Self-scoring autism screen overlooks problems in girls
A widely used screening tool is equally effective at detecting autism symptoms in both black and white toddlers, but misses girls of either race.
A widely used screening tool is equally effective at detecting autism symptoms in both black and white toddlers, but misses girls of either race. Researchers presented the preliminary results today at the 2016 International Meeting for Autism Research in Baltimore.
The findings add to mounting evidence that screening tools overlook the subtle symptoms of autism in girls with the condition.
“Maybe we need to be looking at different things in girls,” says lead researcher Angela Scarpa, director of the Virginia Tech Center for Autism Research, who presented the findings.
Scarpa’s team used machine learning technology to create an automated, self-scoring version of the Modified Checklist for Autism in Toddlers (M-CHAT)-Revised. The test is a 20-item parent survey administered during an 18- or 24-month well-child visit; it takes about 10 minutes to fill out and 5 minutes for pediatricians to score. Based on the results, pediatricians can follow up with children showing signs of autism and determine whether to send them for a full diagnostic evaluation.
The automated version takes scoring out of the pediatricians’ hands, sidestepping the perils of human judgment and providing a clear-cut verdict on whether a child needs further testing, Scarpa says.
Her team looked at survey responses for nearly 15,000 toddlers. About 50 percent of the toddlers are white, 20 percent are black and 30 percent are of mixed or other backgrounds; the children’s mothers have a college education on average. The study included a roughly equal number of boys and girls.
The researchers fed the survey results into an algorithm that scanned them for meaningful patterns. The algorithm could accurately detect autism using only 12 of the 20 survey items, the researchers found. The responses from the remaining eight items — including a question that asked if a toddler engages in pretend play — did not provide meaningful data, Scarpa says.
Gender bias:
The algorithm could also indicate whether a boy was at low, medium or high risk of autism, based on the responses.
However, in girls, it missed the crucial middle ground: It picked up on girls at high risk of autism, and those at low risk, but was unable to identify girls with mild autism symptoms.
Most of the 12 key questions evaluate a child’s ability to share another person’s focus on an activity or object, known as joint attention.
Girls with severe autism tend to have trouble with joint attention, and the algorithm correctly sorted them into the high-risk group. But it missed girls with mild or moderate autism who can follow another person’s gaze and interpret social cues, lumping them into the low-risk group along with typically developing girls.
“We’re missing the nuances or shades of gray in girls with less severe autism symptoms,” Scarpa says. “The question is, ‘How we can improve on these tools to better detect autism in girls?’”
The findings may not apply in families where the parents cannot notice or describe a child’s atypical behaviors, Scarpa says. Still, her team plans to develop the short test into a mobile app that can be used to help remote or underserved communities screen toddlers for autism. It also plans to look deeper for race-based differences in autism risk.
Because the algorithm learns from every case, the researchers say, it will improve over time.
“The cool thing is that the machine learning never stops,” Scarpa says. “We’ll be able to look at [many] variables as we collect more and more data.”
To read more reports from the 2016 International Meeting for Autism Research, please click here.
Recommended reading
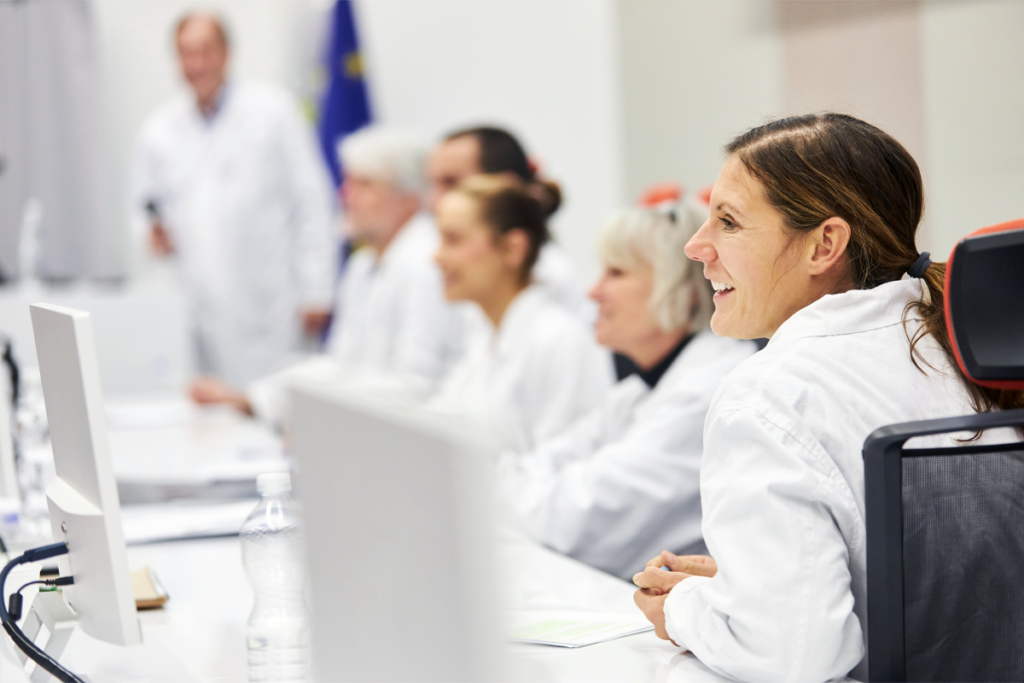
INSAR takes ‘intentional break’ from annual summer webinar series
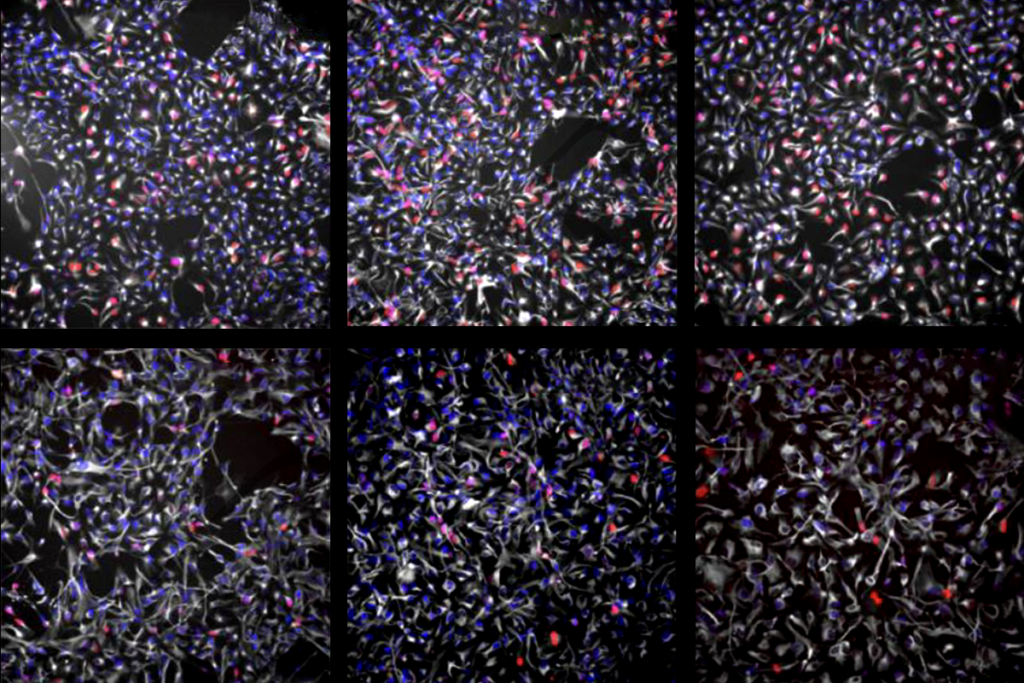
Dosage of X or Y chromosome relates to distinct outcomes; and more
Explore more from The Transmitter
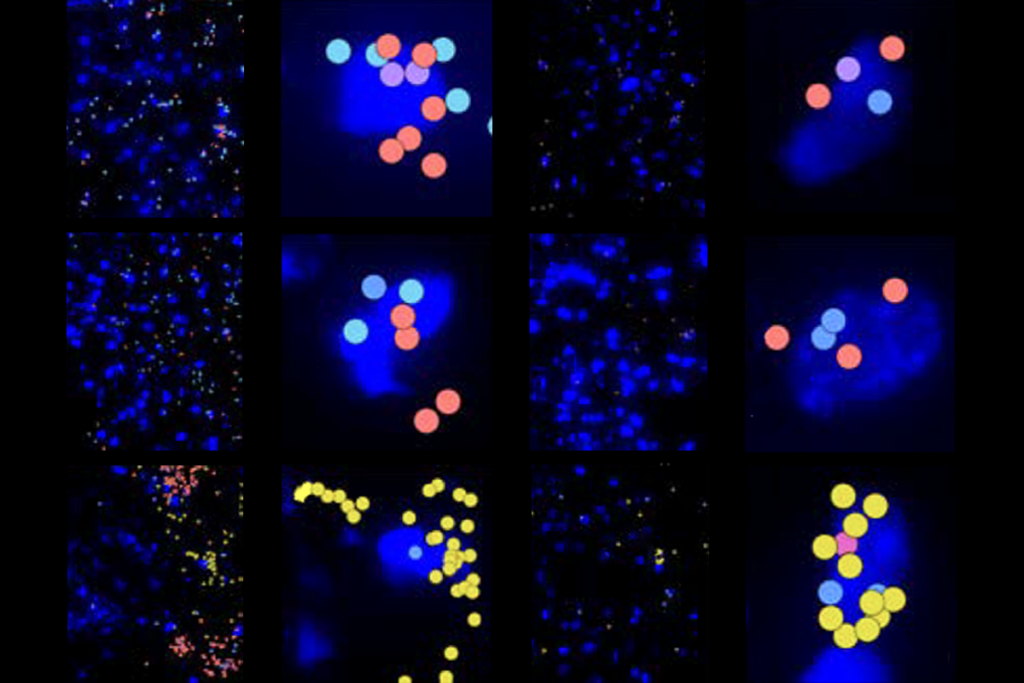
Machine learning spots neural progenitors in adult human brains
Xiao-Jing Wang outlines the future of theoretical neuroscience
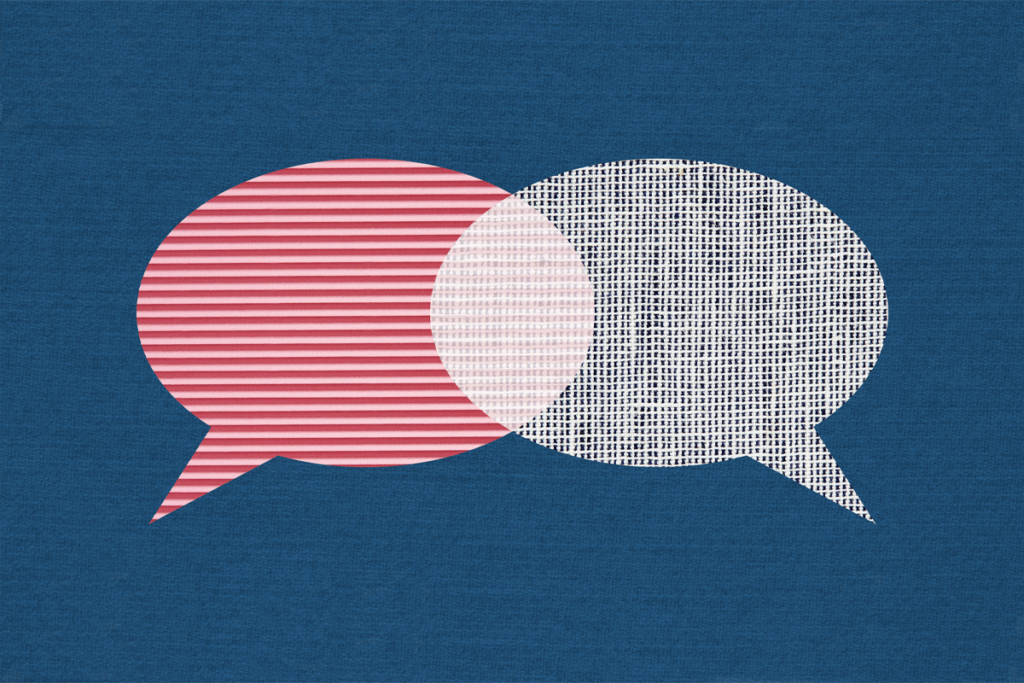