How to use race and ethnicity data responsibly in neuroscience research
Follow these four tips to avoid using the information in problematic ways, including as a proxy for environmental variables.
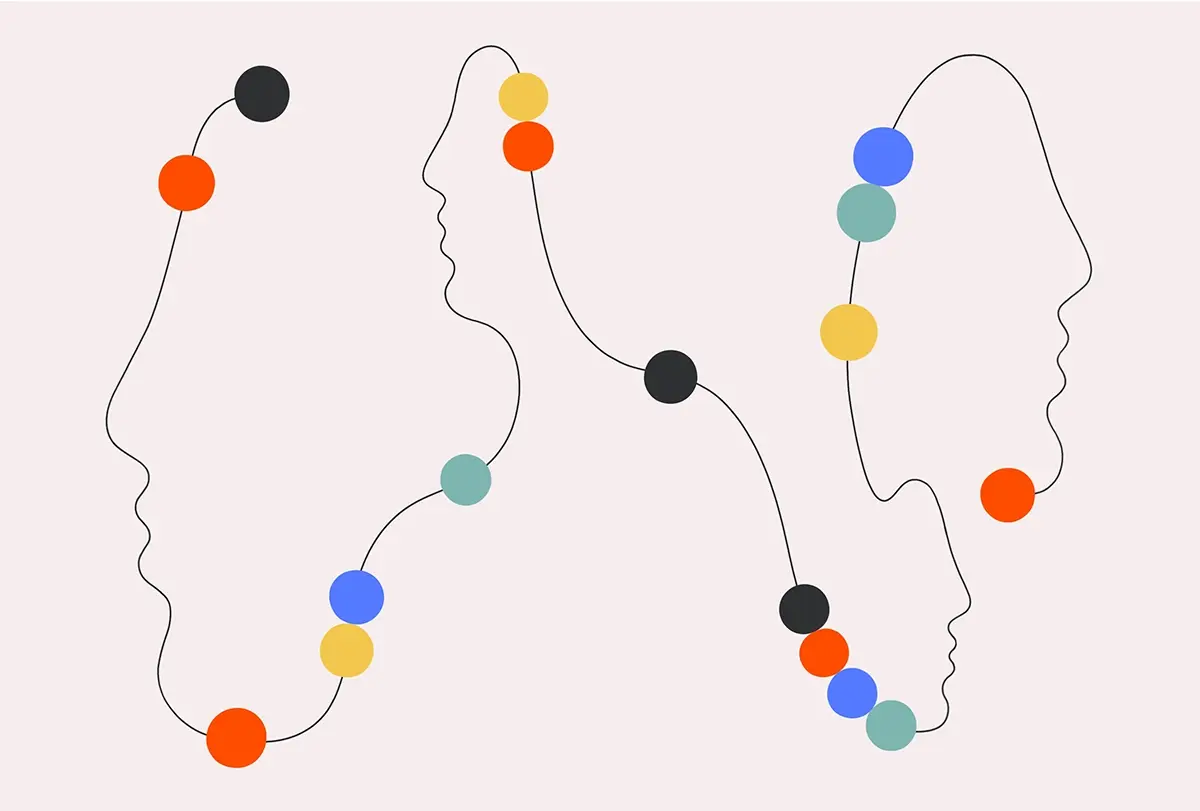
When Carlos Cardenas-Iniguez joined the Adolescent Brain Cognitive Development (ABCD) Study in 2021, his new colleagues were all talking about race, he recalls. Black Lives Matter protests after the murder of George Floyd the previous year had prompted serious dialogue in many sectors, and science was no exception.
One of the ABCD Study’s working groups at the time tried to write a consensus statement on how race and ethnicity would be used in the study but couldn’t come to an agreement, says Cardenas-Iniguez, a postdoctoral scholar at the University of Southern California. The scientists all held different views on how to account for race and ethnicity in the study.
Such differences can hinder research in other ways. Inconsistencies in how scientists report race and ethnicity in their work make it challenging to compare those data across studies, replicate them and even trust that the results are generalizable, Cardenas-Iniguez says.
The ABCD experience led Cardenas-Iniguez and a colleague to come up with recommendations for the “responsible use and communication of race and ethnicity in neuroimaging research,” which were published in Nature Neuroscience last month. The Transmitter asked Cardenas-Iniguez and other experts what researchers can do to advance the race and ethnicity conversation in neuroscience. Here are four of their top tips.
1. Provide as much information as possible about the race and ethnicity categories used in your study.
Race and ethnicity are social constructs that have no scientific or biological meaning, according to research recommendations published in the past three years by several scientific organizations, including the American Medical Association (AMA) and the American Psychological Association (APA), among others.
But scientists should still capture information about their study participants’ self-identified race and ethnicity, says Aravinda Chakravarti, director of the Center for Human Genetics and Genomics at New York University. “How would we know that the government spends its budget on white Americans or Black Americans?” Chakravarti says.
Because the terms and categories for race and ethnicity are controversial and constantly evolving, Chakravarti says, the best thing to do is to provide detailed information about how your study captured and used that information.
“Even if we don’t have the answer on how to do this, we would need people telling us what they are doing so we could discuss it out in the open,” says Teresa Girolamo, assistant professor of speech, language and hearing sciences at San Diego State University. This would allow researchers to spot “poor definitions” that are not “scientifically well founded,” she says.
At a minimum, researchers should report all of the options they provided to participants, which categories they merged (if any) and what categories they classified under “other.” Ideally, scientists should also include a brief note mentioning the limitations of this list and the categories it left out. In his paper, Cardenas-Iniguez provides an example for U.S.-based researchers.
2. Think twice before controlling for race and ethnicity or including them in a statistical model.
Although race and ethnicity have no biological significance, some scientists continue to use them as control variables or proxies for things such as socioeconomic or environmental variables in statistical models. Some of these practices can perpetuate the idea that race and ethnicity are “innate characteristics rather than outcomes of structural racism and racialization,” Cardenas-Iniguez and his colleague wrote in their paper.
Instead, researchers should consider what are they trying to control for.
“If you have other variables that can specifically measure what you are doing instead of using race, just use that one,” Cardenas-Iniguez says. The ABCD Study, for instance, uses geocoded residential information to account for differences in environmental variables.
If a researcher feels there is a legitimate reason to include race and ethnicity in a statistical model, they should explain their rationale. “We are accustomed to rationalizing the inclusion of variables, but with race and ethnicity the practice has been not to mention it,” says Kate Webb, an assistant neuroscientist at McLean Hospital who has written about structural inequalities in neuroscience.
3. Foster diversity in your samples and teams.
White people represent 95 percent of participants in the UK Biobank and about 76 percent of participants in the Human Connectome Project, two large neuroimaging databases. The homogeneity in these samples may limit the generalizability of the research findings.
“The lack of diversity is not a political problem; it’s a scientific problem,” says Agustín Ibañez, director of the Latin American Brain Health Institute at Universidad Adolfo Ibáñez. To help solve that problem, the institute created a neuroimaging dataset with brain scans from more than 700 people across five Latin American countries, who have historically been underrepresented in research.
Ibañez recommends that other researchers try to be mindful of the diversity of their samples.
Researchers must also provide information about their recruitment process. For example, Girolamo says she has heard investigators saying they are going to recruit from the Black community. “And I am like, ‘What is the Black community?’” she says.
Also, be aware of how recruitment protocols could exclude minoritized groups. For instance, if research labs are working only during business hours, “we are only bringing in people into our studies that have the time and the flexibility to be able to come in during those 9-to-5 hours,” says Elvisha Dhamala, assistant professor of psychiatry at the Feinstein Institutes for Medical Research.
It’s also important to foster diversity in research teams and citations. “If you are talking about particular populations, are there people on your team of those identities, and most importantly, are you citing researchers of those identities?” Cardenas-Iniguez says. “There is so much recognition that needs to be done.”
At the end of his article, Cardenas-Iniguez offers a list of questions researchers can ask themselves to verify that their work is not contributing to problematic ideas about race and ethnicity.
4. Hold yourself and others accountable.
Researchers should keep these recommendations in mind not only when conducting their own work, but also when reviewing other scientists’ papers and grant proposals, Webb says. “During peer review, scientists and journal editors actually should be requesting that any inclusion of race and ethnicity in a statistical model is justified,” she says.
Journal editors can also enforce the use of APA or AMA guidelines for talking about race and ethnicity as social constructs, Girolamo adds.
Researchers should also reflect on how they used race and ethnicity in their previous papers and how representative those categories were, and acknowledge any issues when citing that work in the future, Girolamo says. Back in 2020, so many people were cognizant of movements such as Black Lives Matter and Stop Asian Hate, she says. “I am wondering what happened. Now we have people talking about these very relevant issues in science, but we are still doing the same things.”
Recommended reading
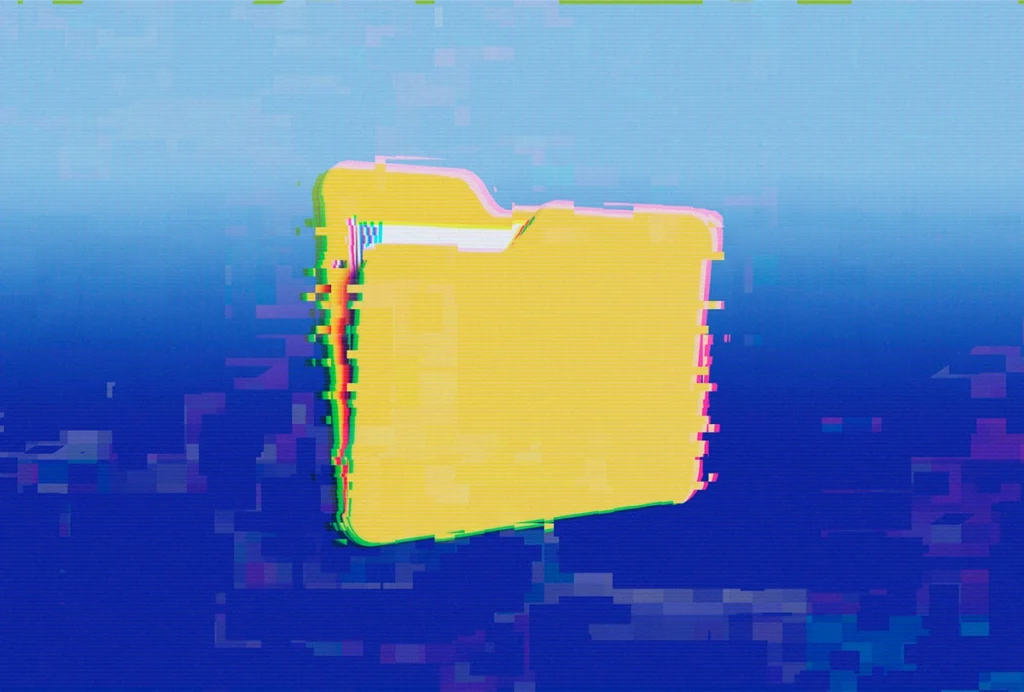
Data access changes to UK Biobank stir unease in neuroscientists
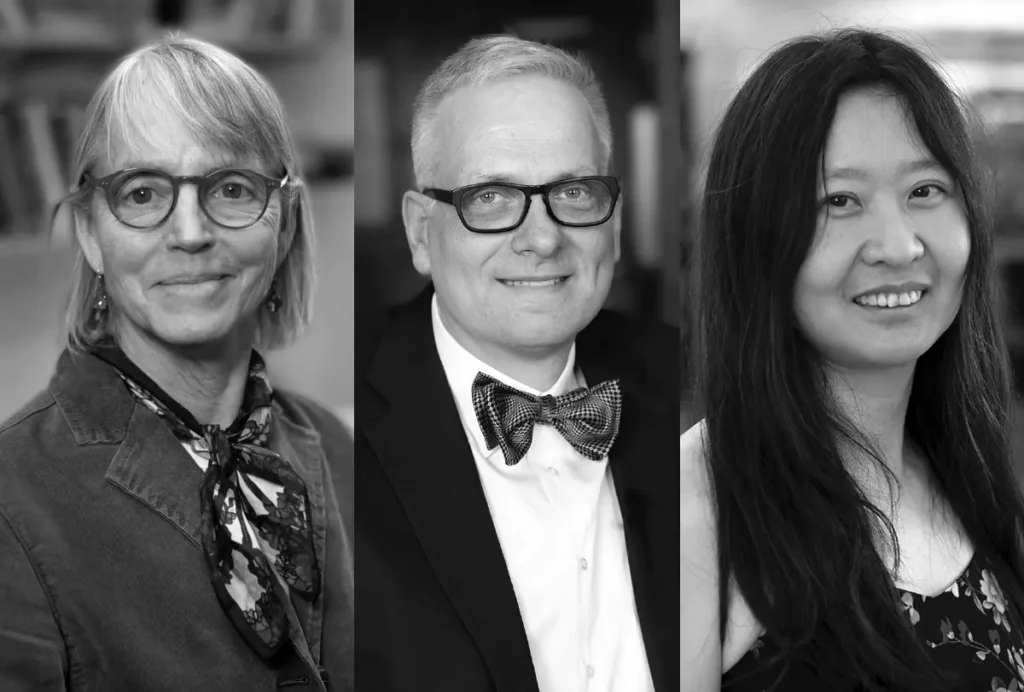
2024 Kavli Prize awarded for research on face-selective brain areas
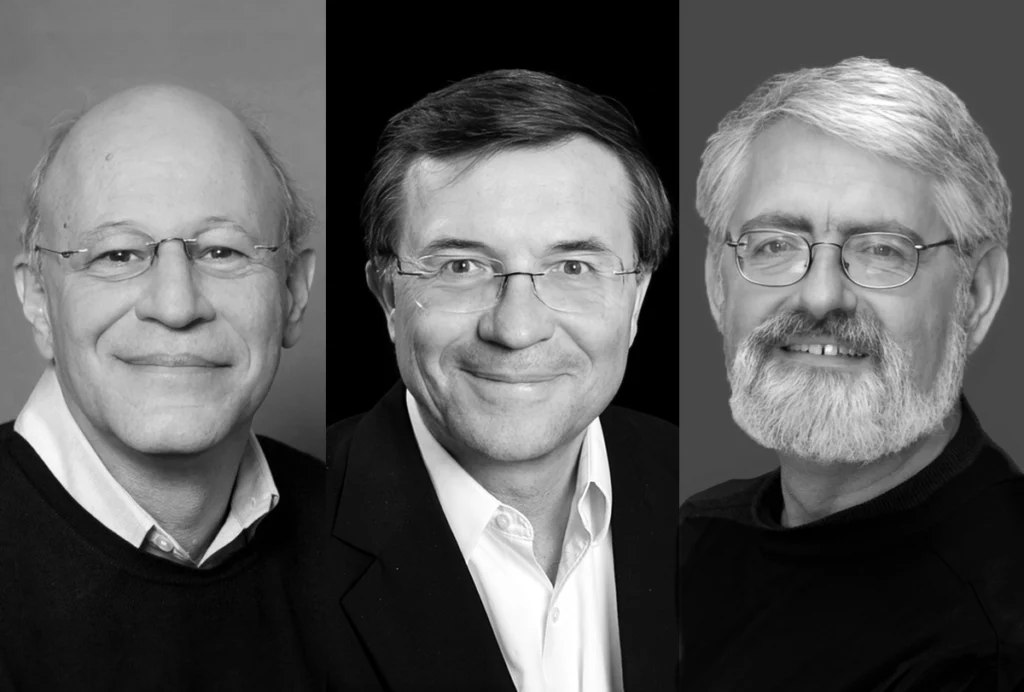
Theoretical and computational neuroscientists net 2024 Brain Prize
Explore more from The Transmitter
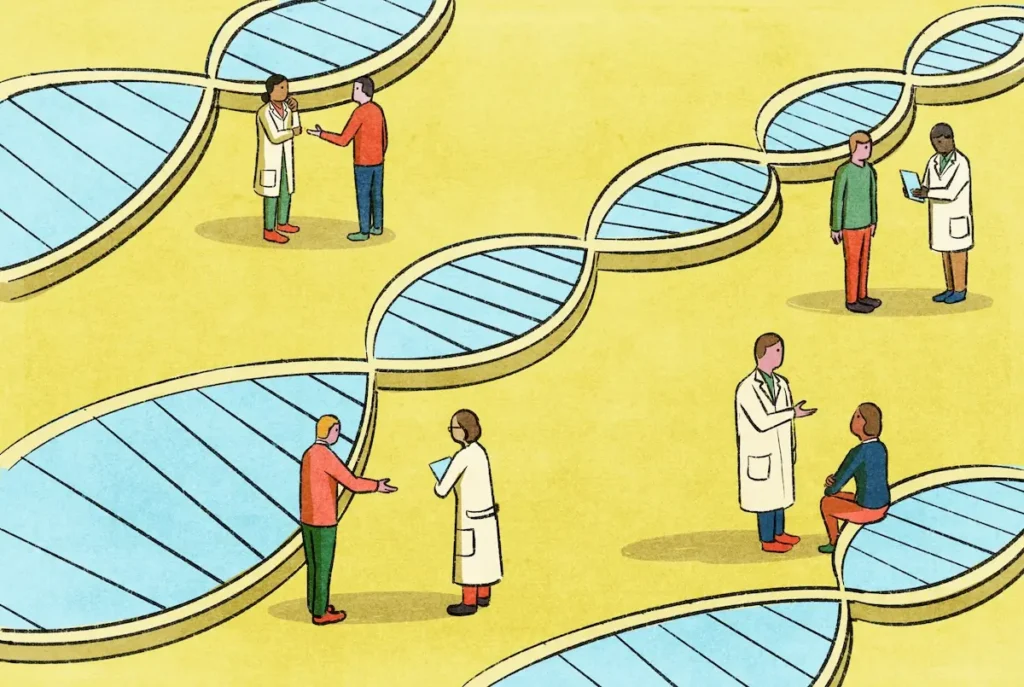
Building an autism research registry: Q&A with Tony Charman
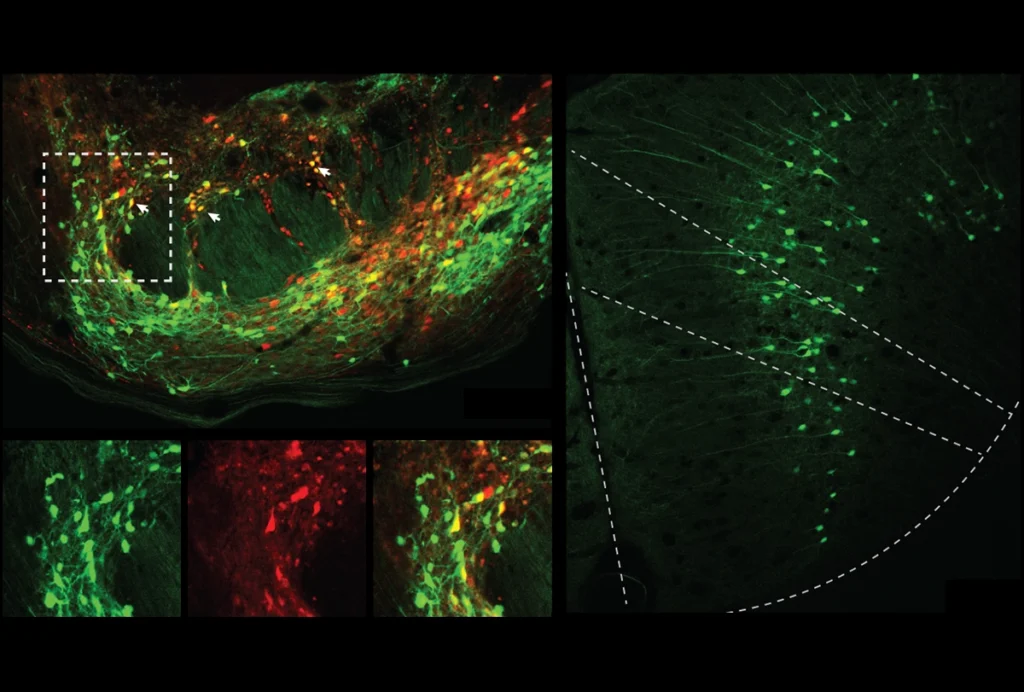