Incentivizing data-sharing in neuroscience: How about a little customer service?
To make data truly reusable, we need to invest in data curators, who help people enter the information into repositories.
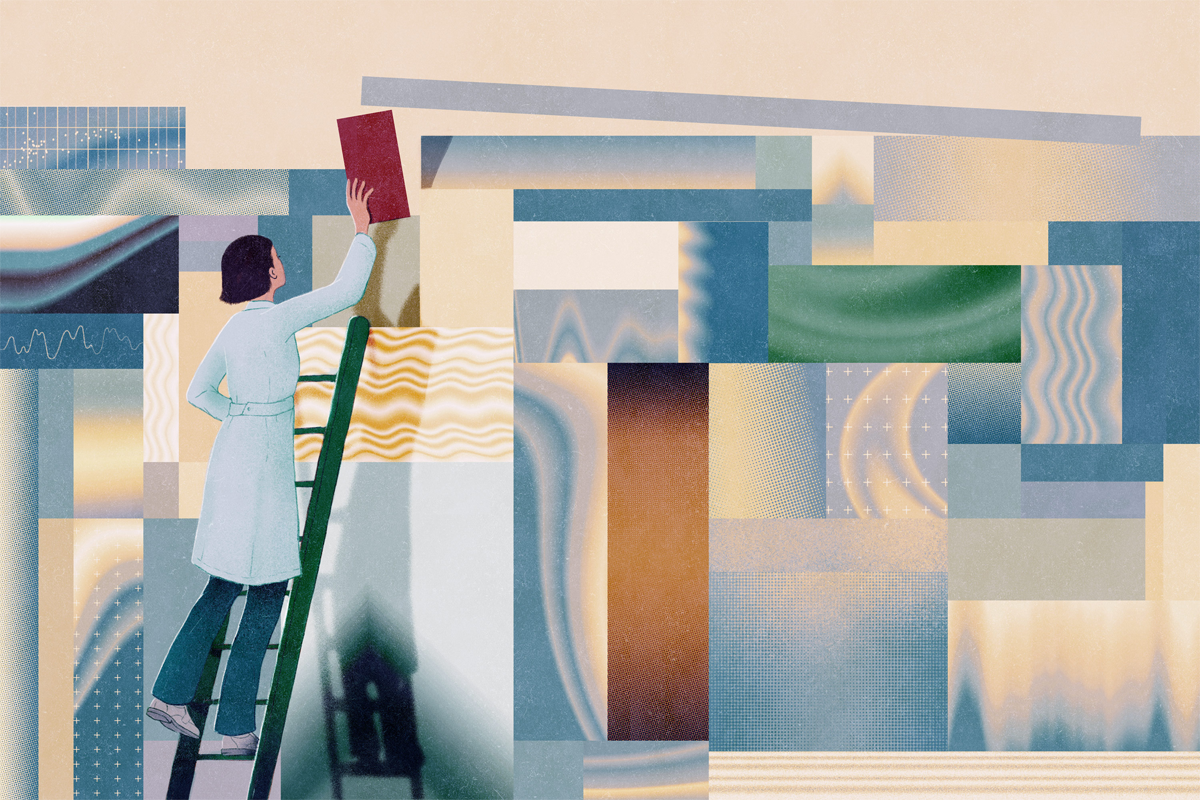
A growing number of funder and journal policies now require neuroscientists to share the data they produce. But to truly reap the benefits, data must be shared well — no easy feat in neuroscience, which generates a multiplicity of complex data types across spatial scales. What will motivate neuroscientists to spend the time and resources required to make their shared data truly useful to others? Because without adequate support, the new data-sharing policies are in danger of becoming simply a “box-checking” exercise.
If we really want to change the culture around data management and sharing, we must focus on improving the data submission experience. In these early, crucial days of wide-scale data-sharing, we must encourage repositories to take a more customer-service-oriented approach to data submission and offer the necessary support to do so. To be most effective, repositories must work to guide and assist researchers through the submission process and not merely point them to documentation or provide feedback about where they failed.
Thanks to investments in large brain projects and organizations such as the International Neuroinformatics Facility, neuroscience has many of the pieces in place to make shared data useful. Researchers have developed a set of guidelines for data reuse, known as “FAIR”: findable, accessible, interoperable and reusable. And neuroscience-specific repositories exist to serve specific data types, neuroscience domains or geographical regions. In recent years, these investments have also spurred the development and adoption of neuroscience-specific standards such as the Brain Imaging Data Structure (BIDS), Neurodata Without Borders, the National Institutes of Health’s Common Data Elements and Common Coordinate frameworks. By supporting these standards, repositories are seeding an ecosystem of tools around particular data types.
But for this infrastructure to pay off, data producers have to be both willing and able to populate these resources with standardized, well-curated data. The fact remains that preparing data for a repository, especially one that requires strict adherence to data and metadata standards, is a significant burden that falls asymmetrically on the investigator. If the burden is perceived to be too great relative to the rewards, researchers have the option to go elsewhere, such as a generalist repository with few submission requirements. Indeed, some repositories have lowered their requirements to ensure they still have customers, but the data they house are far less useful.
Fully preparing data for publication requires skills that researchers rarely possess. Data producers typically have a deep understanding of the data but not the mindset, knowledge or resources to adhere to data and metadata standards that optimize data for reuse. Researchers often submit poor-quality metadata, for example, as work from my group and others has shown. I don’t believe that simply providing researchers with better training in data management or data science will solve the problem.
Instead, we need to deploy professionals, such as knowledge engineers and data curators. Curators can format and document data according to the standards in place at a specific data repository. They can review submitted data, engage with the submitters where necessary to ensure compliance and often provide additional services, such as mapping metadata to controlled vocabularies or tagging data with keywords.
A
few neuroscience repositories, such as EBRAINS (previously the European Human Brain Project Neuroinformatics Platform), Stimulating Peripheral Activity to Relieve Conditions (SPARC), the Open Data Commons for Spinal Cord Injury and the Open Data Commons for Traumatic Brain Injury, have blazed a trail and already invested in curators to improve the consistency and quality of submitted data. Informal surveys show that investigators are often surprised by the positive impact curation has on their work — their data are now “FAIR,” not only to the community, but to the originating lab. When a postdoctoral researcher leaves, their data can be reliably found, accessed and understood. And as researchers work with curators, they start to appreciate how the requirements of a repository — including the use of identifiers, metadata, specific standards and data dictionaries — serve data management overall, and they begin to develop practices within their own laboratories to facilitate sharing.If researchers are supported properly, submitting their data to specialized neuroscience repositories provides practical training to empower effective sharing across the data lifecycle. It catalyzes a feedback cycle in which benefits, tools and knowledge flow back from the repository to the submitting laboratory and back out to other users, whether human or artificial intelligence, in the form of higher-quality, FAIR data. Everybody wins.
”Fully preparing data for publication requires skills that researchers rarely possess.
My experience suggests this approach is effective. SPARC has some fairly stringent data requirements; because the NIH project collects high-quality and varied data on the interaction of the autonomic nervous system with end organs, it uses a cross-modality data standard called the SPARC Data Structure, based on BIDS, to organize the variety of data submitted. In the early days, scientists submitting data found the process frustrating and labor intensive, leading to many angry emails. While the technical team worked to improve the infrastructure, the curators worked to establish good relationships with the investigators, acknowledging when the process was difficult and assisting them over any barriers. Over time, curators observed that establishing a respectful, supportive relationship with data submitters rendered their experience much less burdensome. And despite early significant frustrations, when surveyed, many investigators indicated that they intend to continue using SPARC as their data-sharing platform even after their SPARC-specific funding ends.
Human curation is expensive and hard to scale, and funders are often reluctant to pay for it. But I don’t believe that this level of human support will be needed forever. In the SPARC project, for example, a young investigator, Bhavesh Patel, seeing the effort required to organize and upload data, developed a software wizard called SODA to automate file-level operations and to guide the researcher step by step through the process. As researchers started to understand what was being asked of them and began using SODA, the process became more efficient for both submitters and curators. We can expect the rapid advance of AI to have a significant impact on curation, data integration and other data challenges.
But in the meantime, we need good data, and that will come from well-curated, standardized and well-managed data in specialist repositories. Reducing the burden on the data submitter, not by lowering requirements but by investing in customer-service-oriented curation, will go a long way toward unleashing the full power of data science on this most complex of organs, the brain.
Disclosure: Martone is on the board of directors and has equity interest in SciCrunch Inc, a tech startup out of the University of California, San Diego that develops tools and services for reproducible science.
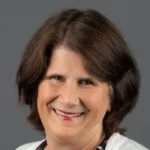
Recommended reading
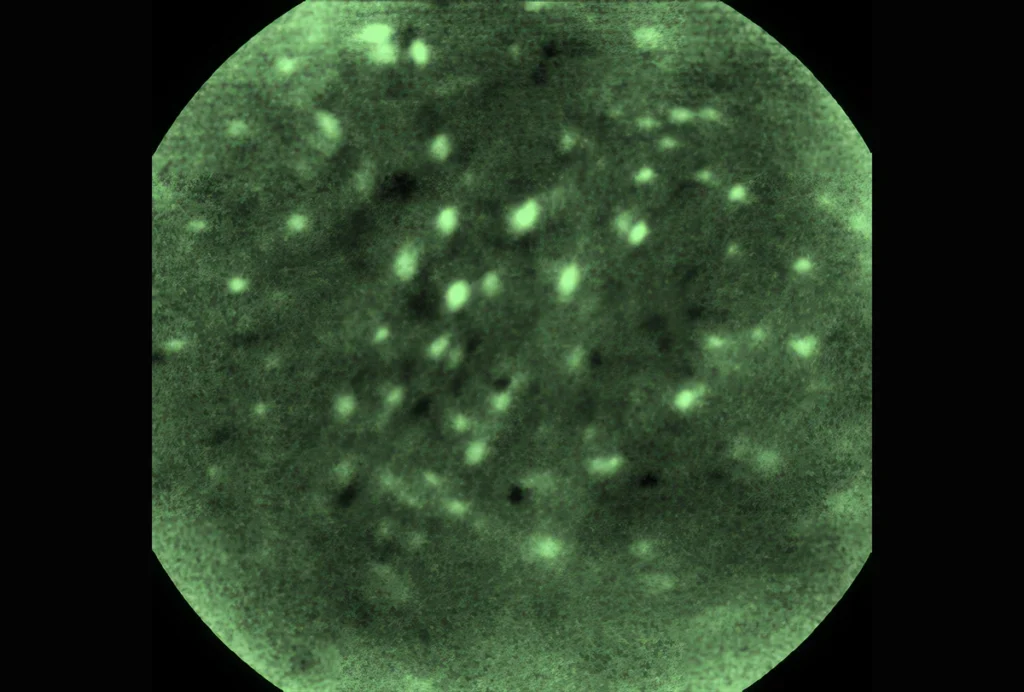
Designing an open-source microscope
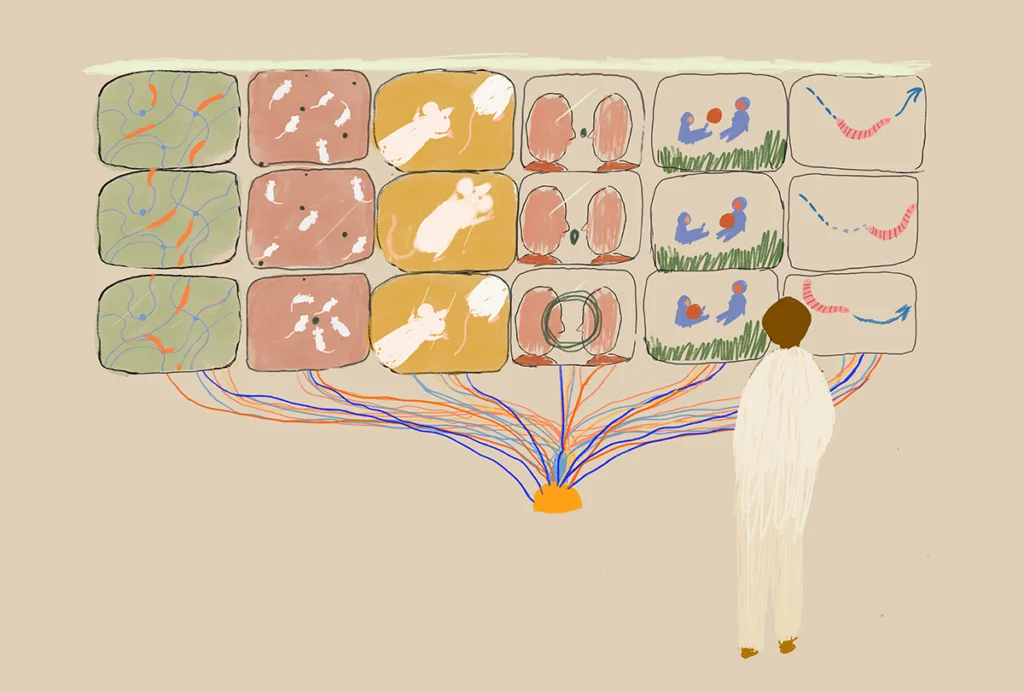
Neuroscience needs a research-video archive
Explore more from The Transmitter
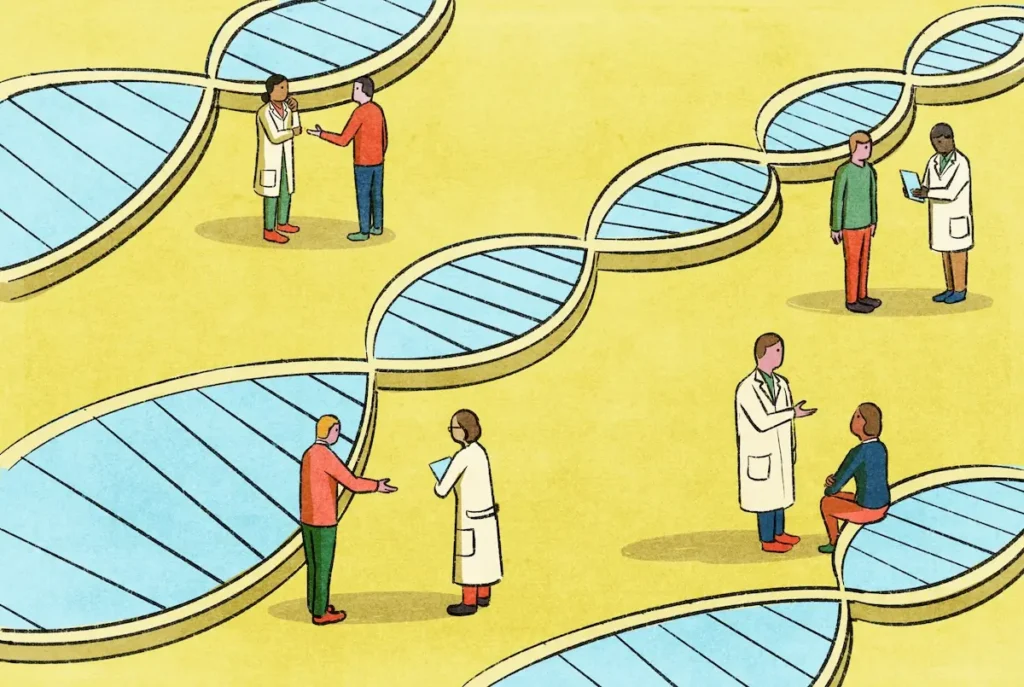
Building an autism research registry: Q&A with Tony Charman
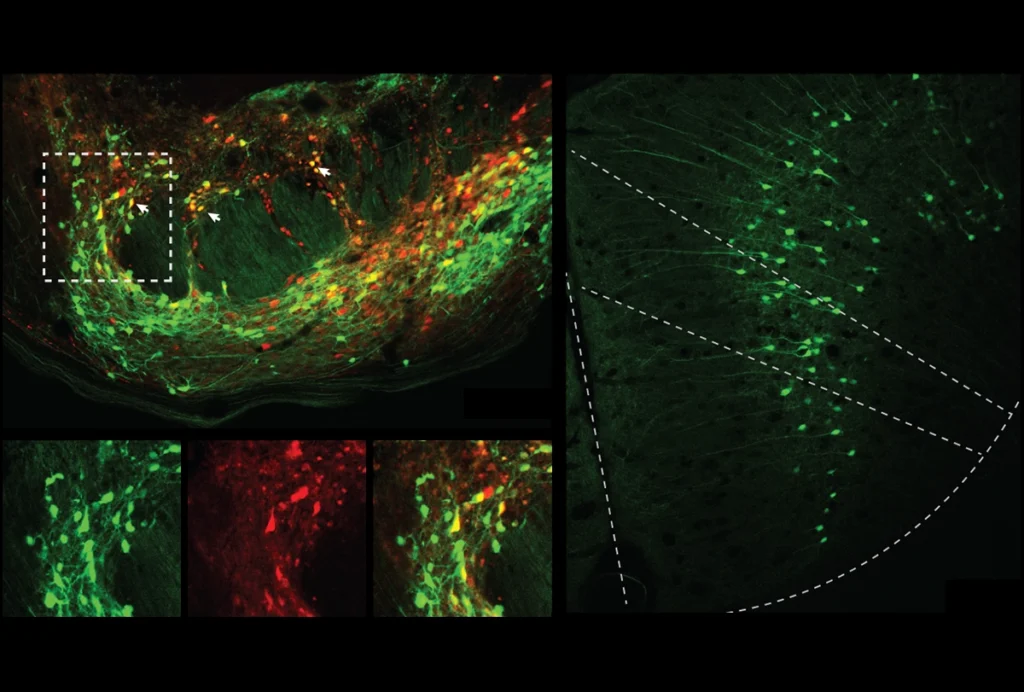