Morton Ann Gernsbacher is Vilas Research Professor and Sir Frederic Bartlett Professor of Psychology at the University of Wisconsin–Madison. She is a specialist in autism and psycholinguistics and has written and edited professional and lay books and over 100 peer-reviewed articles and book chapters on these subjects. She currently serves as co-editor of the journal Psychological Science in the Public Interest and associate editor for Cognitive Psychology, and she has previously held editorial positions for Memory & Cognition and Language and Cognitive Processes. She was also president of the Association for Psychological Science in 2007.
Morton Ann Gernsbacher
Professor
University of Wisconsin - Madison
From this contributor
Book review: “Neurotribes” recovers lost history of autism
Steve Silberman’s new book, “Neurotribes,” recounts his 15-year quest to understand “the legacy of autism.”
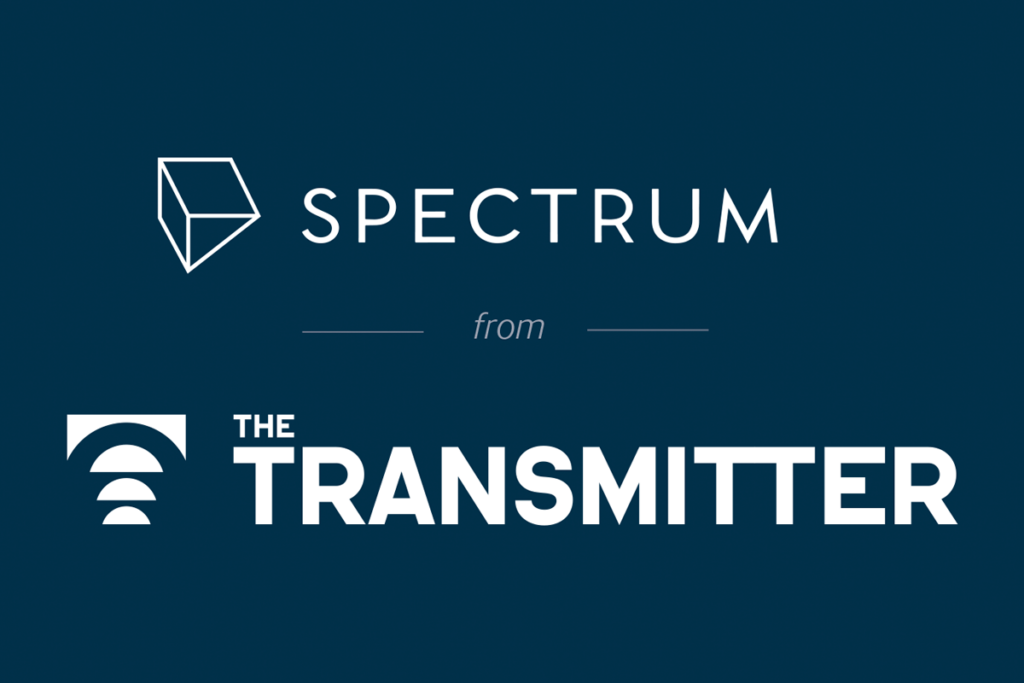
Book review: “Neurotribes” recovers lost history of autism
Explore more from The Transmitter
Federal funding cuts imperil next generation of autism researchers
As the International Society for Autism Research’s annual meeting begins, its next president reflects on a brewing crisis.
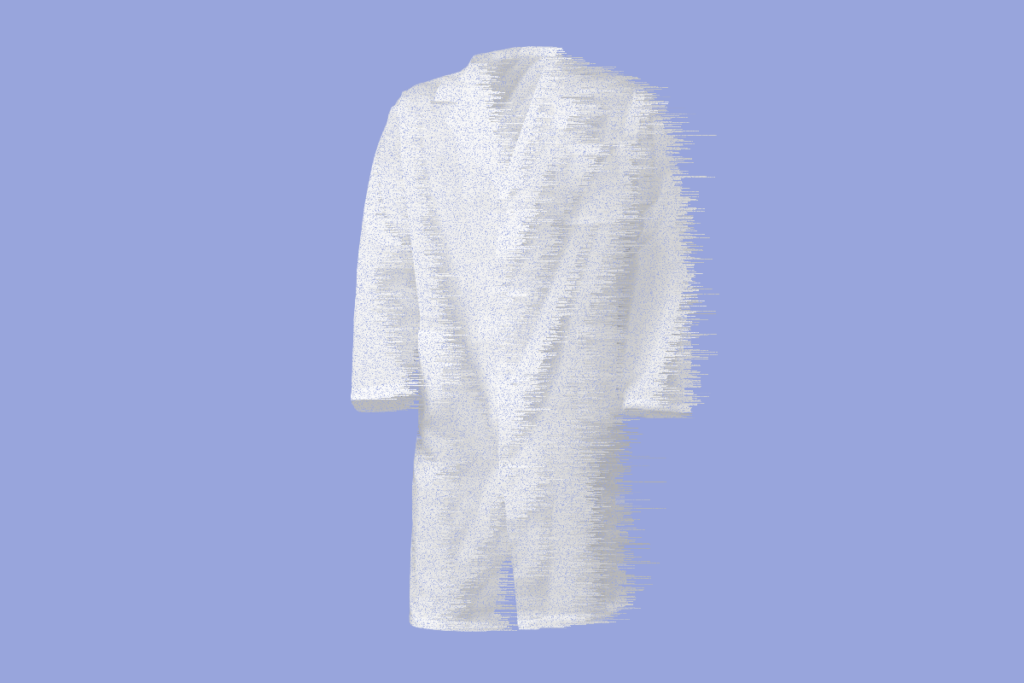
Federal funding cuts imperil next generation of autism researchers
As the International Society for Autism Research’s annual meeting begins, its next president reflects on a brewing crisis.
Null and Noteworthy: Reanalysis contradicts report of immune memory in astrocytes
The analysis, which has not yet been peer reviewed, attributes the finding to misidentified immune cells instead.
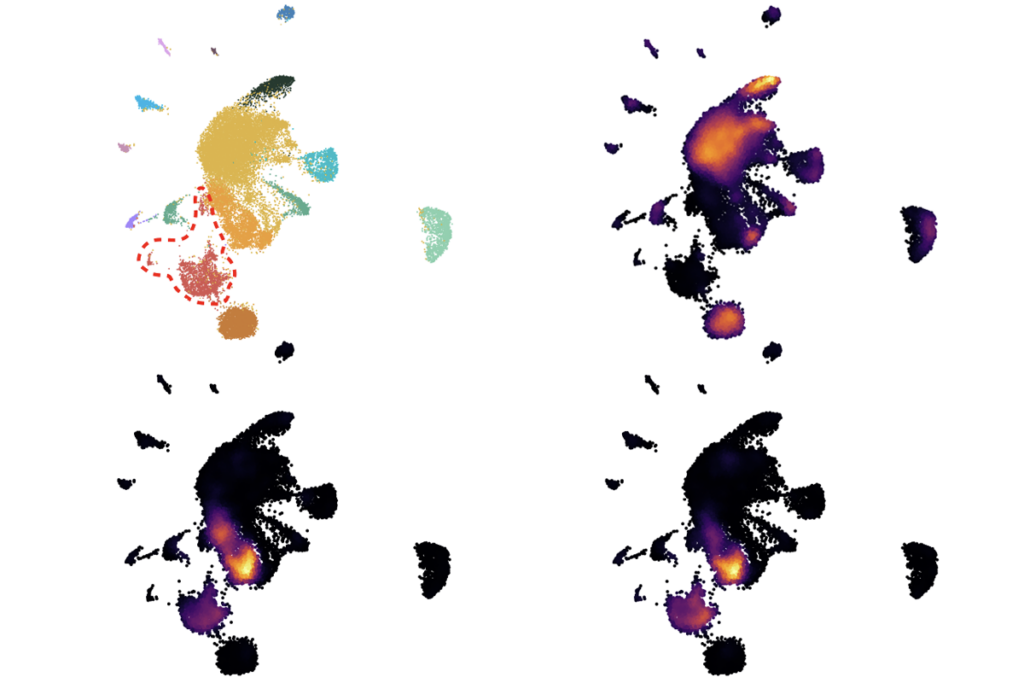
Null and Noteworthy: Reanalysis contradicts report of immune memory in astrocytes
The analysis, which has not yet been peer reviewed, attributes the finding to misidentified immune cells instead.
Documenting decades of autism prevalence; and more
Here is a roundup of autism-related news and research spotted around the web for the week of 28 April.
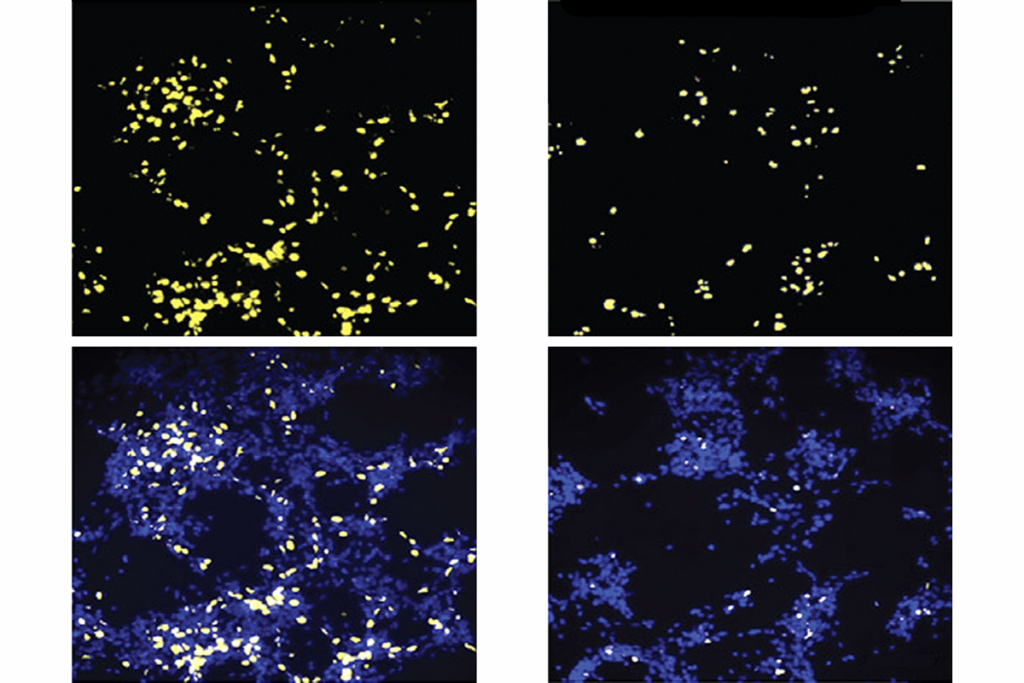
Documenting decades of autism prevalence; and more
Here is a roundup of autism-related news and research spotted around the web for the week of 28 April.