Earl K. Miller is Picower Professor of Neuroscience at the Massachusetts Institute of Technology, with faculty roles in the Picower Institute for Learning and Memory and the Department of Brain and Cognitive Sciences. His lab focuses on neural mechanisms of cognition, especially working memory, attention and executive control, using both experimental and computational methods. He holds a B.A. from Kent State University and an M.A. and Ph.D. from Princeton University. In 2020, he received an honorary Doctor of Science degree from Kent State University.
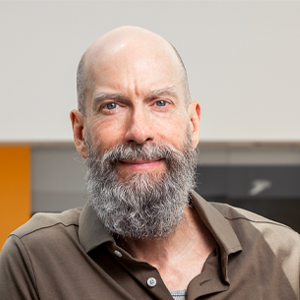
Earl K. Miller
Professor of neuroscience
Massachusetts Institute of Technology
Selected articles
- “An integrative theory of prefrontal cortex function” | Annual Review of Neuroscience
- “Top-down versus bottom-up control of attention in the prefrontal and posterior parietal cortices” | Science
- “The importance of mixed selectivity in complex cognitive tasks” | Nature
- “Gamma and beta bursts during working memory readout suggest roles in its volitional control” | Nature Communications
Explore more from The Transmitter
Federal funding cuts imperil next generation of autism researchers
As the International Society for Autism Research’s annual meeting begins, its next president reflects on a brewing crisis.
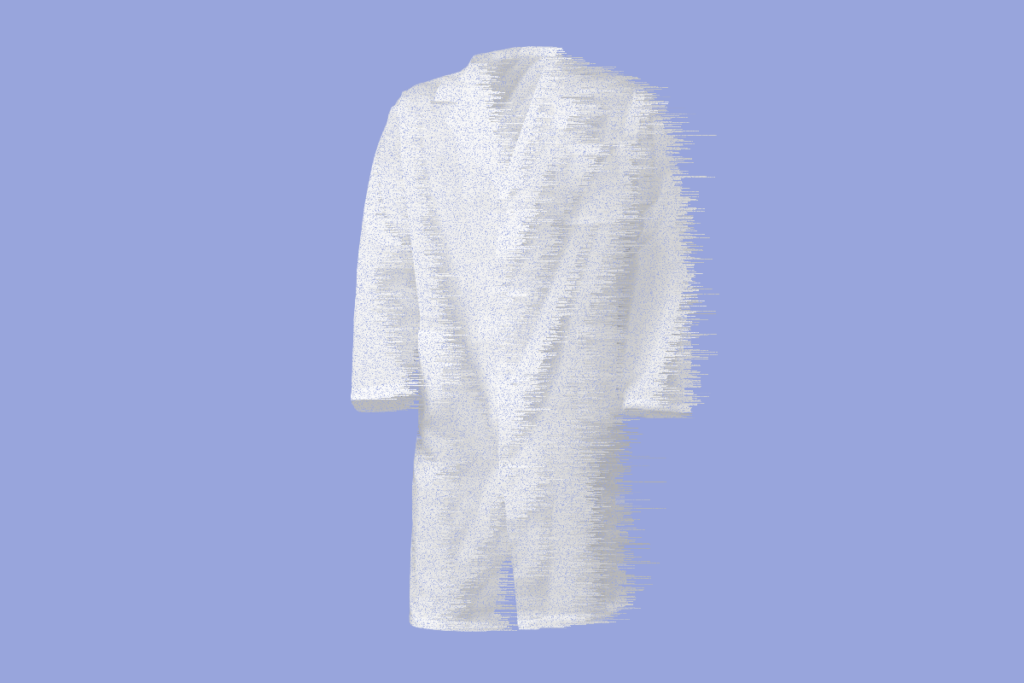
Federal funding cuts imperil next generation of autism researchers
As the International Society for Autism Research’s annual meeting begins, its next president reflects on a brewing crisis.
Null and Noteworthy: Reanalysis contradicts report of immune memory in astrocytes
The analysis, which has not yet been peer reviewed, attributes the finding to misidentified immune cells instead.
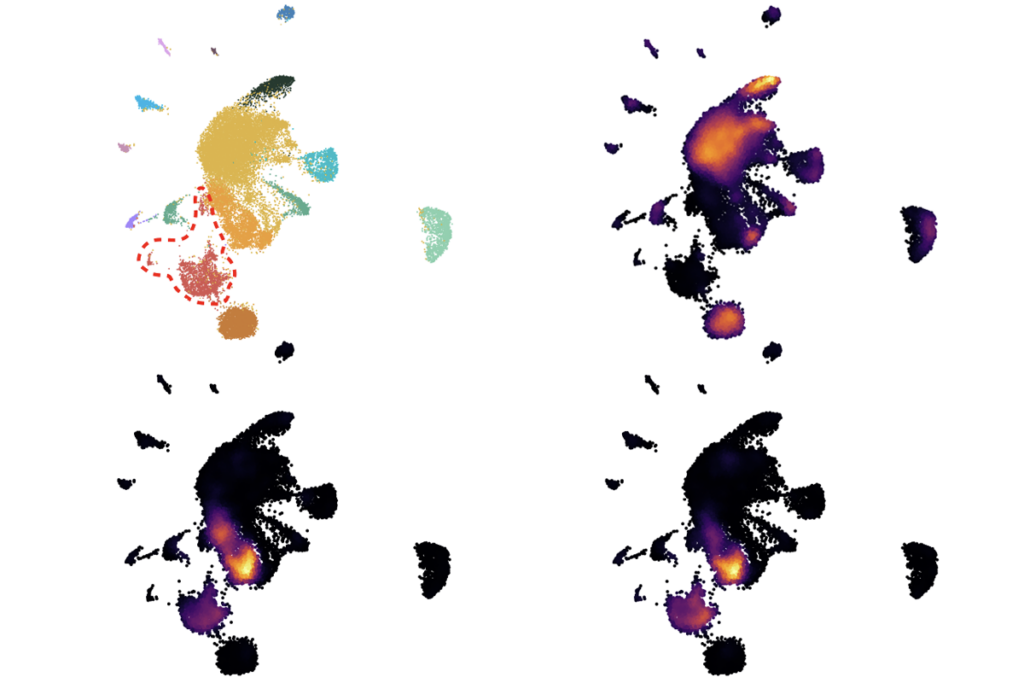
Null and Noteworthy: Reanalysis contradicts report of immune memory in astrocytes
The analysis, which has not yet been peer reviewed, attributes the finding to misidentified immune cells instead.
Documenting decades of autism prevalence; and more
Here is a roundup of autism-related news and research spotted around the web for the week of 28 April.
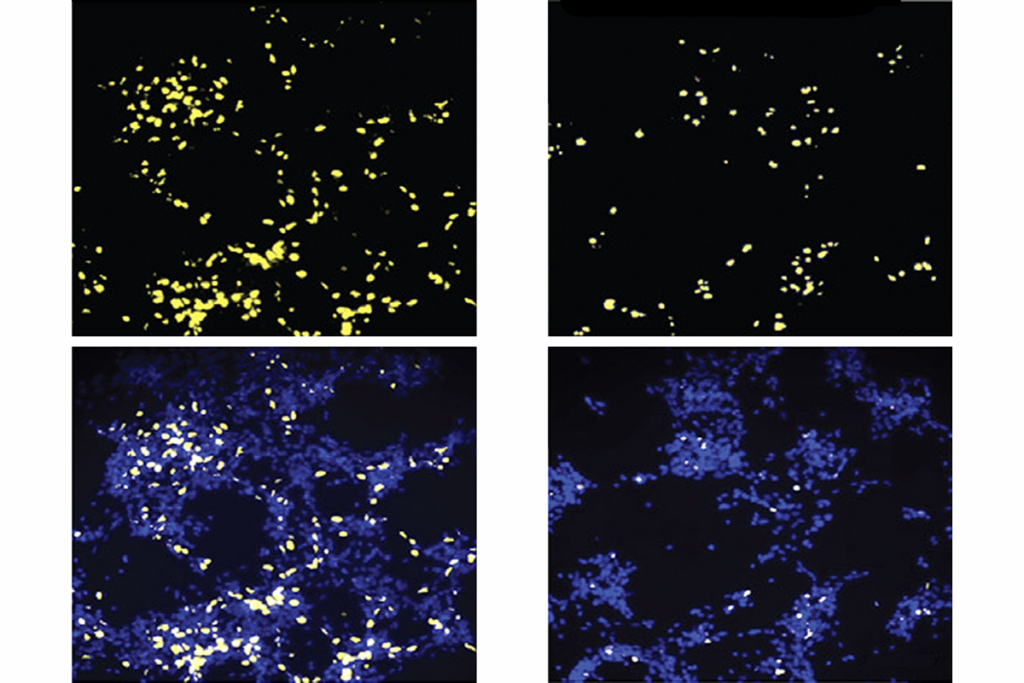
Documenting decades of autism prevalence; and more
Here is a roundup of autism-related news and research spotted around the web for the week of 28 April.