Felicia Davatolhagh is a postdoctoral researcher at the University of California, Los Angeles in Anne Churchland’s lab, where she studies how cortical circuits are altered during decision-making in a genetic mouse model of autism. She also serves as a member of the neurobiology department’s Justice, Diversity and Inclusion (JEDI) group.
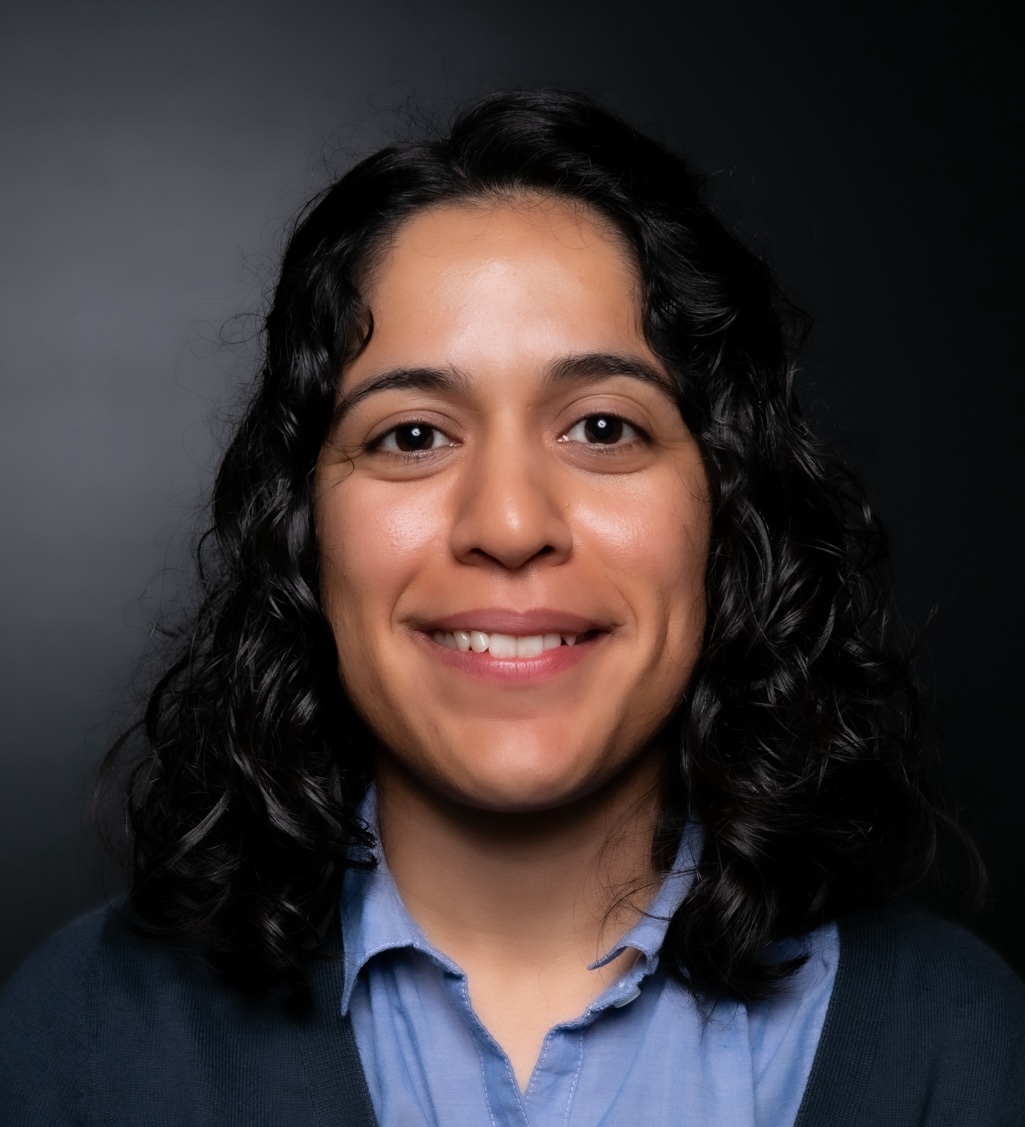
Felicia Davatolhagh
Postdoctoral researcher
University of California, Los Angeles
From this contributor
Women are systematically under-cited in neuroscience. New tools can change that.
An omitted citation in a high-profile paper led us to examine our own practices and to help others adopt tools that promote citation diversity.
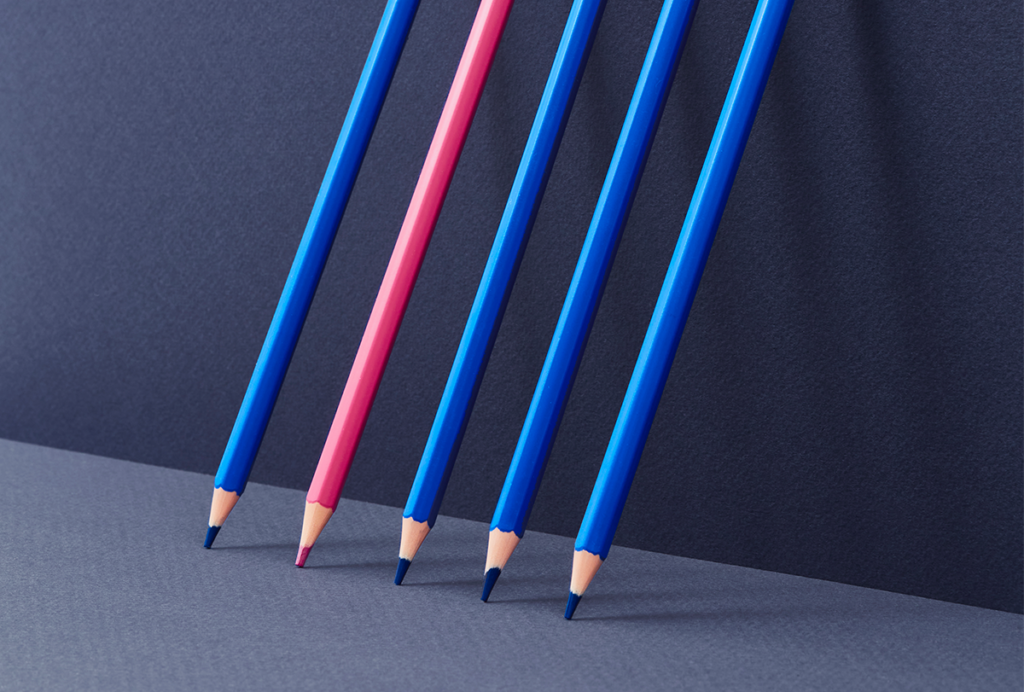
Women are systematically under-cited in neuroscience. New tools can change that.
Explore more from The Transmitter
Null and Noteworthy: Neurons tracking sequences don’t fire in order
Instead, neurons encode the position of sequential items in working memory based on when they fire during ongoing brain wave oscillations—a finding that challenges a long-standing theory.
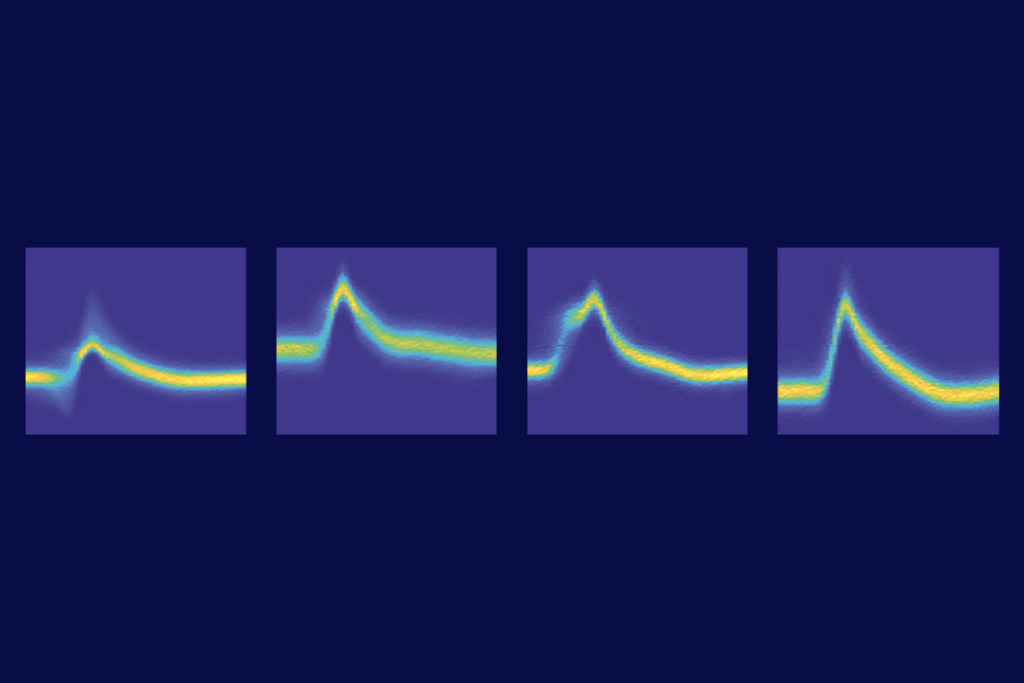
Null and Noteworthy: Neurons tracking sequences don’t fire in order
Instead, neurons encode the position of sequential items in working memory based on when they fire during ongoing brain wave oscillations—a finding that challenges a long-standing theory.
How to teach this paper: ‘Neurotoxic reactive astrocytes are induced by activated microglia,’ by Liddelow et al. (2017)
Shane Liddelow and his collaborators identified the factors that transform astrocytes from their helpful to harmful form. Their work is a great choice if you want to teach students about glial cell types, cell culture, gene expression or protein measurement.
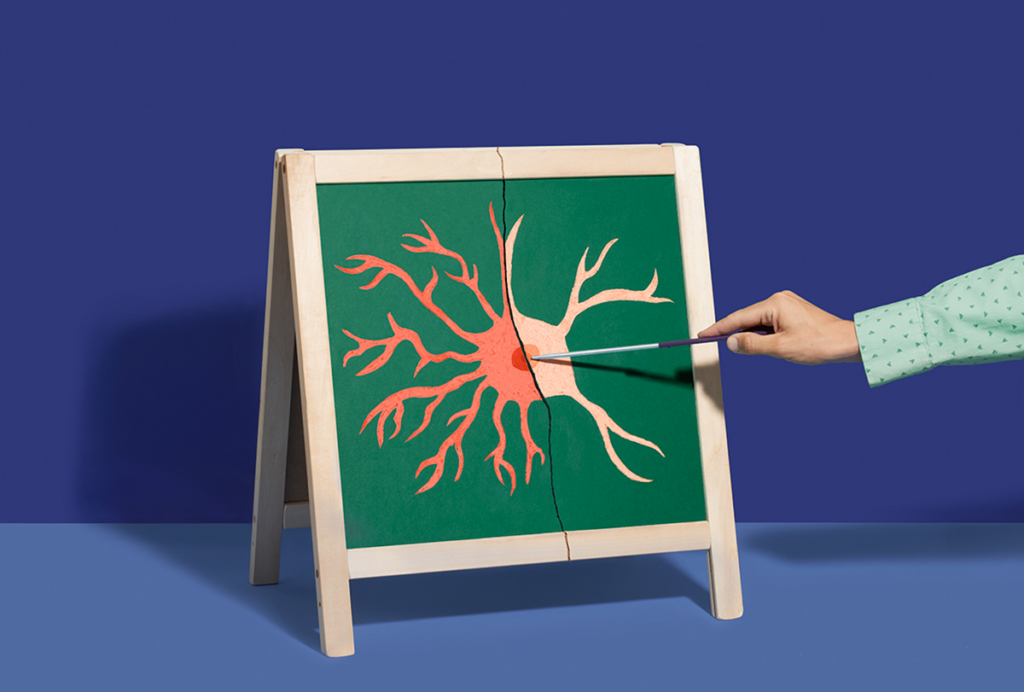
How to teach this paper: ‘Neurotoxic reactive astrocytes are induced by activated microglia,’ by Liddelow et al. (2017)
Shane Liddelow and his collaborators identified the factors that transform astrocytes from their helpful to harmful form. Their work is a great choice if you want to teach students about glial cell types, cell culture, gene expression or protein measurement.
Astrocytes sense neuromodulators to orchestrate neuronal activity and shape behavior
Astrocytes serve as crucial mediators of neuromodulatory processes previously attributed to direct communication between neurons, four new studies show.
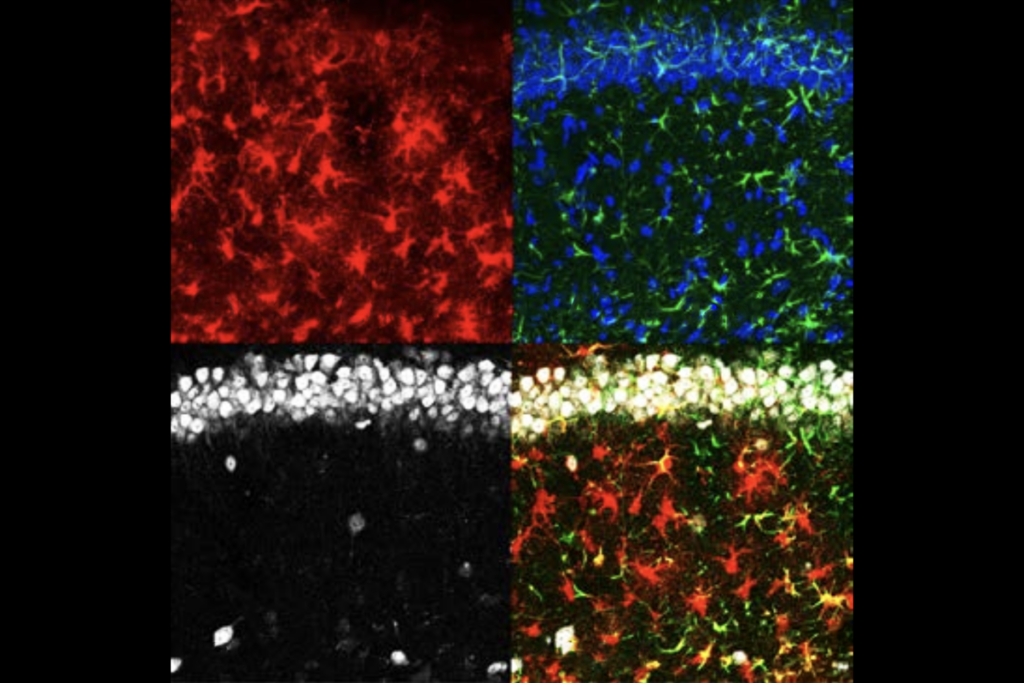
Astrocytes sense neuromodulators to orchestrate neuronal activity and shape behavior
Astrocytes serve as crucial mediators of neuromodulatory processes previously attributed to direct communication between neurons, four new studies show.