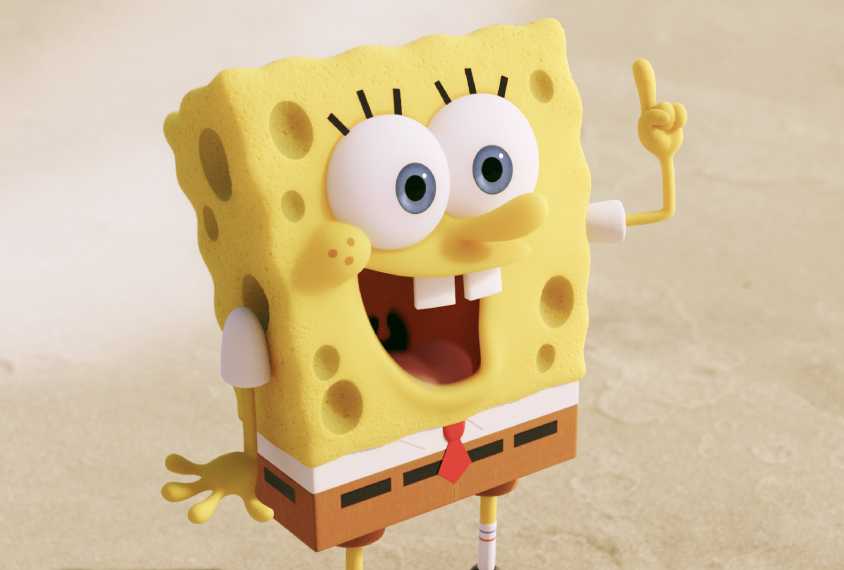
Grouping people with autism masks variations in visual learning
Some young children with autism excel on tests in which they must learn to connect visual cues.
Some young children with autism excel on tests in which they must learn to connect visual cues. And yet learning ability varies greatly among children on the spectrum, such that the success of some can be lost in the group average.
The unpublished findings were presented today at the 2017 Society for Neuroscience annual meeting in Washington, D.C.
“The punch line here is: If you were to average your data by group, you would miss significant heterogeneity in learning in autism, which is actually quite informative to understanding heterogeneity in symptoms,” says Rebecca Jones, assistant professor of neuroscience in psychiatry at Weill Cornell Medicine in New York, who presented the work.
The researchers studied ‘statistical learning,’ the ability to learn from exposure to environmental stimuli. For example, a baby learns language by hearing his parents speak. Several studies have hinted at problems in auditory learning in children with autism and in animal models of the condition.
But few studies have addressed how well children with autism learn from visual cues. A 2014 study showed that young children with the condition have different brain responses than their neurotypical peers on visual learning tests. One study in adults with autism found they are masters at learning visual patterns.
The new findings suggest that young children with the condition have varied behavioral learning patterns.
“There’s a large proportion of kids with autism whose learning patterns are similar to typically developing kids,” Jones says. “I think that’s great news.”
Spotting SpongeBob:
In the new work, 56 children with autism and 68 controls aged 2 ½ to 8 played a visual-learning game on an iPad. The children watched as various characters from the cartoon “SpongeBob SquarePants” appeared on the screen. The children didn’t know it at first, but two of the characters predicted SpongeBob’s appearance: One character often preceded a cameo from SpongeBob; the other rarely heralded his arrival.
The children were asked to tap on SpongeBob as soon as they spotted him. When the typical children figured out the other characters were predictors, their learning was reflected in how quickly they tapped. In particular, they would pause after the ‘low-chance’ character popped up, freezing before tapping the next character. Their reaction didn’t speed up when they saw the ‘high-chance’ character, though, which suggests they were already tapping as fast as they could.
The autism group, by contrast, did not show a pattern of responses indicative of learning. Their response time slowed over time in both the ‘high-chance’ and ‘low-chance’ scenarios.
But performance among individuals in the autism group varied dramatically. When the team looked at each child’s responses, rather than the group average, 34 of the children (61 percent) showed they had learned the associations as rapidly as the neurotypical group.
The degree of a child’s learning correlated with the severity of her autism features. Children with mild social problems performed more like typical children than did those with significant impairments. Having good verbal skills was associated with better performance in both typical children and those with autism.
“This is consistent with this idea that those who have higher statistical-learning skills have higher verbal skills,” Jones says.
The researchers plan to repeat the experiment while children lie in a brain scanner, to try to correlate patterns of brain activity with degree of statistical learning. They also aim to study how learning patterns change with certain behavioral interventions.
For more reports from the 2017 Society for Neuroscience annual meeting, please click here.
Recommended reading
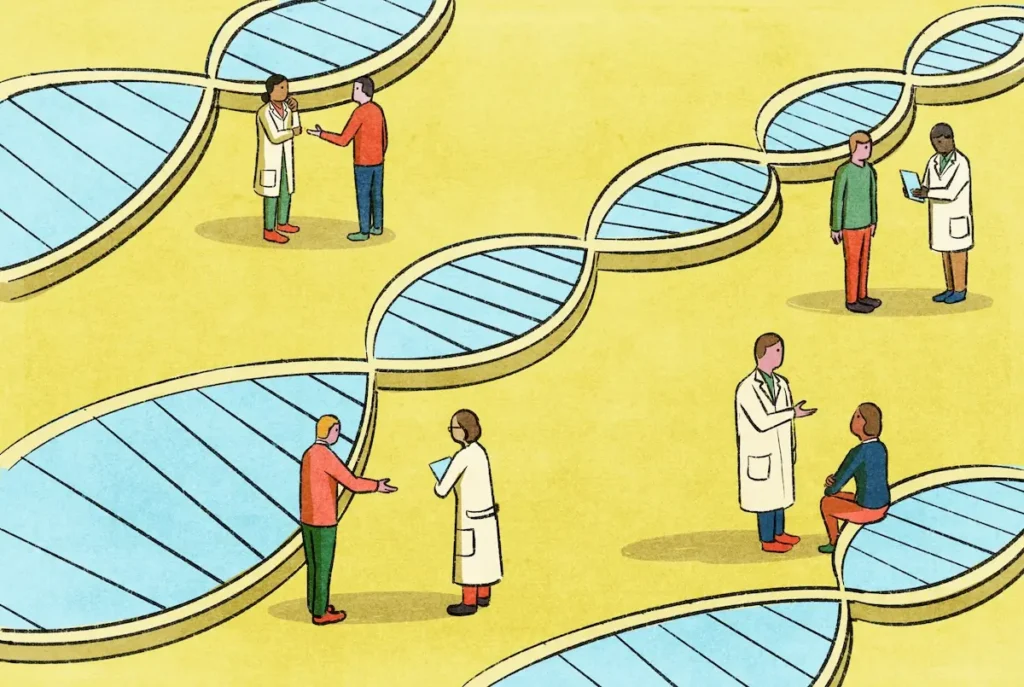
Building an autism research registry: Q&A with Tony Charman
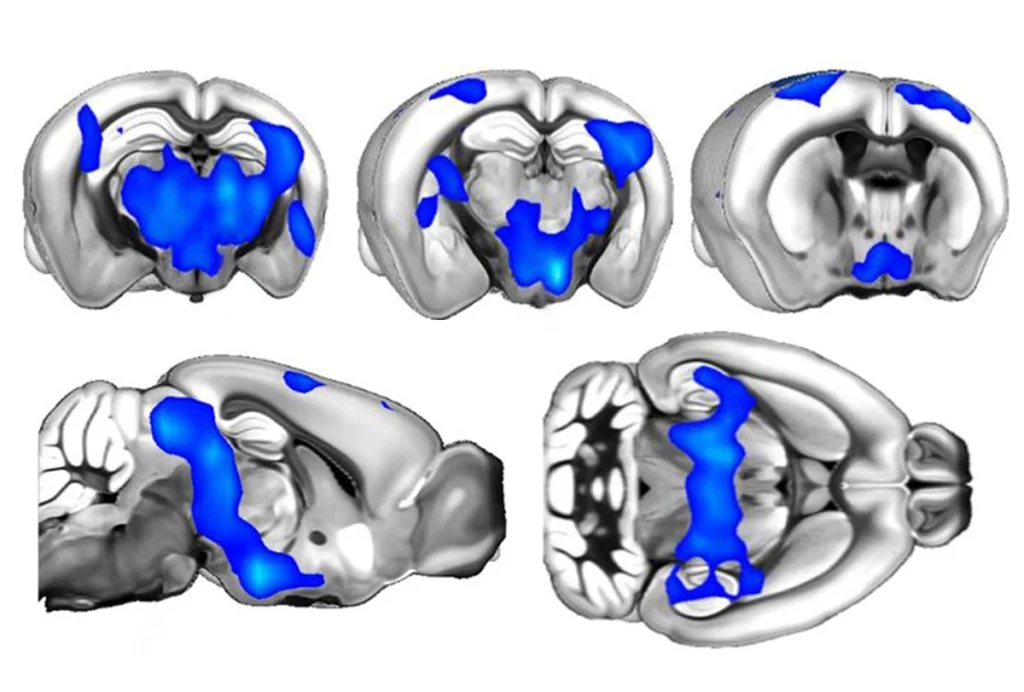
CNTNAP2 variants; trait trajectories; sensory reactivity
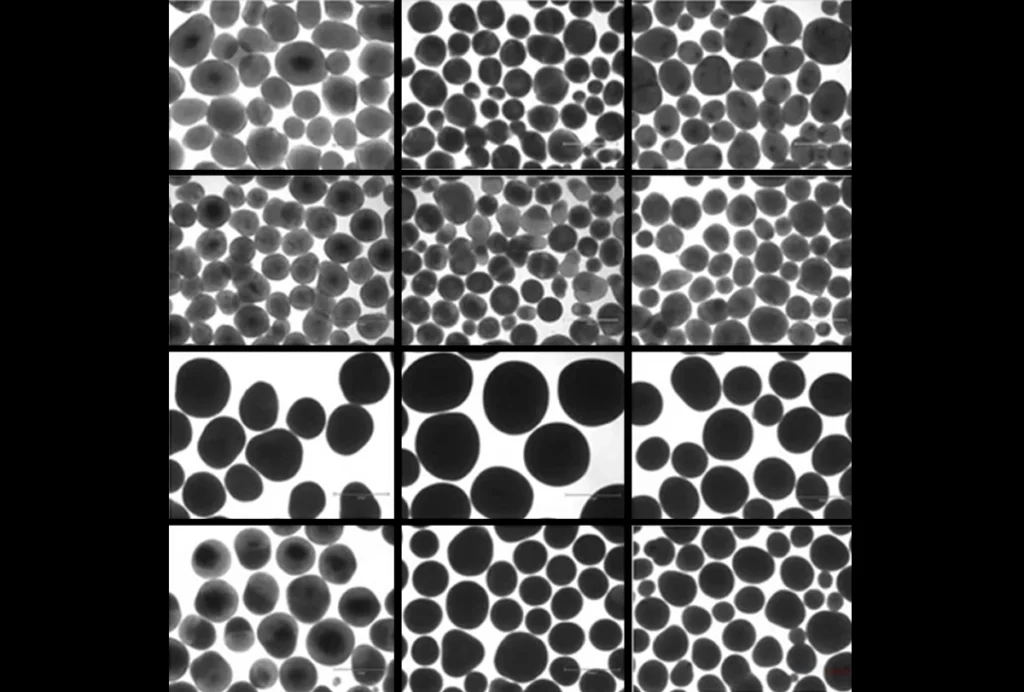
Brain organoid size matches intensity of social problems in autistic people
Explore more from The Transmitter
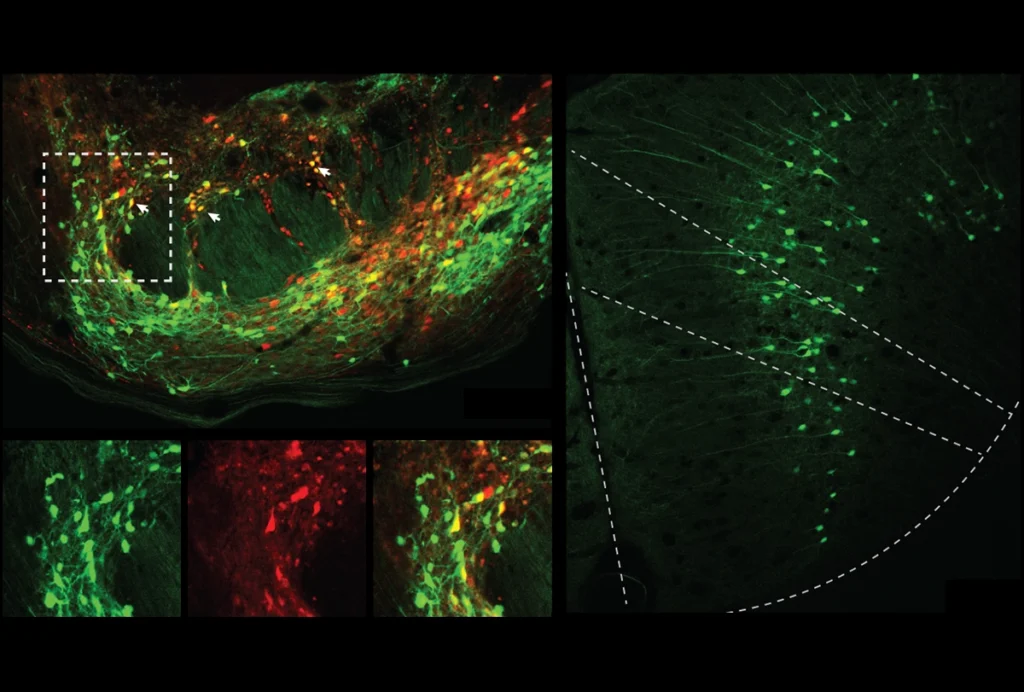
Cerebellar circuit may convert expected pain relief into real thing
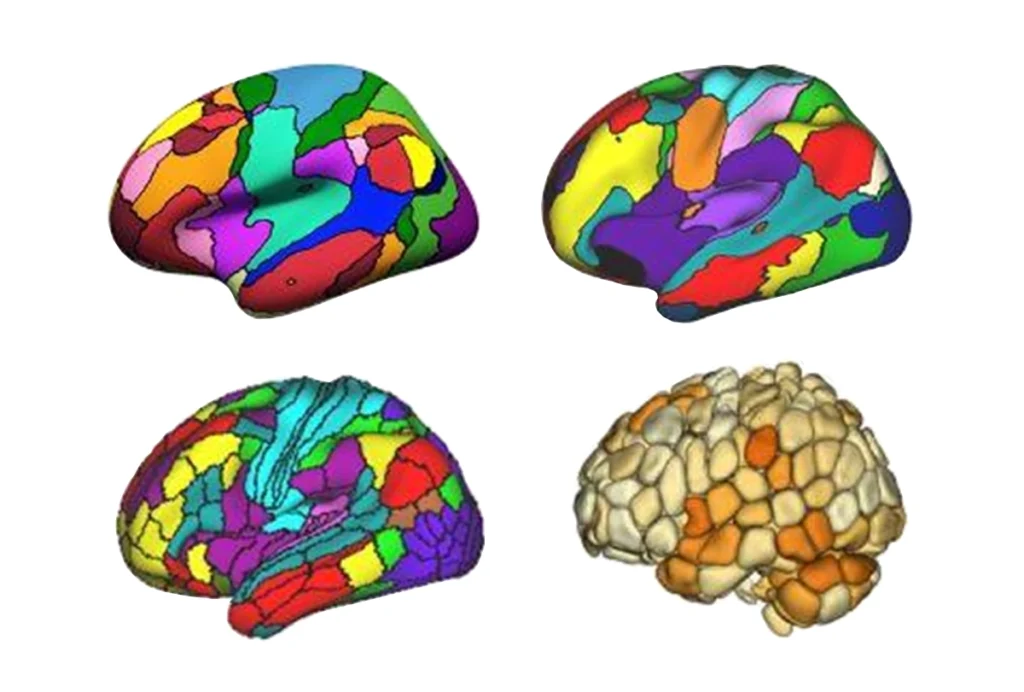