How to break new ground in the search for autism biomarkers
A large dataset that catalogs the features of many brain conditions could transform the quest for biomarkers of autism.
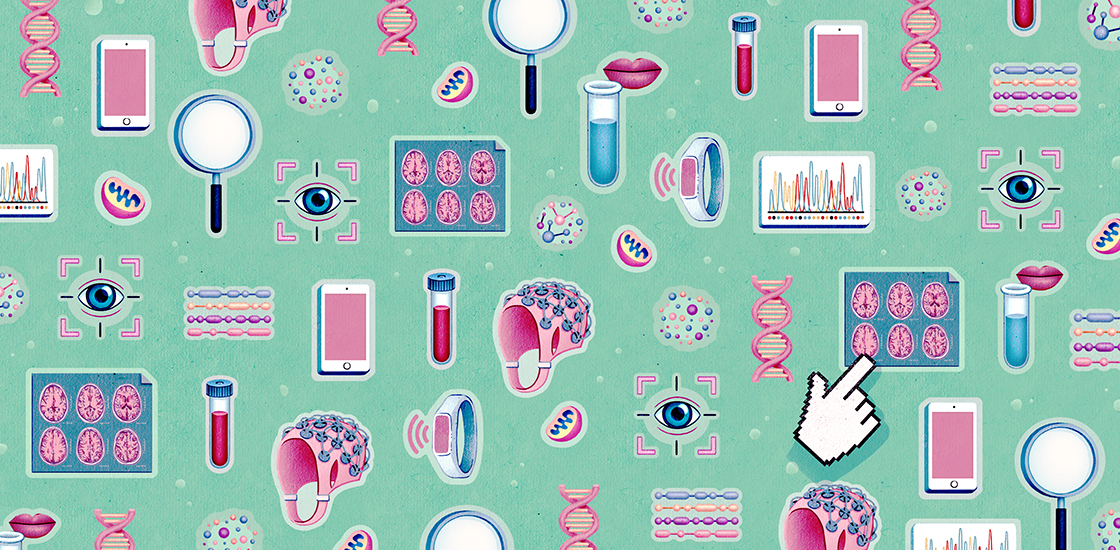
Finding biological markers for psychiatric conditions is the holy grail of mental health research. Clinicians currently rely on subjective measures to diagnose these conditions and track an individual’s response to treatment. Replacing these subjective measures with objective ones called ‘biomarkers’ would be nothing short of transformative1.
Decades of research into genes, proteins and the brain have generated promising leads for biomarkers. For instance, we now have a long list of genes implicated in autism risk. But these findings have yet to improve the way clinicians diagnose autism and monitor the condition over time.
Our current diagnostic categories do not reliably map to an individual’s underlying biology. For instance, two people with a diagnosis of autism may have different genetic risk factors. They may also differ in their features. The field has responded by looking for biologically defined subtypes of autism. For example, we know that autism in a person with mutations in the gene CDH8 tends to look different from the condition in someone with a mutation in SCN2A.
Researchers have also begun to examine features that span diagnostic boundaries. For instance, certain features of autism are also seen in people with attention deficit hyperactivity disorder and anxiety disorders. Understanding that certain features are common to multiple conditions can bolster our search for biomarkers by boosting the amount of data we have to draw upon. More and more researchers are embracing this ‘trans-diagnostic’ approach.
A new initiative from the Child Mind Institute in New York City, where I work, is taking this tack to jumpstart the search for biomarkers in autism and other brain conditions. We are creating a large trans-diagnostic dataset involving thousands of individuals who show a broad range of psychiatric features. The dataset includes a wide array of biological and behavioral data, some of which will be captured in real time over weeks by portable devices such as smartphones.
Using this approach, we think we can unearth statistically valid biomarkers more quickly than efforts confined to one diagnostic category or type of data at a time.
Breaking boundaries:
A trans-diagnostic dataset is an essential starting point. The vast majority of studies compare individuals who have a condition with the general population, not with those who have another condition that has overlapping features. And most studies are too small to distinguish subtypes within a diagnostic category.
Our institute’s Healthy Brain Network, launched in 2015, is an effort to collect a wide range of cognitive, behavioral, brain imaging and biological data from 10,000 New York residents aged 5 to 21 years2. Nearly any child or young adult who agrees to the study’s terms and can follow basic instructions is eligible. However, we are primarily recruiting children whose parents have concerns about their psychiatric symptoms.
More than 1,800 individuals have enrolled so far; about 15 percent of them have autism.
Participants come to the institute for four sessions, each lasting 3 to 3.5 hours. They receive a comprehensive diagnostic evaluation, and their families receive treatment recommendations and referral information. We assess learning and language — abilities affected in a variety of psychiatric conditions.
We are working to collect a range of biological data for each participant. The data will come in part from traditional tools, such as brain imaging, eye tracking, blood and saliva tests, and gene sequencing. They will also come from devices that participants carry with them.
Momentary measures:
Wearable devices such as Fitbits can measure a person’s sleep, heart rate, breathing, movements, physical activity, stress levels and vocalizations in real time. Wearables are tracking the physical activity and sleep of each participant over the course of a month.
Smartphones can prompt individuals to complete self-report surveys or specific tasks as they go about their day. These intermittent measurements, called ecological momentary assessments, can capture moods, behaviors and experiences over days, weeks or months. In our project, smartphones will soon be collecting cognitive, mood and voice data from participants.
Used in combination, these tools can transform the accuracy and reliability with which we measure certain features in individuals with autism.
The project is also making it possible to integrate data drawn from different techniques. For example, we are collecting both electroencephalography and functional magnetic resonance imaging data from each participant. Integrating the data from these two tools could dramatically enhance our understanding of brain function and help to identify novel biomarkers.
We expect data collection for this initiative to wrap up in 2022. However, we are making the data available to the scientific community as it arrives (with proper privacy protections in place). Already, researchers from more than 60 institutions around the world are using the 1,100 datasets created to date. They are exploring leads for biomarkers and generating their own.
Some of these researchers have already reached out to us with insights and advice. This give-and-take may prove to be as valuable to advancing the search for biomarkers as the biobank itself.
Michael Milham is vice president of research and Phyllis Green and Randolph Cowen Scholar at the Child Mind Institute in New York City.
Recommended reading
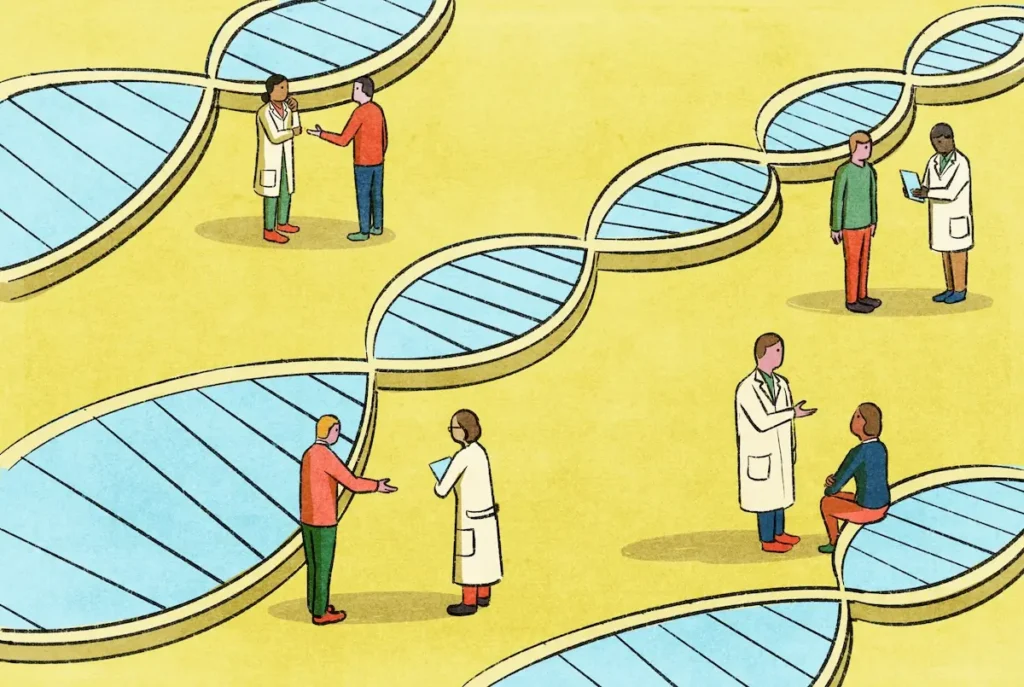
Building an autism research registry: Q&A with Tony Charman
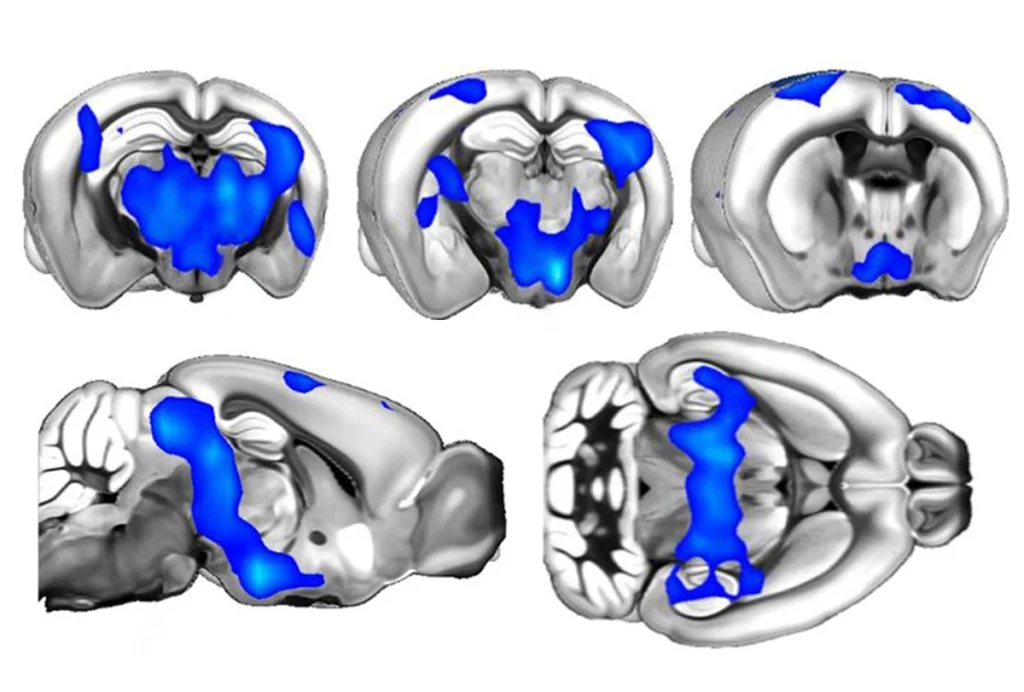
CNTNAP2 variants; trait trajectories; sensory reactivity
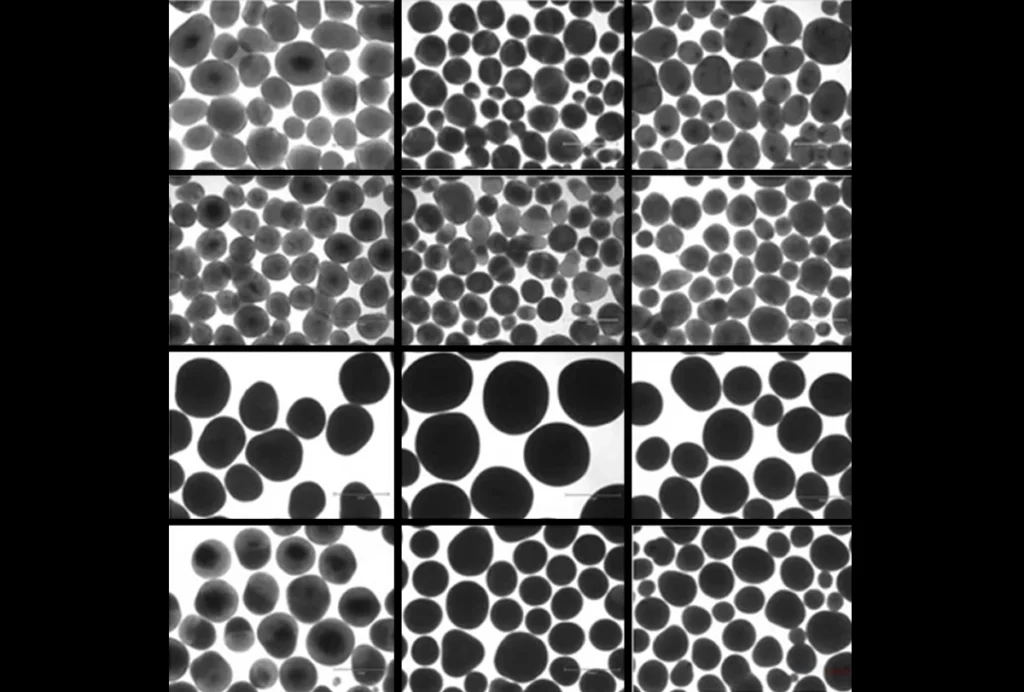
Brain organoid size matches intensity of social problems in autistic people
Explore more from The Transmitter
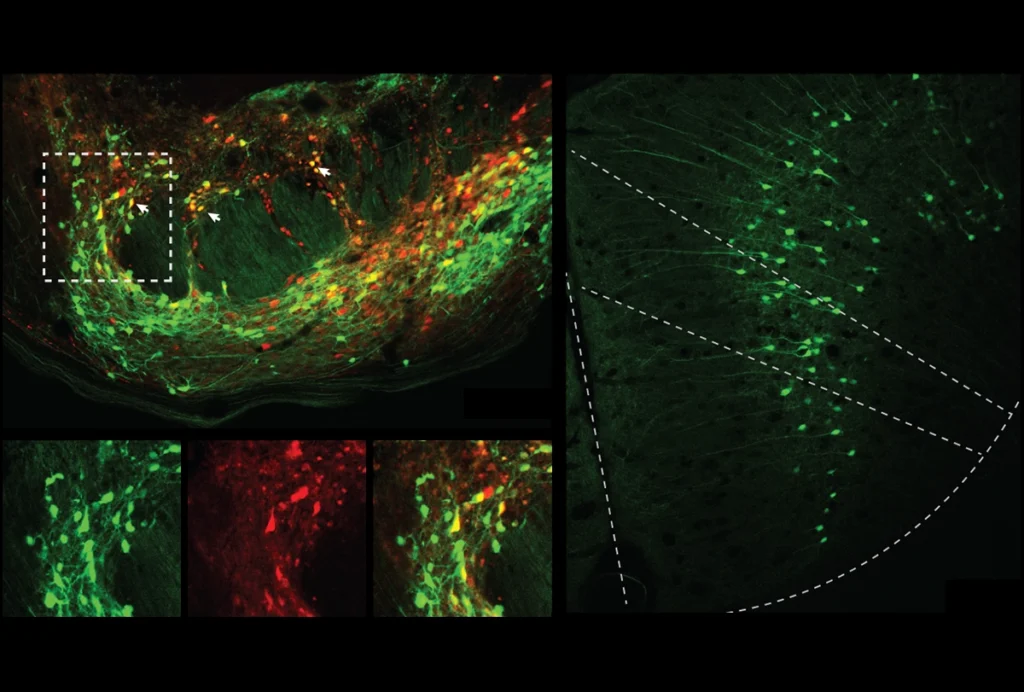
Cerebellar circuit may convert expected pain relief into real thing
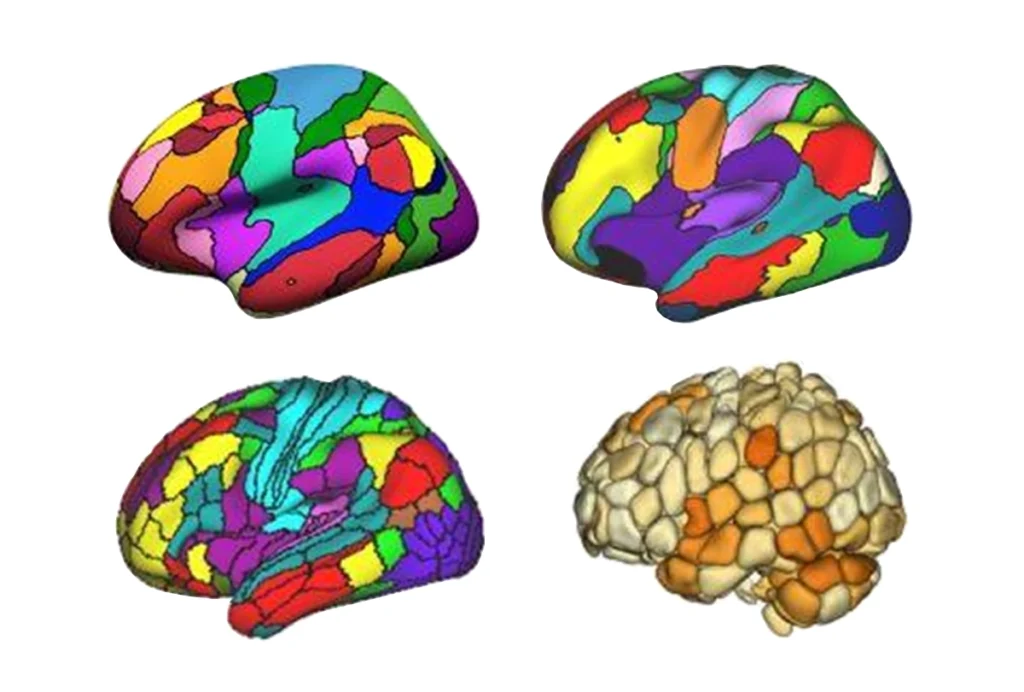