Brain scans may soon paint personal pictures of mental health
Statistical techniques and information about the timing of brain activity are laying the groundwork for practical applications of brain imaging.

As a scientist who routinely scans people’s brains, I find it difficult to remain blasé about a technology that enables me to peer inside the human brain, giving me a glimpse of its structure and function.
Noninvasive brain imaging provides insights into our senses, what we see and hear. The scans may enable us to predict a person’s performance on a task or test, by showing telltale activity levels in certain areas.
Scientists have even used them to reconstruct what people are watching on a movie screen, decoding the cogs and wheels of visual perception. In the courtroom, judges have considered scans of an alleged perpetrator’s brain during sentencing. In some cases, a brain scan may reveal an obvious neurological problem that might mitigate the sentence.
Clinically, brain activity patterns may help explain or identify neurological conditions such as schizophrenia, traumatic brain injury, autism or Alzheimer’s disease. In fact, across a range of conditions, researchers have been able to pinpoint an individual’s psychiatric diagnosis from such a scan. Averages across several studies suggest that these diagnoses are accurate 74 percent of the time for attention deficit hyperactivity disorder and 87 percent of the time for autism, though more studies are needed to confirm these results1.
Such practical applications of the technology are ethically and legally controversial — in part because brain imaging is still in its infancy. One of the biggest issues surrounding various practical uses of the technology is that, despite amazing advances in knowledge, we do not yet fully understand what the brain scans are telling us.
But a groundswell of technical advances is giving us new insights into brain scans that should enable us to use them more widely in the clinic, courtroom and other settings. The advances include new statistical techniques, combination approaches and methods that capture changes in neural activity over time. Meanwhile, ongoing collaborative efforts such as the Autism Brain Imaging Data Exchange (ABIDE) and the Human Connectome Project are generating larger datasets and more high-quality brain imaging data.
Capturing conditions:
Current brain imaging experiments have basic pitfalls — such as the lack of replication of results1. Most studies to date have relatively small sample sizes — about 20 to 30 participants is the norm — which makes it difficult to obtain reliable results. Movement in the scanner can also distort images.
However, perhaps the largest problem we face is the lack of specificity.
Most studies compare a group of people who have a condition with a group of unaffected individuals. As a result, researchers cannot determine whether the identified differences in the brain arise from the condition in question or from a feature that marks any unhealthy brain.
At the same time, diagnostic categories can be controversial and difficult to define. For instance, autism is a diverse condition with myriad subtypes, making it hard to find a particular brain signature for the condition as a whole.
There are several approaches that hold great promise for bringing brain imaging technology to the point where it is more practically useful. One is the use of techniques that can help us identify meaningful patterns in the data and potentially predict how rapidly a condition might progress.
This approach is especially critical given individual variations in brain anatomy and function. Various machine-learning approaches can identify patterns, but one widely used approach to identify brain networks is an advanced statistical approach called independent component analysis2.
These statistical techniques allow scientists to decode brain scans at various levels of resolution, from individual data points, or ‘voxels,’ to brain networks and groups of networks. Researchers can then combine the data at each level to create a comprehensive picture of brain function.
Critical combinations:
Another promising approach is ‘multimodal imaging,’ which combines complementary brain imaging techniques. Because every imaging method is imperfect, combining methods could provide a more complete picture of the brain and potentially improve scientists’ ability to make predictions about individuals3.
For example, by including information about brain activity during diverse tasks as well as measurements of brain volume and the connections between regions, we can provide a deeper understanding of how conditions such as autism affect the brain.
The BRAIN Initiative, a project sponsored by the National Institutes of Health (NIH), is aimed at building a detailed picture of neural activity across the brain. It may also offer new technologies that enable us to probe the brain in unprecedented detail. Moving from a macroscopic view to a microscopic one may reveal new information about the biological mechanisms underlying brain conditions.
Meanwhile, we are refining methods that capture how brain regions interact, by detecting their simultaneous activation, or ‘connectivity4.’
For many years, functional connectivity measurements were based on an average of data collected over 5 to 15 minutes of scanning. We have since realized, however, that we can glean more information about connectivity if we use approaches that capture patterns of connectivity that vary over time, the so-called ‘chronnectome.’
By using such dynamic connectivity approaches, we have substantially improved the accuracy of the information we glean about the brain in various conditions. For example, we have used this technique to show that people with schizophrenia tend to get ‘stuck’ in brain states longer than other people do5.
This information about the timing of brain activity may provide important clues to a condition. For example, an approach using dynamic connectivity has already suggested that individuals with features of autism show typical patterns of brain connectivity for shorter periods of time than controls6.
Big-picture projects:
Where all of these brain imaging data end up is important. Encouragingly, the brain imaging field has begun to move toward a culture of open science, in which research groups freely share data. There is still a long way to go before such sharing is commonplace, but several large collaborative projects are helping to greatly expand the quantity, and improve the quality, of brain imaging data.
For example, the Human Connectome Project is a five-year effort to characterize the human connectome, the pattern of connections in a typical brain. In this effort, several labs, funded by the NIH, are amassing large numbers of brain scans from the general population and collecting them in a common database. Spinoffs of this project aim to identify patterns of brain connections that characterize certain conditions, such as autism.
Other grass-roots approaches include the 1000 Functional Connectomes Project, in which investigators from around the world contribute functional magnetic imaging datasets to a repository, and its autism offshoot, ABIDE, which is a collection of data from various autism imaging studies.
Combined with technological progress in the field, these collaborative projects have the potential to transform our understanding of the human brain and how conditions such as autism affect it. It is only a matter of time before advances in brain imaging lead to tools that clarify diagnoses and guide clinicians toward the best treatment for individuals.
- Arbabshirani M. et al. Neuroimage Epub ahead of print PubMed
- Calhoun V.D. and T. Adali IEEE Rev. Biomed. Eng. 5, 60-73 (2012) PubMed
- Calhoun V.D. and J. Sui Biol. Psychiatry Cogn. Neurosci. Neuroimaging 1, 230-244 (2016) PubMed
- Calhoun V.D. and T. Adali IEEE Signal Process. 33, 52-66 (2016) Abstract
- Miller R.L. et al. PLOS ONE 11, e0149849 (2016) PubMed
- Rashid B. et al. (2016, June). A pediatric population-based resting state study of connectivity dynamics in typical development. Poster session presented at the meeting of the Organization for Human Brain Mapping, Geneva, Switzerland Full text
Recommended reading
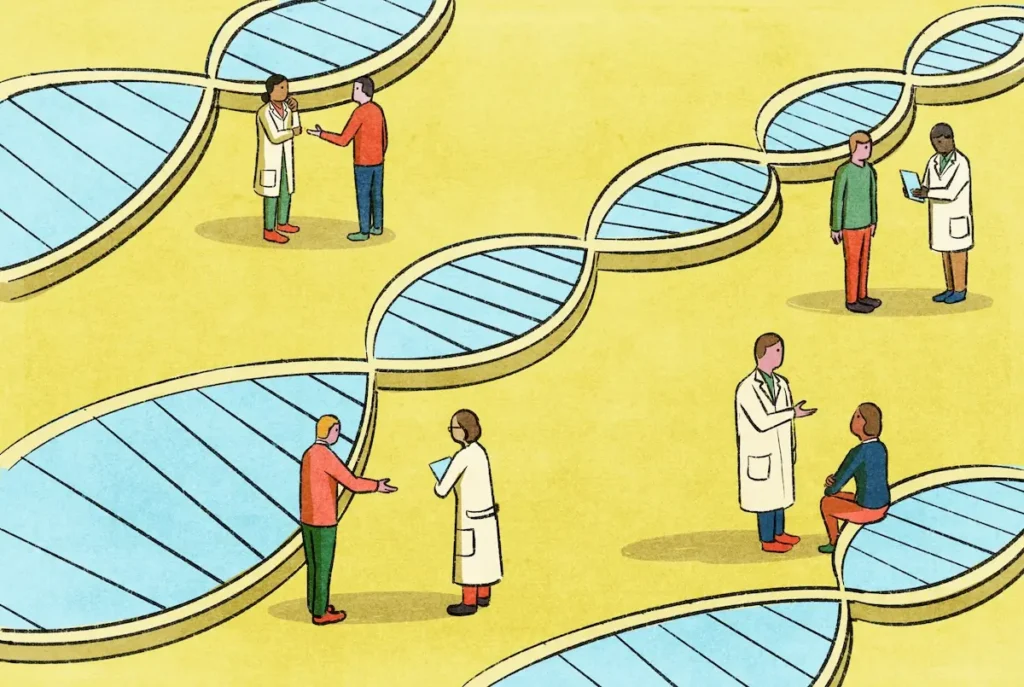
Building an autism research registry: Q&A with Tony Charman
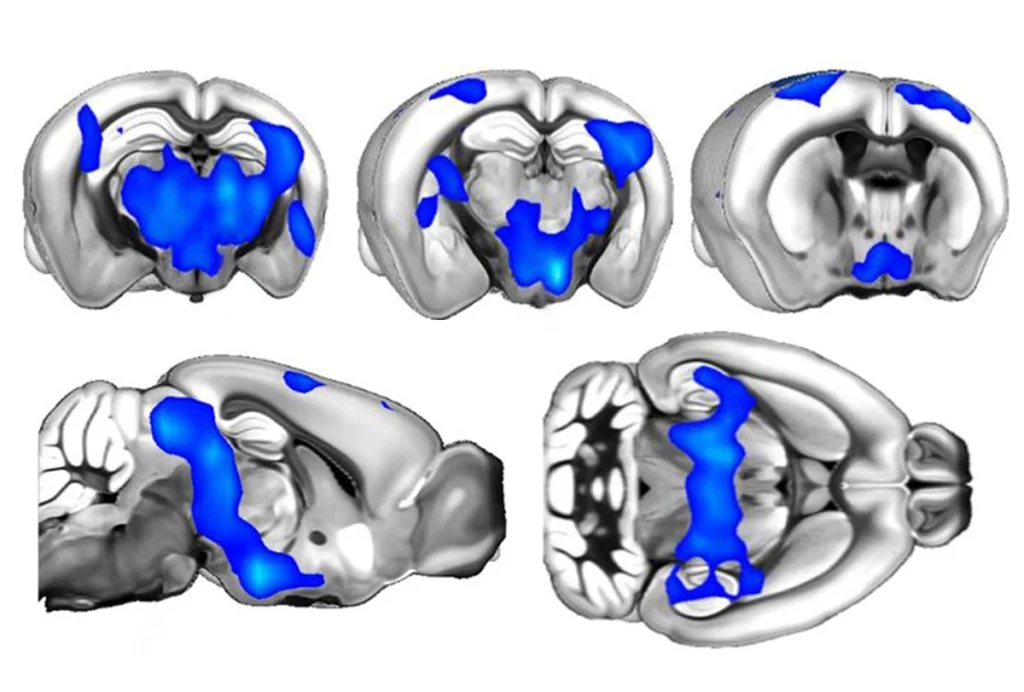
CNTNAP2 variants; trait trajectories; sensory reactivity
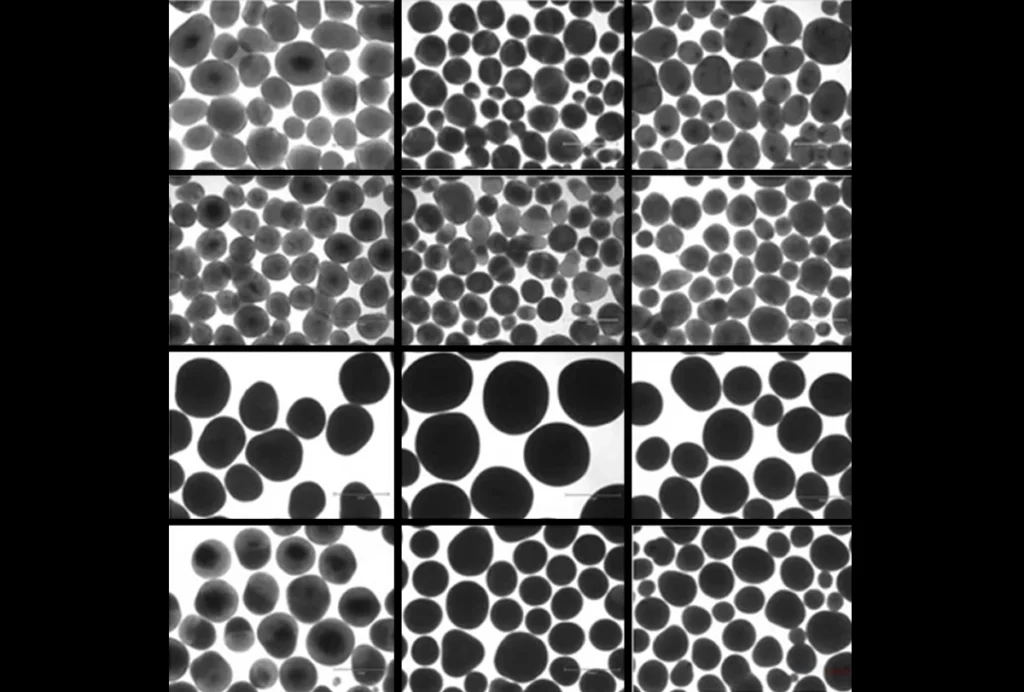
Brain organoid size matches intensity of social problems in autistic people
Explore more from The Transmitter
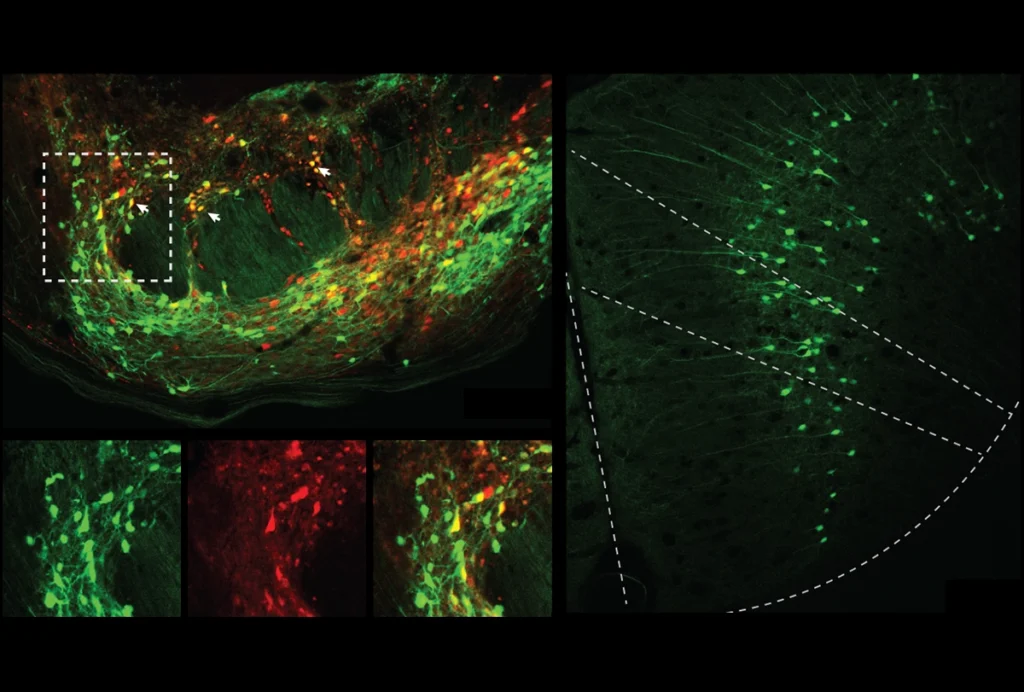
Cerebellar circuit may convert expected pain relief into real thing
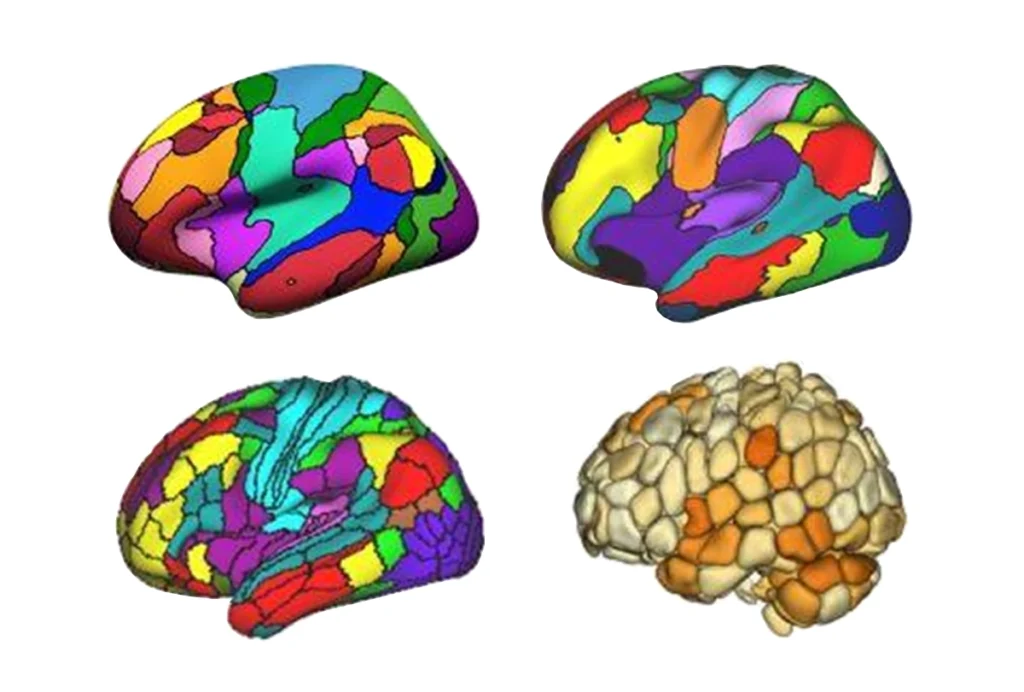