Knowledge graphs can help make sense of the flood of cell-type data
These tools, widely used in the technology industry, could provide a foundation for the study of brain circuits.
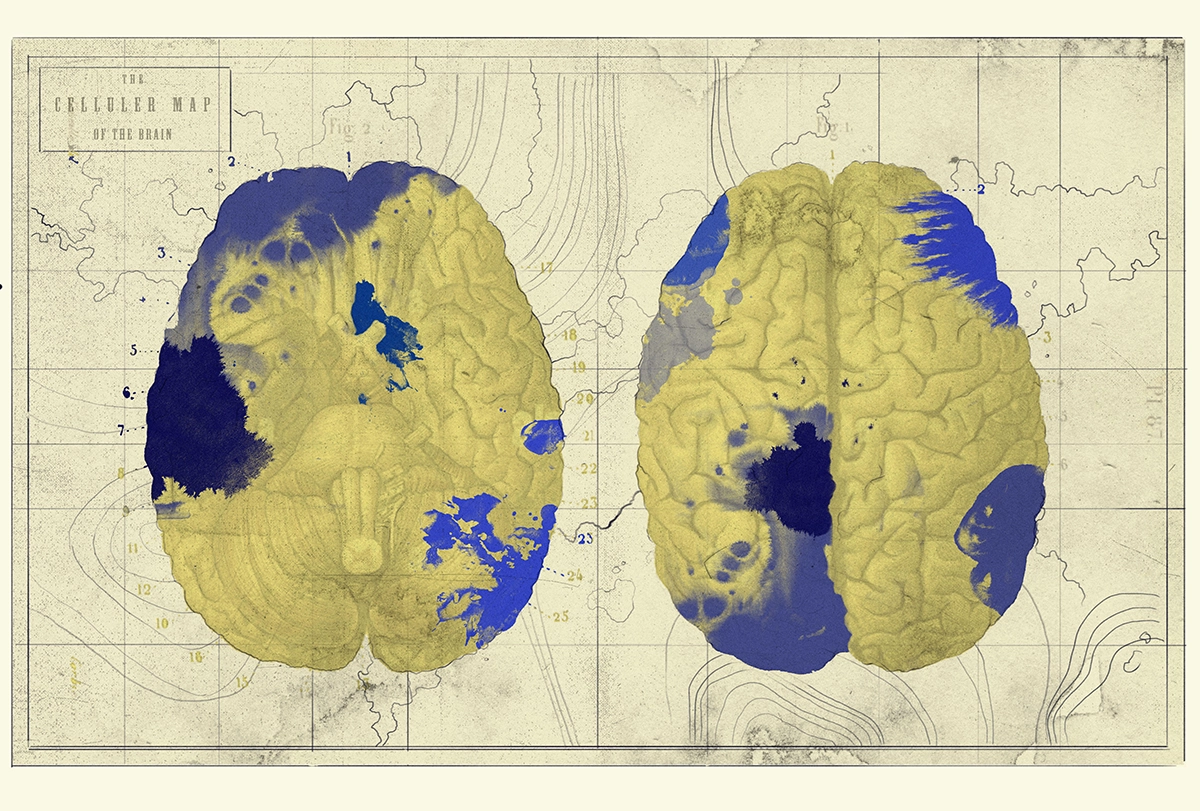
As a field, we’ve progressed in leaps and bounds in our ability to describe the brain’s cell types since pioneer neuroscientist Santiago Ramón y Cajal first used histological stains to visualize and classify neurons more than a century ago. Researchers now have an immense variety of data at hand—including anatomical, physiological, molecular and connectivity data—that can help classify brain cells. The newest, and perhaps most expansive, additions to the arsenal are single-cell genomic sequencing and high-throughput image analysis. Together, these tools have changed the landscape of neuroscience, enabling for the first time systematic high-throughput measurements of cells in the mammalian brain.
The promise of these new approaches was made clear last October with the publication of the largest human brain cell atlas to date. The effort, known as the BRAIN Initiative Cell Census Network (BICCN), used single-cell profiling methods to identify thousands of cell types, representing five years of work from hundreds of researchers, including myself.
As a participant and leader in these consortia, I have witnessed the tremendous power these approaches have to enhance our understanding of the brain. But they come with major informatics and computational challenges that we must solve. To create a truly comprehensive and quantitative description of cellular diversity, we need to develop new methods for organizing and navigating the information that cell census efforts produce and for mapping relationships between cell types and their properties.
I propose developing a platform for aggregating transcriptomic and other cell-type data that researchers can easily access, update and query. This type of collective resource, similar to that developed in the early days of genomics, will be an essential steppingstone to understand the function of the brain’s circuits.
Neurons and glia, the brain’s fundamental cell types, exhibit immense variety in their gene expression, shape and connections, and in the case of neurons, their electrical firing patterns. This makes defining and categorizing the brain’s cells extremely challenging. Indeed, we have no universally accepted notion of the various types of neurons and glia. High-throughput single-cell genomics, using a technique called RNA-seq, tracks the activity of thousands of genes in single cells or nuclei, offering a new characteristic by which to define cell types. Computational algorithms designed to detect clusters of gene expression group classes of cells with similar patterns. This combined approach is highly quantitative and identifies cell groups that often align well with cells’ morphological or physiological properties.
The BICCN consortium used these methods to create an essentially complete census of all the cell types in the mouse brain as defined by gene expression. A new effort, the BRAIN Initiative Cell Atlas Network (BICAN), is underway to extend this survey to human and nonhuman primates and create a comprehensive inventory of the cell types of the mammalian brain.
A major task ahead is to make sense of the massive amounts of data produced by these and other projects. One option is to map cell types hierarchically like a phylogenetic tree, a branching diagram that shows the evolutionary relationships among species based on similarities and differences in their physical or genetic characteristics. Most brain atlas projects attempt to map relationships between cell types using this approach, but it has limitations. Researchers can’t always precisely pinpoint cells’ developmental origin. And it’s unclear how well this representation works in evolutionarily older structures of the brain, which have many diverse and specialized cell types. Indeed, in these regions, we often find that transcriptomic relationships may not be easily represented as a simple tree-like structure.
I propose instead that complex relationships among transcriptionally defined cells may be more effectively represented by data structures known as knowledge graphs.
Knowledge graphs are a widely used in the technology industry and in computer science to aggregate and organize data and could provide a foundation for the study of brain cell types and circuits. Concretely, a knowledge graph is a relational data structure in which nodes represent entities, such as cell types and their attributes. The links, or edges, between nodes represent their relational and statistical associations.
K
nowledge graphs are well suited to cell-type data for several reasons. First, researchers are still actively studying which cellular properties are essential for defining cell types. Flexible data structures that can manage different versions of attributes and taxonomies can better capture our evolving picture. Second, knowledge graphs can capture uncertainty in relationships; for example, the strength of the connection between two nodes can indicate whether two cell types are truly distinct or represent a continuum. Finally, knowledge graphs can help determine whether new data reflect a novel finding, which is especially important given the capacity to profile millions of cells quickly.Cell-type knowledge graphs could also advance neuroscience by providing a knowledge structure, much as modern molecular biology and bioinformatics techniques have made it possible to organize genomics information into accessible databases with powerful search and annotation tools. These databases have been invaluable in helping to identify genes’ functions and the role they play in basic biology and disease.
To illustrate the potential of this approach, we recently developed an open online resource, the Cell Type Knowledge Explorer (CTKE), an interactive knowledge graph application that aggregates multimodal data from the BRAIN Initiative’s collaborative study of the cell types of the primary motor cortex in the mouse, human and marmoset. The CTKE aggregates cell-type data from many laboratories to produce data visualizations and text summaries. Underlying the CTKE is a data-driven knowledge base platform and ontology that links atlas data to a well-established body of neuroscience knowledge.
To build the CTKE, we began with standardized transcriptomics data and then incorporated other data modalities, including anatomical, electrophysiological, developmental and other cell properties. Users can search CTKE data by cell-type names, minimal sets of marker genes and historical terms from the literature, such as “pyramidal” or “chandelier.”
Much as comparing genomes among related species reveals how conserved these sequences are, mapping cell-type properties among the three species can reveal similarities and differences. This comparison can potentially help us to understand which characteristics are uniquely human. Users can explore rich phenotypic information about cell types from mice, humans and marmosets, including visualizations incorporating different types of data—molecular signatures from single-cell transcriptomics, morphological reconstructions, electrophysiological characteristics and the cell type’s locations in the brain. Though only a start, this resource is representative of modern knowledge-based approaches to data organization and can be readily extended to any region of the brain.
Teams at the Allen Institute and the Massachusetts Institute of Technology are building a novel brain knowledge platform (BKP), supported by the National Institutes of Health BICAN funding and Amazon Web Services technology, which will have the capacity to cover all brain cell types and their properties and relationships. The ultimate goal of the BKP is to become a dynamic resource that is continually updated with new information about brain cells and their types. Users will be able to query existing knowledge and to compare new experimental results, making it possible to get answers to research questions faster.
The project faces a number of challenges, including how to ensure the availability and consistency of cell-type information and how to meet the technology requirements to maintain the platform as an active resource. But with community interest and support, a cell-type community knowledge framework could become a benchmark resource for cell types in the cortex, promoting collaborative participation in the field.
Recommended reading
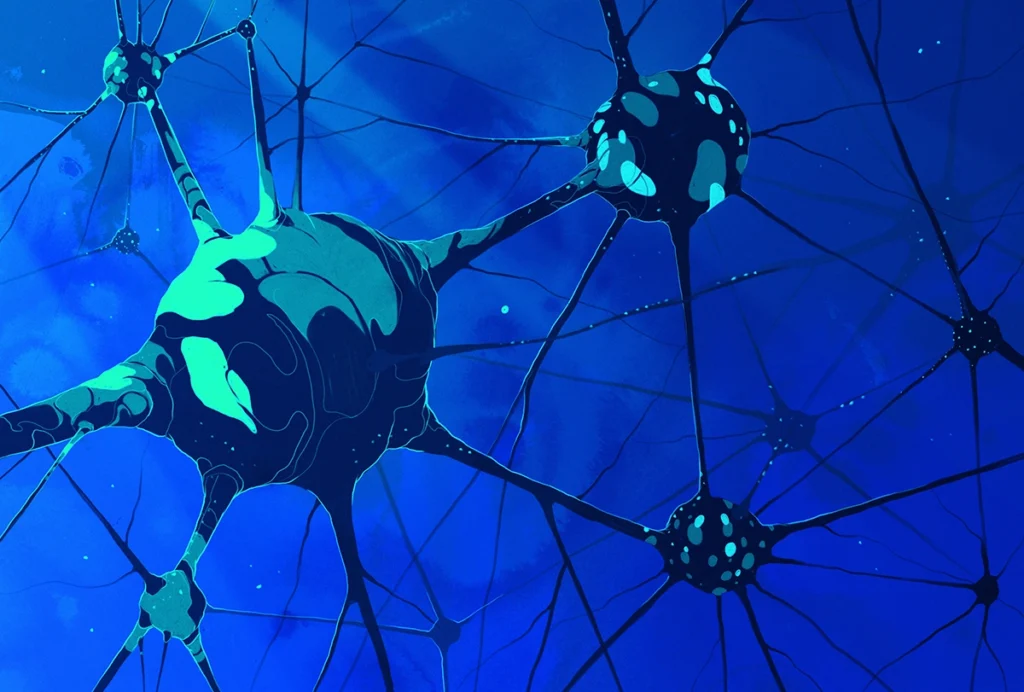
Where do cell states end and cell types begin?
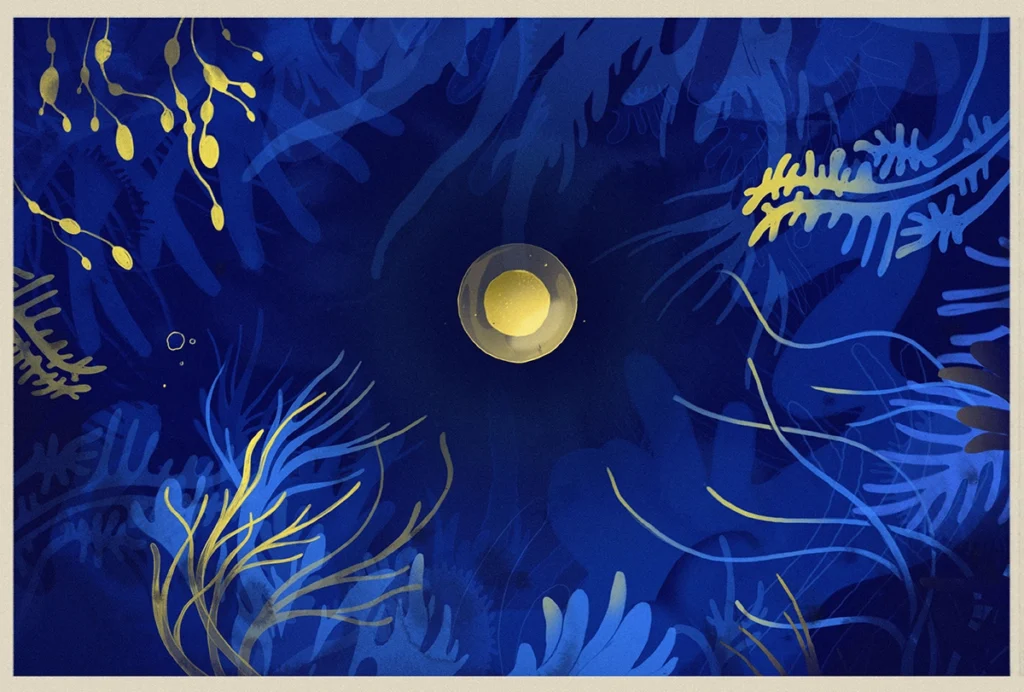
Building a brain: How does it generate its exquisite diversity of cells?
Explore more from The Transmitter
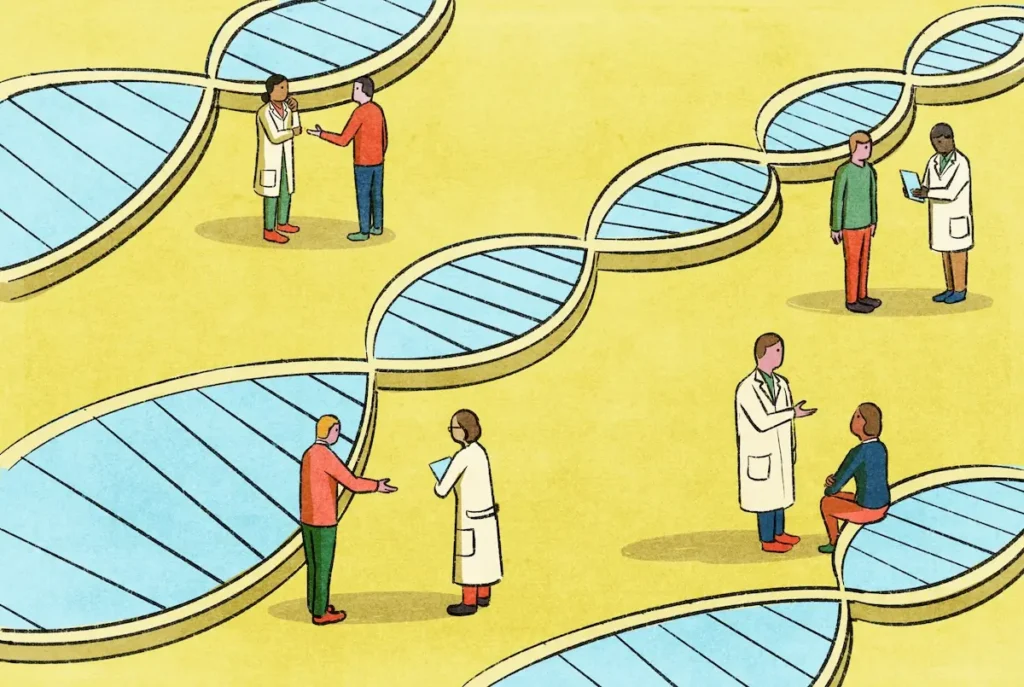
Building an autism research registry: Q&A with Tony Charman
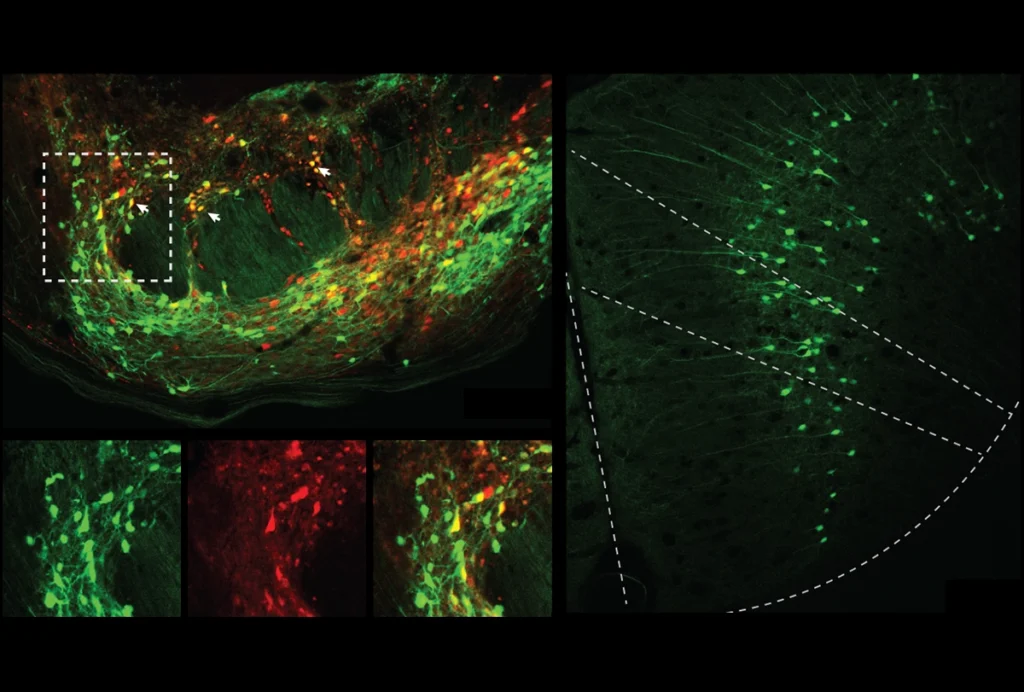