Breaking down the winner’s curse: Lessons from brain-wide association studies
We found an issue with a specific type of brain imaging study and tried to share it with the field. Then the backlash began.
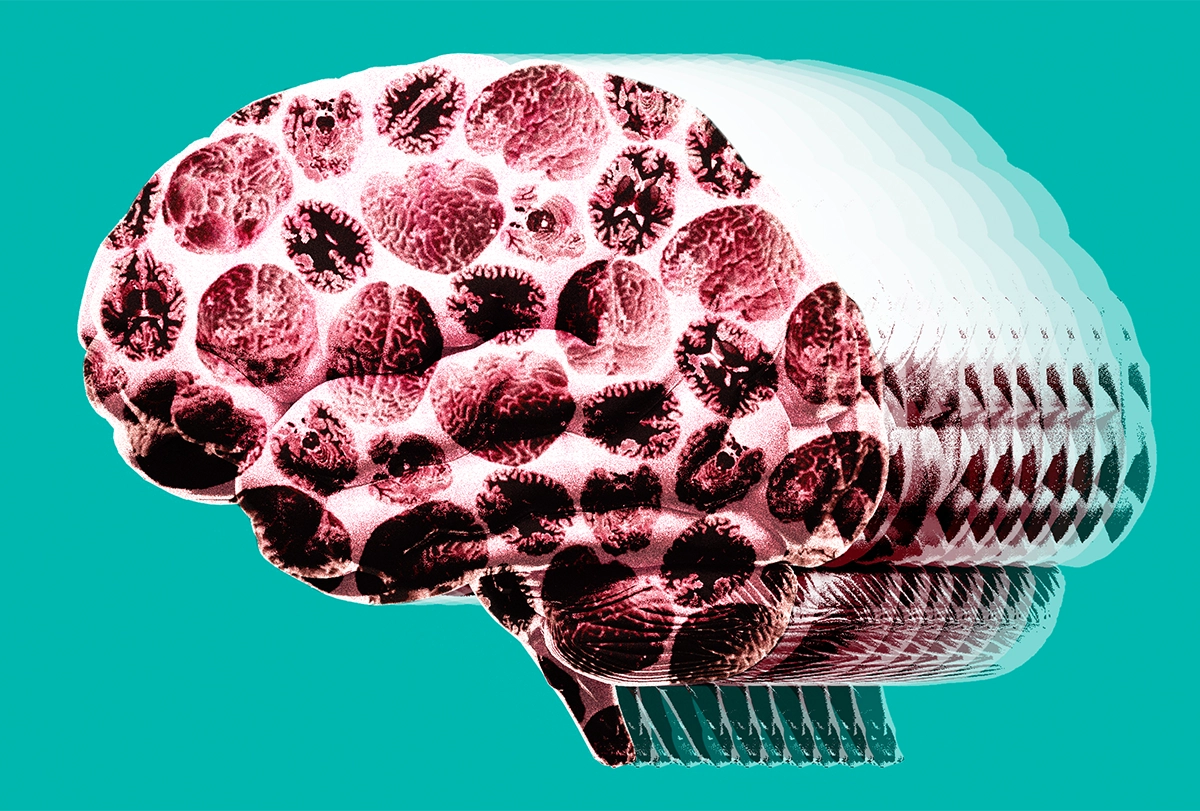
In 2022, we caused a stir when, together with Brenden Tervo-Clemmens and Damien Fair, we published an article in Nature titled “Reproducible brain-wide association studies require thousands of participants.” The study garnered a lot of attention—press coverage, including in Spectrum, as well as editorials and commentary in journals. In hindsight, the consternation we caused in calling for larger sample sizes makes sense; up to that point, most brain imaging studies of this type were based on samples with fewer than 100 participants, so our findings called for a major change.
But it was an eye-opening experience that taught us how difficult it is to convey a nuanced scientific message and to guard against oversimplifications and misunderstandings, even among experts. Being scientific is hard for human brains, but as an adversarial collaboration on a massive scale, science is our only method for collectively separating how we want things to be from how they are.
The paper emerged from an analysis of the Adolescent Brain Cognitive Development (ABCD) Study, a large longitudinal brain-imaging project. Starting with data from 2,000 children, Scott showed that an average brain connectivity map he made using half of the large sample replicated almost perfectly in the other half. But when he mapped the association between resting-state activity—a measure of the brain during rest—and intelligence in two matched sets of 1,000 children, he found large differences in the patterns. Even with a sample size of 2,000—large in the human brain imaging world—the brain-behavior maps showed poor reproducibility.
For card-carrying statisticians, the result was not surprising. It reflected a pattern known as the winner’s curse, namely that large cross-sectional correlations can occur by chance in small samples. Paradoxically, the largest correlations will be “statistically significant” and therefore most likely to be published, even though they are the most likely to be wrong.
Smaller-than-expected effect sizes were old news in genome-wide association studies (GWAS), which had been forced more than a decade ago to push their sample sizes higher, eventually into the millions. But the neuroimaging field lagged behind—despite warnings about sampling variability in 2009 from neuroimaging statistician Tal Yarkoni.
Our finding offered a clear example of the problem, and we felt it was important to share with the field. But it was a difficult message for the community to hear. Indeed, when we decided to publish the finding, prominent collaborators asked to be removed from the manuscript, because they did not want to be associated with its message. Bracing for more fallout, we started to call it the “Manhattan Project.”
W
e knew functional MRI (fMRI) researchers sensitized to bad press in the past might project their worst fears onto our article. Several high-profile papers in the field, including one on a dead salmon, have identified statistical issues with analyses of fMRI data, calling into question some published findings, sometimes overly broadly. So we took great pains with the abstract, praising the transformational power of fMRI research, for example. Our findings applied to a specific type of fMRI study, which we coined brain-wide association studies (BWAS), rather than to classical brain-mapping studies, and we tried to clearly outline the difference. Classical fMRI activation studies look for an association between a brain region, such as the visual cortex, and a behavior, such as watching a checkerboard, a typically large effect that can be detected in an individual. BWAS studies, in contrast, look for cross-sectional correlations between brain metrics and behavioral phenotypes, such as psychopathology. These correlations are typically much smaller and require much larger samples.But outlining this distinction wasn’t enough. The most common response we got was, “How can you say that all fMRI studies are wrong?” We never said anything like that—we are true believers in the awesome powers of fMRI—but this misconception ran deep. Even people well-versed in human brain imaging research conflated classical fMRI activation studies and BWAS. At an early presentation, for example, one person argued that our claims stood in conflict with interesting fMRI work in studies with sample sizes of 1 to 10. The small-sample precision fMRI mapping they were praising was, in fact, our own work.
Despite the fact that we defined BWAS in the manuscript, the misconception equating it with all fMRI research has persisted. Soon after publishing our study, we received a grant review quoting our BWAS, claiming that the proposed sample was too small. But the grant was for a study of within-patient interventions (pre/post neurosurgery), which have much larger effect sizes than BWAS and therefore do not require thousands of participants, as we explained clearly in the proposal. (Nico has since started tweeting “fMRI ≠ BWAS” every time there is an opening.)
Some press coverage, too, conflated fMRI and BWAS, including an editorial in another prominent journal, and our critics said we should have done more to influence journalists. We tried hard to explain all the nuance. One anti-misunderstanding technique we started using when presenting our work is to follow our most important arguments with another sentence to explicitly state what we are not saying.
T
wo years later, we still get skeptical questions about our BWAS article. Some researchers have argued for exceptions, for example that the strongest brain-wide associations can be replicable with sample sizes in the hundreds or that image processing and multivariate methods produce slightly larger BWAS effects. We disagree with these approaches. This continued focus on generating the largest BWAS effect in a small dataset only pulls us deeper into the winner’s curse paradox. Methods optimized for specific data or that highlight a single association create the ideal conditions for publication-bias-driven effect-size inflation.On the flip side, several presentations and preprints showcase efforts to further optimize BWAS with large sample sizes. Important work is underway to investigate the complex trade-offs between collecting more and better imaging, behavioral and other data per participant versus adding more participants. Other studies are evaluating the benefits of different population sampling schemes, such as overloading the tails of distributions for specific variables of interest, repeated and longitudinal sampling, and making samples more representative of the human population.
These BWAS optimization efforts are unlikely to yield magical shortcuts. When it comes to population neuroscience, or epidemiology with brain imaging, big representative samples will save us, not math magic in small samples. When it comes to fMRI or lesion studies, one participant may be all you need. fMRI ≠ BWAS.
Recommended reading
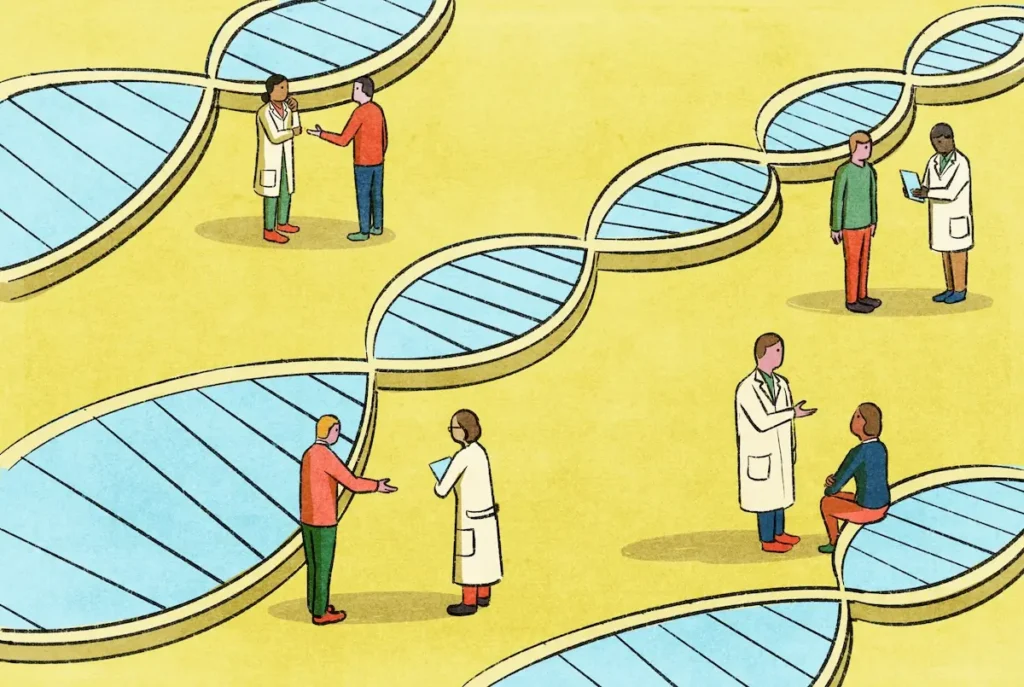
Building an autism research registry: Q&A with Tony Charman
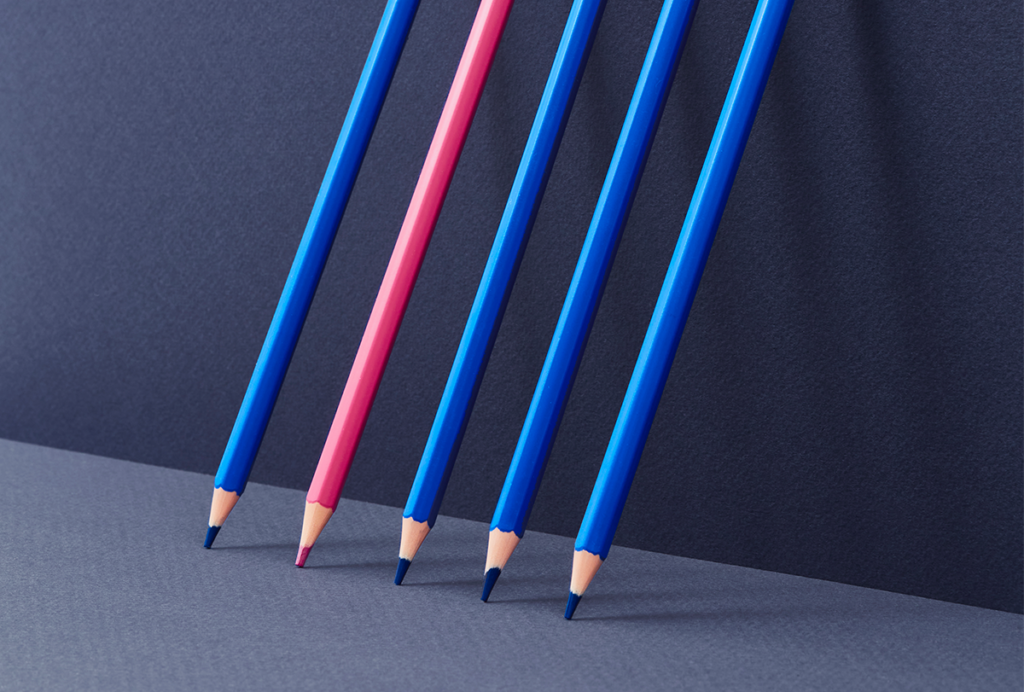
Women are systematically under-cited in neuroscience. New tools can change that.
Explore more from The Transmitter
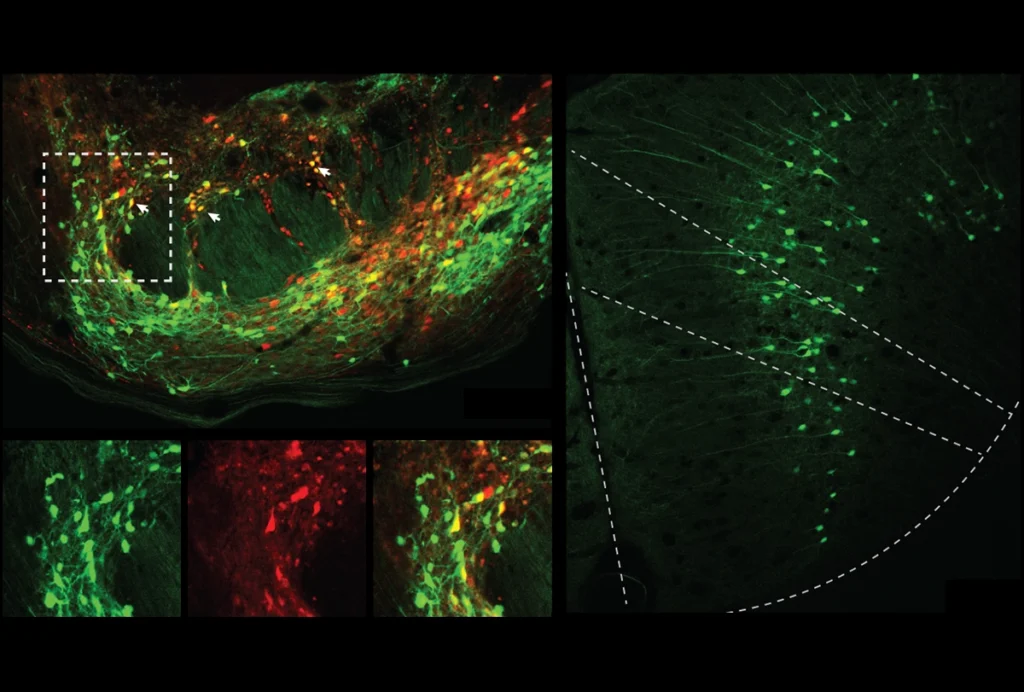
Cerebellar circuit may convert expected pain relief into real thing
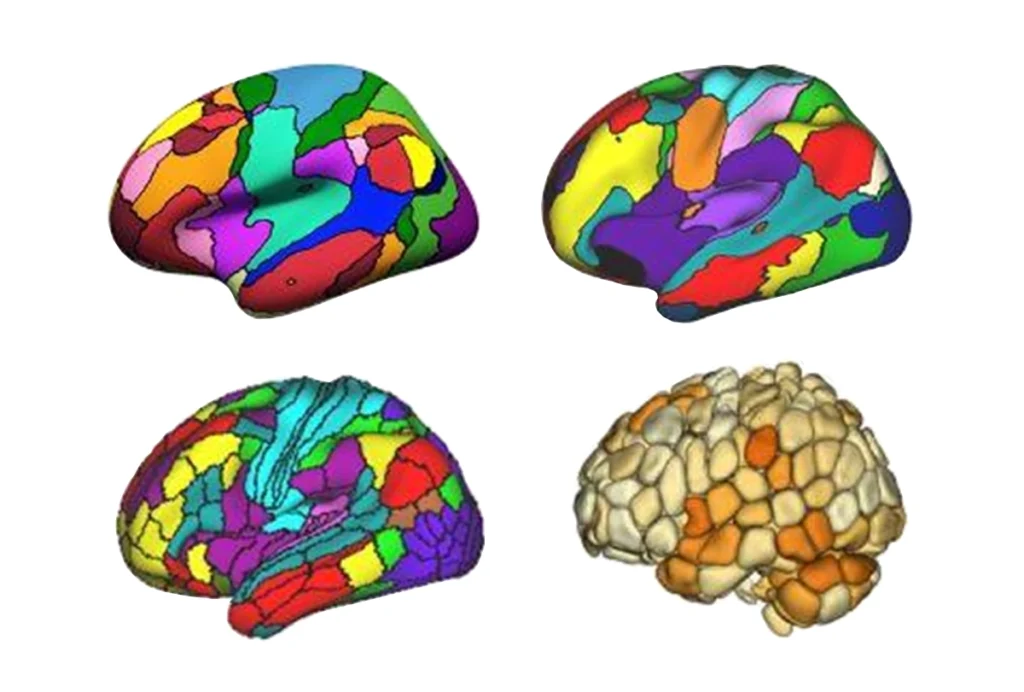