Boost your writing with AI personas
Asking ChatGPT to review your own grant proposals can help you spot weaknesses.
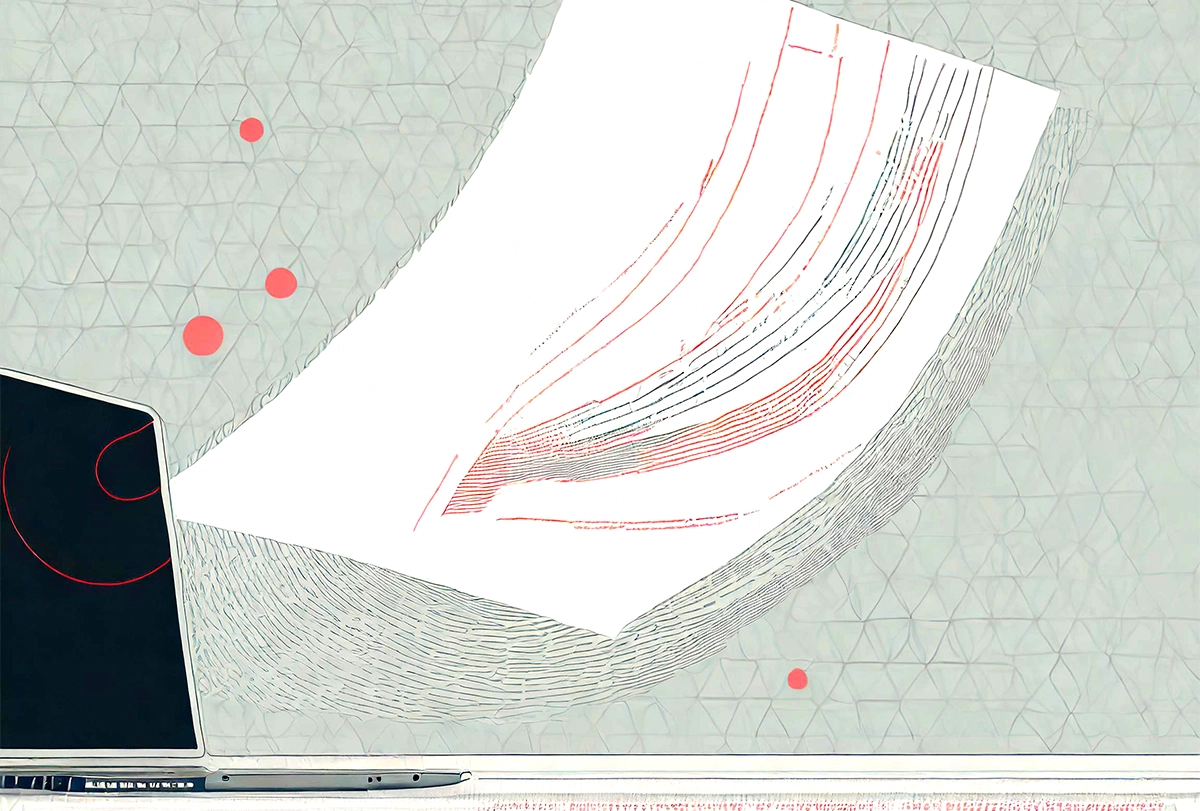
One of ChatGPT’s earliest parlor tricks was getting it to adopt a “persona.” ChatGPT became one writer’s personal stylist and another’s life coach, and yet another user asked it to act as Eric Cartman from “South Park” and pontificate on the benefits of a healthy diet. One startup, called character.ai, invited users to train chatbots to impersonate anyone from Isaac Newton to Super Mario. It was—at one point, at least—in funding talks at more than $5 billion valuation.
Are artificial-intelligence (AI) personas just high-dollar entertainment, or can they provide useful scientific feedback? I was intrigued by this possibility when business professor Ethan Mollick, of the Wharton School of the University of Pennsylvania, posted his thoughts about it on Twitter:
Not sure how to feel about this as an academic: I put one of my old papers into GPT-4 (broken into into 2 parts) and asked for a harsh but fair peer review from a economic sociologist.
It created a completely reasonable peer review that hit many of the points my reviewers raised pic.twitter.com/VTVwkB8ubL
— Ethan Mollick (@emollick) March 19, 2023
I tried a GPT-powered peer review with one of my own papers. The feedback it provided wasn’t comprehensive, but it did raise valid points—including some useful comments about the implications of the work that I wish I’d incorporated into the discussion section. In other words, I came to about the same conclusion as Professor Mollick: GPT gave a completely reasonable peer review.
You don’t have to trust me or Mollick. A study posted on arXiv in October took a more systematic approach to peer reviewing with large-language models. A team of computer scientists at Stanford University developed a pipeline that takes a paper and analyzes the writing to produce structured feedback, including significance and novelty, potential reasons for acceptance, potential reasons for rejection, and suggestions for improvement. The team gathered a human reviewer feedback dataset, comprising 8,745 comments for 3,096 accepted papers across 15 Nature journals, and 6,505 comments for 1,709 papers (both accepted and rejected) from a computer science research conference called the International Conference on Learning Representations (ICLR). They then used AI to generate feedback on these same papers and employed computational methods to compare overlap in points of critique.
The advice given by GPT-4 on scientific manuscripts, they found, overlapped with human-raised feedback by 30 percent for Nature journals and 39 percent for submissions to ICLR—about the same degree of overlap that they measured between any two human reviewers. What I found more intriguing is that this overlap increases to nearly 44 percent for papers that were ultimately rejected from ICLR, suggesting that GPT-4 might be even more useful at identifying the shortcomings of less robust research. (You can see the prompt they used in supplementary Figure 5 of the preprint.)
This isn’t to say that you should use AI to peer review the work of others—this is expressly forbidden by the National Institutes of Health (NIH) and may run afoul of journal policies. But AI could be a useful tool to help level up your own work before you submit it to a funding agency or journal.
Let’s take a look at an example. Here, I adapted the prompt from the preprint above. Instead of asking the AI to review a paper, I asked it to review a specific aims page. And instead of the five categories the authors use, I used a rubric I developed based on a diagram available online from a grant-writing book. To avoid any confidentiality issues and to ensure I could evaluate the quality of the feedback, I used a specific aims page from a funded NIH F31 grant of my own from several years ago.
Prompt:
You compose high-quality peer review feedback on specific aims pages for grant applications to be submitted to the NIH.
Start by “feedback outline.”
And then organize your feedback into sections, guided by the following eight questions:
- What is the specific gap in knowledge and/or barrier(s) to progress being addressed?
- What is the objective of the proposed research?
- What is the central hypothesis?
- How do the specific aims test the hypothesis?
- What are the expected outcomes of the specific aims?
- How would the expected outcomes of the specific aims achieve the objective?
- How does achieving the objective fill the gap in knowledge or overcome the barrier(s) to progress?
- How does achieving the objective advance the scientific discipline? What are the clinical implications?
Each section should have two bullet points. In the first, state how the author answers the question in the list. In the second bullet point, suggest ways to improve and strengthen the specific aims page for that aspect. For each suggestion to improve, use **>=2 sub bullet points** to further clarify and support your arguments in painstaking detail. Be as specific and detailed as possible. Be thoughtful and constructive. Write outlines only.
“““INSERT TEXT OF SPECIFIC AIMS PAGE”””
(Putting text in triple quotes ensures that ChatGPT differentiates the text to be acted on from the instructions.)
ChatGPT output:
Feedback Outline
- Gap in Knowledge/Barrier(s) to Progress
- Author’s Answer: The inability of current research to fully understand the neural mechanisms behind sensory filtering in the mammalian cerebellum, which is linked to disorders such as autism and schizophrenia.
- Suggestions for Improvement:
- Clarify the specific aspects of sensory filtering that remain unexplored: Detail how existing research has fallen short in elucidating these mechanisms.
- Strengthen the rationale:
- Provide more detailed evidence linking the gap to the mentioned disorders.
- Highlight previous attempts to address this gap and explain their limitations.
- Objective of the Proposed Research
- Author’s Answer: To dissect the cellular and circuit mechanisms of sensory filtering using weakly electric mormyrid fish as a model system.
- Suggestions for Improvement:
- Specify the broader implications: Elaborate on how findings from this model system could inform understanding of the mammalian cerebellum.
- Define clear, measurable goals:
- State explicitly how success will be evaluated.
- Describe expected milestones that would demonstrate progress toward the objective … (see ChatGPT link below for full text)
ChatGPT link: https://chat.openai.com/share/e00e1151-b16b-4d17-ab6a-8c986a868b83
This feedback outline provides two useful things. First, by asking the AI to reflect your writing back to you, you can get a better sense of what you’re actually conveying, rather than what you think you’re conveying. It’s difficult to overstate how valuable this reality check can be during the drafting stages.
Second, you get some suggestions for improvement. Admittedly, the suggestions are a mixed bag. Some aren’t applicable to a training (F) grant, and some of the shortcomings the AI brings up are addressed elsewhere in the grant. However, it does raise some valid points. One concern, for example, was that the proposal may overstate how much experiments in mormyrid fish would shed light on the mammalian cerebellum. This strikes me as a legitimate concern that I’d want to better address here or substantiate better in another part of the grant application. The AI also points out I haven’t provided sufficient preliminary data, which isn’t true if you read the rest of the grant. But I could have foregrounded more preliminary data in the aims page.
The interesting thing about creating an AI persona is that you can ask follow-up questions to refine specific feedback you’re interested in, or just cut right to the chase, as I did: When I asked my AI reviewer what the most likely reasons for rejection were, it suggested “insufficient justification of the model system,” which, to be fair, is a critique I heard constantly when working in an unusual model organism.
Personas don’t have to be limited to a generic “peer reviewer.” In different GPT chat threads, you can craft different reviewer personas. You might ask the AI to take the point of view of a decision-maker at a funding agency or to emulate scientists outside your discipline. You can also provide the grant’s selection criteria and the mission or mandate of the funding organization and ask GPT to evaluate how your grant falls short of the funder’s criteria. The more details you provide, the better behavior you’ll get from the AI.
This is not to say that AI personas are providing you ground truth. Rather, think of them as brainstorming partners or participants in a writing workshop. The personas offer a mix of feedback—some of it immediately helpful and some less so. Your expertise plays a crucial role in sifting through this feedback to find what’s useful. In this sense, an AI persona is less a simulated peer reviewer than a novel method to engage with a kind of interactive, specialized guidance system. The true value of AI personas lies not in teaching us how to write better grants per se but in highlighting the blind spots in our writing. It acts as a mirror, reflecting back the areas for improvement we inherently know but might have overlooked.
Data-privacy concerns arise when using standard web interfaces, as user inputs can be adopted to train future AI models, though certain technical workarounds offer more protection. And at least one major journal (Science) and the U.S. National Institutes of Health have banned the use of AI for some purposes. Lastly, although generative AI generally does not pose a high risk of detectable plagiarism, that risk may increase for highly specialized content that is poorly represented in the training data (which might not be much of a concern for the typical user but could be a larger concern for the typical scientist). Some AI systems in development may overcome some of these problems, but none will be perfect. We’ll discuss these and other issues at length as they arise.
Explore more from The Transmitter
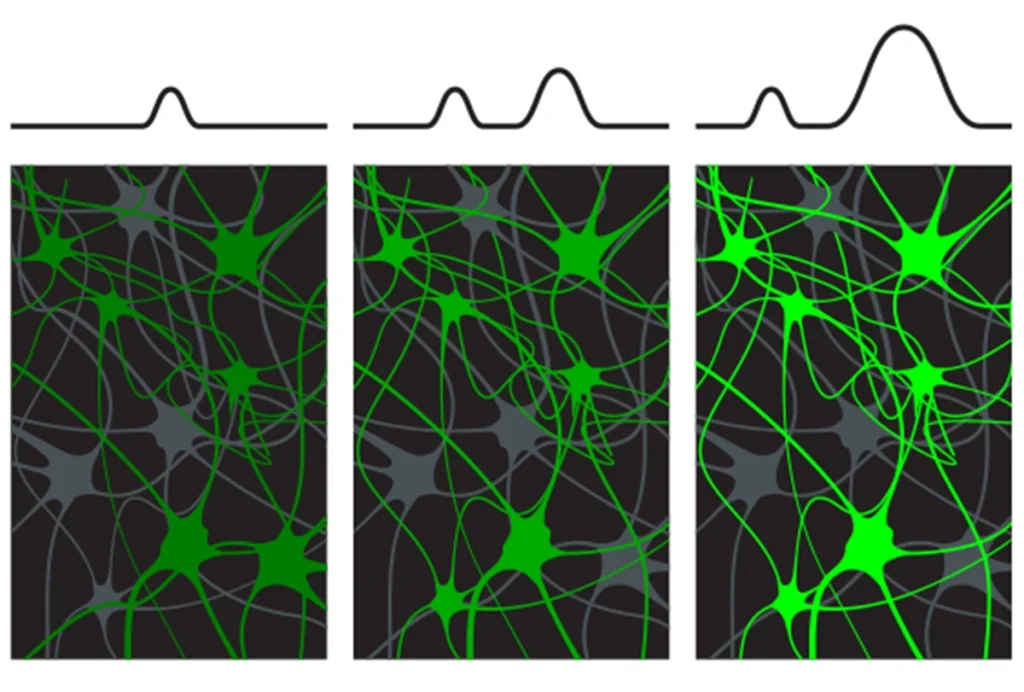
Cocaine, morphine commandeer neurons normally activated by food, water in mice
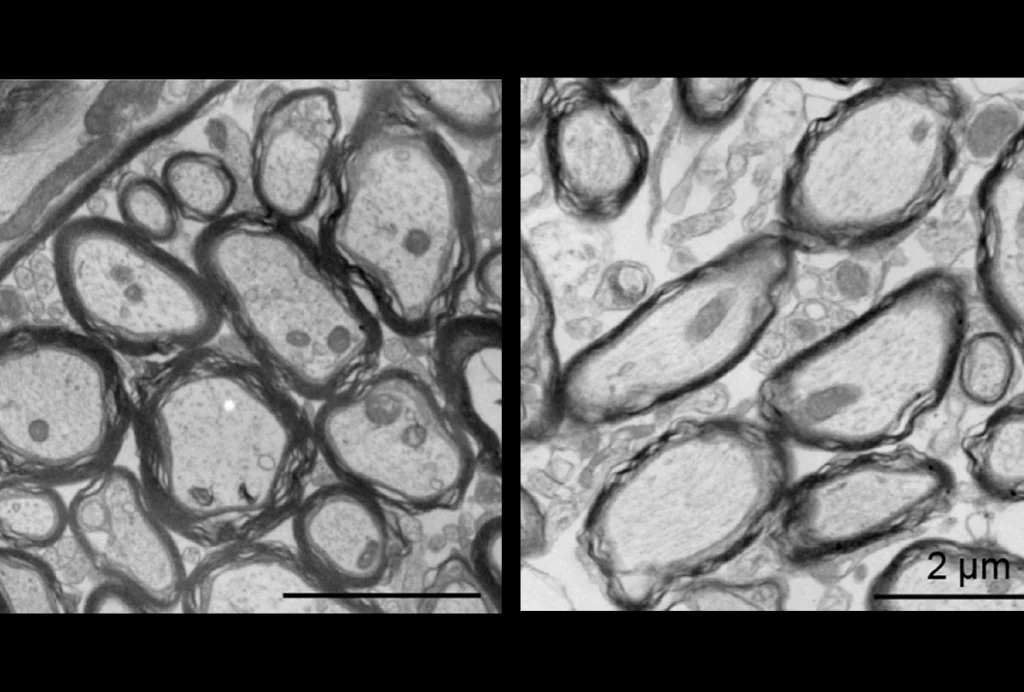
X chromosome inactivation; motor difficulties in 16p11.2 duplication and deletion; oligodendroglia
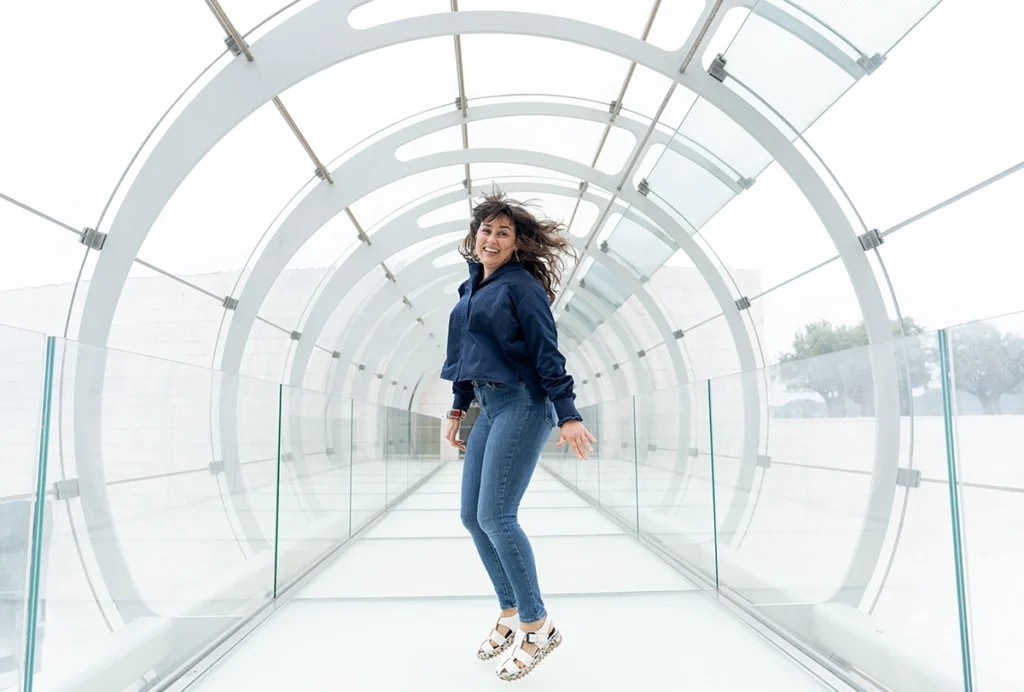