore, LPL’s Hebbian component is essential to prevent activity collapse.
Even if the science isn’t your specialty, the underlying structure is clear:
Rationale: “To confirm that the Hebbian term is essential for latent predictive learning (LPL) to prevent representational collapse … ”
Approach: “ … we simulated learning without the Hebbian term … ”
Results: “We observed that the neuron’s activity collapses to zero firing rate as expected (Fig. 2c). Conversely, learning with the Hebbian term but without the predictive term did not result in collapse.”
Interpretation: “Therefore, LPL’s Hebbian component is essential to prevent activity collapse.”
This “Rationale, Approach, Results, Interpretation” structure is a common way scientists present their findings. It’s not written on a stone tablet anywhere that you must report results that way, but it has become the norm, in part because of its clear narrative arc. The Rationale sets the stage, explaining the experimenter’s motive. The Approach and Results chronicle what happened and how, forming the main plot. Finally, the Interpretation offers the author’s gloss on what it all means — the moral of the story.
Artificial-intelligence tools such as ChatGPT can capitalize on the highly ordered nature of scientific writing to streamline your writing process. Take the typical Results paragraph. You already know from reading this column that you’ll probably want to describe the Rationale, Approach, Results and Interpretation for a given finding. Write this information out roughly, without any thought for coherence, grammar or style. Or even just speak into your device. Then, feed the rough draft (or voice transcription) into a tool such as ChatGPT, along with the underlying structure (Rationale, Approach, Results, Interpretation) as a template. This workflow will give you a pain-free rough draft, which you’ll need to check for accuracy and edit further.
To see an AI-assisted voice-to-paragraph workflow in action, let’s take an example from one of my own papers. That way, I’ll be in a good position to judge whether ChatGPT introduces inaccuracies, exaggerations or other issues — at least in principle. This paper is nearly 10 years old, after all. (As a technical note, I’m using the OpenAI web interface with GPT-4. To keep things reproducible, I’m not using any custom instructions. To avoid the effects of chat history, I’m starting a new chat for each prompt.)
Prompt:
You are writing a Results section for a scientific manuscript. Your task is to assemble the following voice transcription into a coherent paragraph. The information about the scientific experiment being described should be ordered as follows: Rationale, Approach, Results, Interpretation. Your response should retain all necessary details from the original transcription, such as numbers or specific names. If the transcription mentions a number or value but does not name it, put in a placeholder: “[INSERT VALUE HERE].” Place figure call-outs in parentheses. If a reference is mentioned, put it in parentheses at the end of the sentence. Your tone should be formal but prioritize clarity and concision.
Here is the transcript: “OK, let’s see … where to start? Alright. So there have been some studies before this. They found that the, uh, mossy fibers … they go into the, uh, the Eminentia granularis posterior, EGp for short. Where granule cells that connect to ELL. Reference Figure 1B. Mossy fibers originate from different parts of the brain and even the spinal cord. Cite relevant papers … Bell 1981, then Szabo 1979 and 1990, I think. These mossy fibers carry different types of information. There’s EOCD, proprioceptive and electrosensory signals. I think Bell talked about this in 1992 paper, and we should also cite the Kennedy paper from 2014. Oh, and ours, Sawtell in 2010. Need to keep those in mind. So our main question is, do some of these mossy fibers carry movement-related information, um, corollary discharge? To figure that out, we combined fictive preparation with extracellular recordings from putative mossy fiber axons in EGp. Bell and others mentioned this method. We found that a group of those tonically active mossy fibers showed changes in their firing rate during spontaneous movement. That’s in Figure S1. Or when there was microstimulation-evoked motor activity. About 23 out of 48 fibers showed this. That’s in Figure 2C. Then, for the fibers that had rhythmic motor activity. That was, let’s see, 19 out of 23. The rhythmic firing rate changes matched up with the motor nerve activity. There’s a correlation value here … be sure to put that in. There was also a clear link between the frequency of the mossy fiber firing rate changes and the frequency of the motor nerve bursts after smoothing. That’s shown in Figure 2D. Some fibers even showed this during spontaneous bursts. OK, finally, for some of the mossy fibers we tested with different microstimulation intensities, the frequency of their firing rate changes increased with the intensity of the microstimulation. Put in the numbers here. Those will be displayed in Figure 2E. So, in conclusion from this data, mossy fibers seem to convey motor info that’s connected to the rhythm of swimming movements.”
ChatGPT output:
Previous studies have elucidated that mossy fibers project into the Eminentia granularis posterior (EGp), where granule cells establish connections to the ELL (Figure 1B). These fibers, originating from various regions of the brain and even the spinal cord, transmit diverse types of information, including EOCD, proprioceptive, and electrosensory signals (Bell 1981; Szabo 1979, 1990; Bell 1992; Kennedy 2014; Sawtell 2010). The principal question under investigation was whether certain mossy fibers relay movement-related information, specifically corollary discharge. To address this, we employed a combination of fictive preparation and extracellular recordings from presumptive mossy fiber axons in EGp, an approach previously mentioned by Bell and colleagues. Our observations revealed that a subset of tonically active mossy fibers exhibited variations in their firing rate during spontaneous movement (Figure S1) or in response to microstimulation-evoked motor activity. Notably, 23 out of the 48 fibers displayed this behavior (Figure 2C). Among these, 19 out of 23 fibers with rhythmic motor activity demonstrated rhythmic firing rate alterations that were synchronized with motor nerve activity, with a specific correlation value [INSERT VALUE HERE]. A direct association was discerned between the frequency of the mossy fiber firing rate modifications and the frequency of the smoothed motor nerve bursts (Figure 2D). Some fibers even exhibited this pattern during spontaneous bursts. When subjected to varying microstimulation intensities, certain mossy fibers demonstrated that the frequency of their firing rate modifications escalated in tandem with the microstimulation intensity [INSERT VALUE HERE] (Figure 2E). Conclusively, our data strongly suggests that mossy fibers transmit motor-related information closely aligned with the rhythmicity of swimming movements.
ChatGPT link: https://chat.openai.com/share/e88bd31e-ca63-4d7d-b356-4bbbc6c01443
Although the paragraph generated might not be publication-ready — the style can be uneven, at times painfully flat or overly ornate, and there are words that could be trimmed — the foundational structure is in place, and you are in a good position to begin revising. You might argue that you could achieve the same result in less time without ChatGPT, and perhaps that’s true. However, my experience shows that using AI in this manner can help writers sidestep two common problems with first drafts. Some writers — myself included — are paralyzed by the blank page. For this group, speaking thoughts aloud and then using a template with ChatGPT to produce a workable draft is a major psychological relief and a great way to overcome procrastination. Other writers tend to dive too quickly into drafting without adequate structural planning and then struggle to revise the resulting jumble of words. Preparing a template for ChatGPT forces these writers to think about structure first, making subsequent revisions easier.
Prime prompts: Supplying AI with details about the context, formatting, tone and template a writing task calls for leads to more useable drafts as output.
It’s true that a Results paragraph is, in some ways, as simple as scientific writing gets. But using this strategy on a simple format can help users develop good practices that can prove especially important when using AI tools to assist with more complex passages. Think of scientific writing as a cognitive act that requires ongoing decisions at multiple levels: substance (the core ideas and their connections), structure (how words, sentences and paragraphs are organized for clarity and impact) and style (word choice that balances personal taste with professional expectations). Although these three levels aren’t wholly separable, it’s crucial to think ahead of time at which level you want tools such as ChatGPT to intervene.
For a Results paragraph, the substance is set fairly strictly by your findings, and the structure is largely predetermined by scientific conventions, leaving mainly the style. Tools such as ChatGPT may not produce stylistically perfect text, but you can further refine it to your specifications. And because you came prepared with a clear template and your thoughts in order, you can help ensure that AI is limited to the grunt work of drafting, rather than unduly influencing the creative process. To be sure, you may want to tap AI for structural or conceptual assistance, but doing so may require different approaches, which we’ll explore in future columns. The key lies in recognizing and respecting these distinctions.
Data-privacy concerns arise when using standard web interfaces, as user inputs can be adopted to train future AI models, though certain technical workarounds offer more protection. And at least one major journal (Science) and the U.S. National Institutes of Health have banned the use of AI for some purposes. Lastly, although generative AI generally does not pose a high risk of detectable plagiarism, that risk may increase for highly specialized content that is poorly represented in the training data (which might not be much of a concern for the typical user but could be a larger concern for the typical scientist). Some AI systems in development may overcome some of these problems, but none will be perfect. We’ll discuss these and other issues at length as they arise.
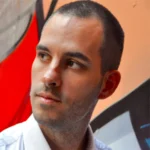
Recommended reading
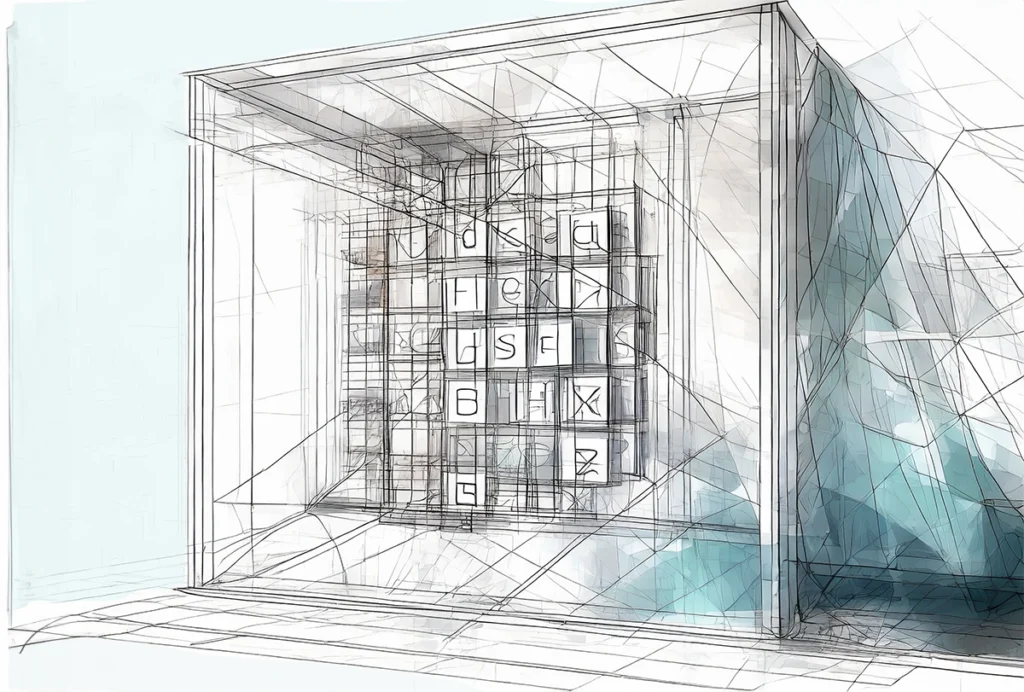
From bench to bot: How to use AI to structure your writing
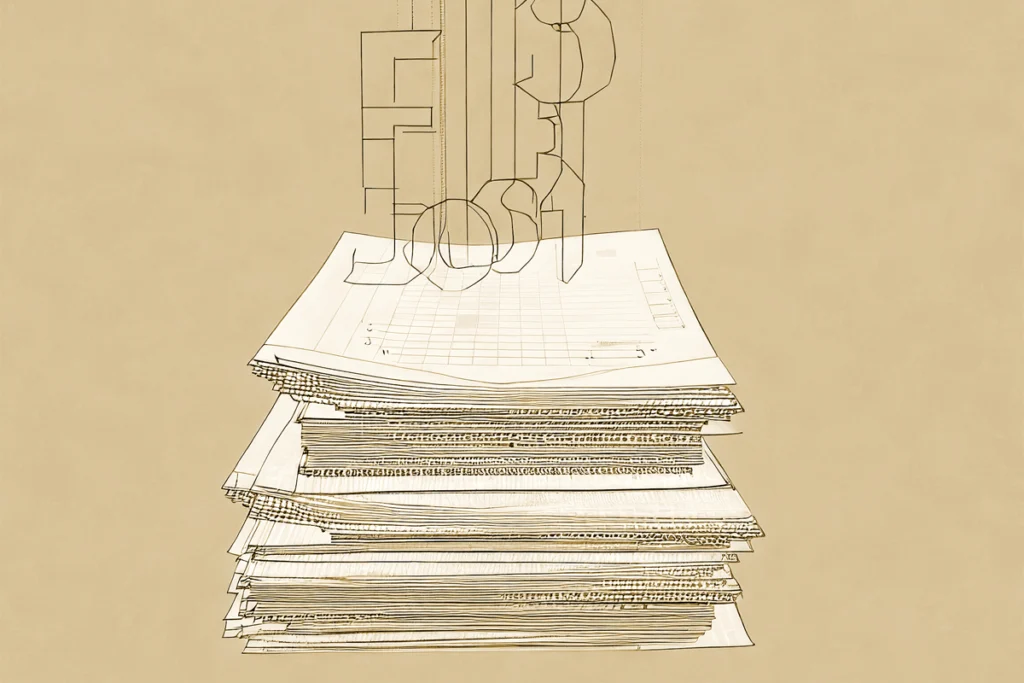
From bench to bot: A scientist’s guide to AI-powered writing
Explore more from The Transmitter
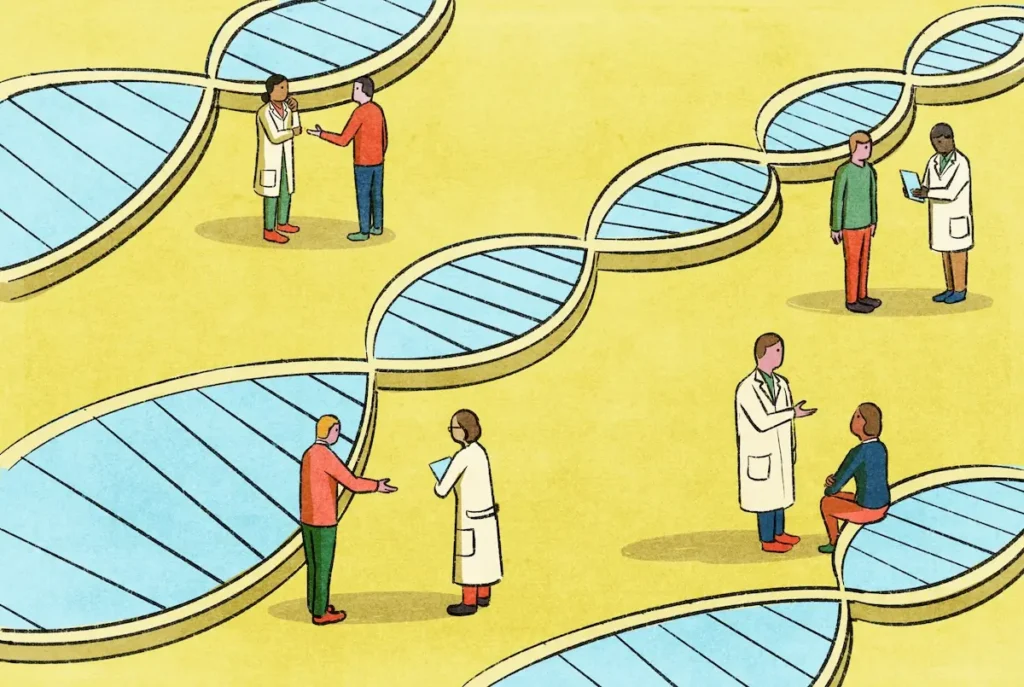
Building an autism research registry: Q&A with Tony Charman
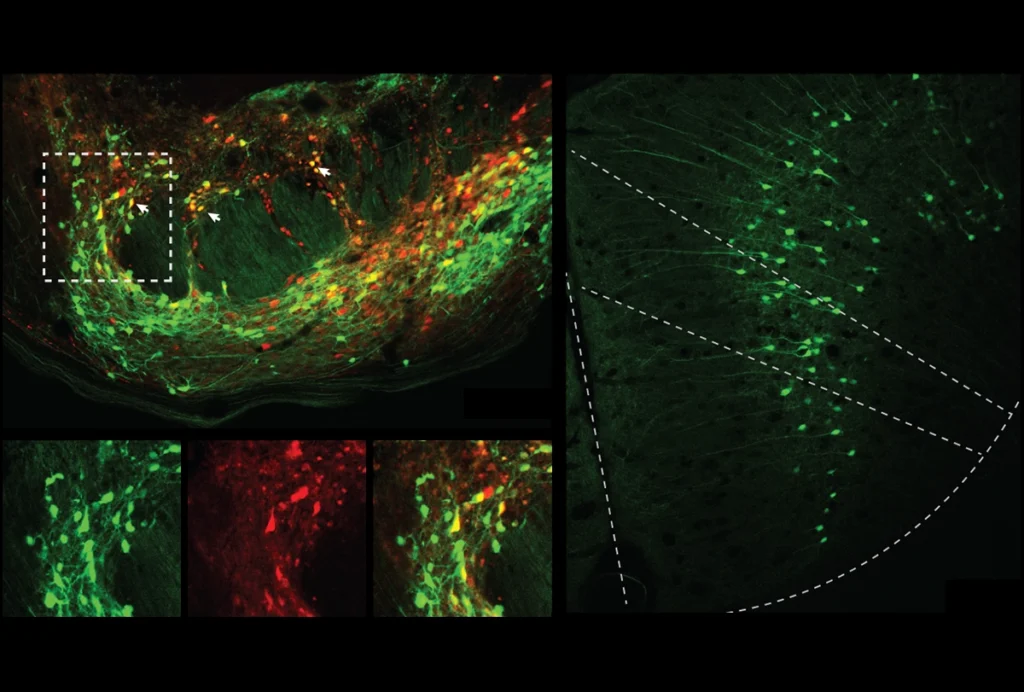