Mouse models aren’t perfect mimics of neurodevelopmental conditions, but they are often crucial for testing candidate treatments.
In the case of Angelman syndrome, a genetic condition caused by mutation or loss of the maternal copy of the UBE3A gene, model mice can replicate the condition’s hallmark lack of UBE3A protein, plus its frequent seizures and motor difficulties. And now that multiple therapies for the syndrome are in the works, assessing the behaviors of these model mice — and how they change with treatment — is particularly important, says Michael Sidorov, principal investigator at the Center for Neuroscience Research at Children’s National Hospital in Washington, D.C. “There’s a real need to be able to screen these drugs preclinically.”
His team developed a single ‘severity score’ of mouse behavior that differentiates Angelman syndrome model mice from wildtype mice. And they packaged their technique into a graphical user interface called ‘phenotyping using a multidimensional behavioral analysis algorithm’ — or PUMBAA — for anyone to use.
Sidorov spoke with Spectrum about the new tool, described in a study published this month in Translational Psychiatry, and how it could help other researchers studying neurodevelopmental conditions.
This interview has been edited for length and clarity.
Spectrum: How do researchers typically test behaviors in Angelman syndrome model mice?
Michael Sidorov: There’s a great behavioral battery developed by Ype Elgersma, who we collaborated with, that can reliably differentiate between Angelman model mice and wildtype mice. It consists of a set of five behavioral tests that, among other things, assess the animals’ motor skills, repetitive behaviors and willingness to explore an open space.
We worked with him to see if we could do a little bit better at assessing the behaviors of these mice: Does it have to be these behavioral tests? And can we summarize that behavior as a single number?
S: How did you get to that single number?
MS: We used principal component analysis, which is mathematically complicated but conceptually simple. The idea is to take a dataset that has many dimensions and reduce its dimensionality. In this case, our dimensions are the eight measures in our battery of tests — seven measures from the five behavioral tests, plus the animal’s weight. But we didn’t want to plot something in eight-dimensional space.
We discovered that we can plot the data in two-dimensional space with two components — principal component one (PC1) and principal component two (PC2) — in which those dimensions represent where the greatest variation in the data occurs. You can plot them like X and Y coordinates, and 95 percent of the time you can tell correctly whether a mouse is an Angelman mouse or a wildtype mouse just based upon that.
But the really cool thing is that you actually don’t even need the y-axis. You can distinguish between Angelman syndrome mice and wildtype mice just by using the x-axis, or PC1. And so you can represent overall behavioral severity as a single number.
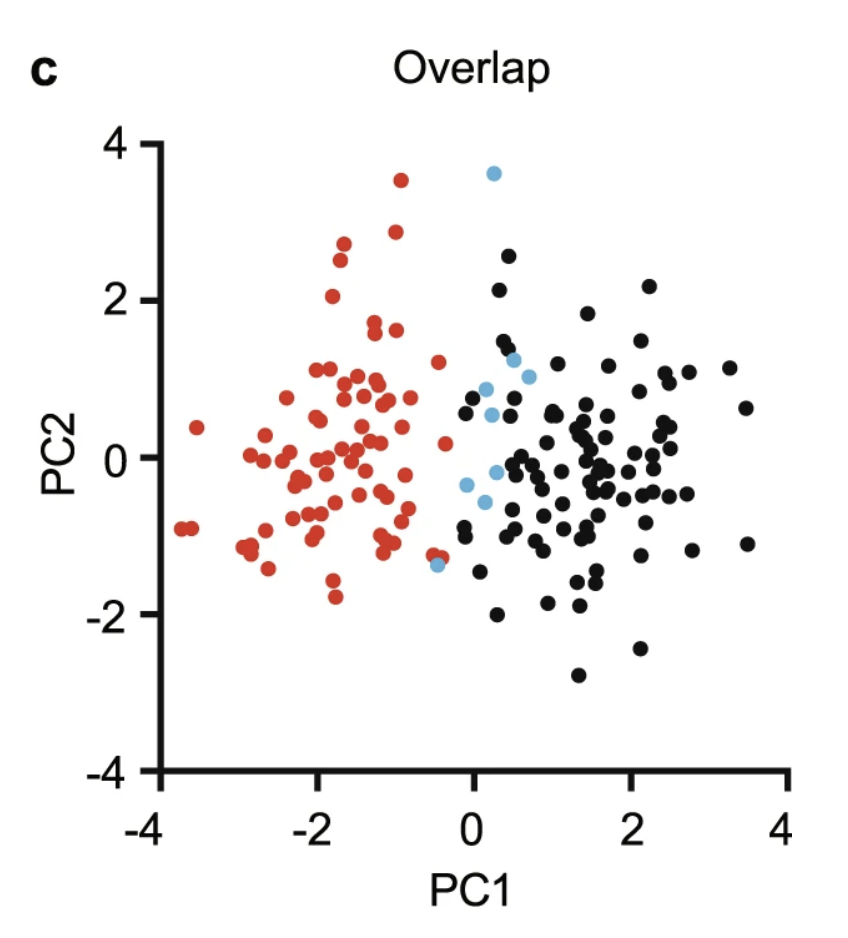
That single number represents the overall behavioral profile of a mouse, and so it can be used to ask whether, for example, a new behavior that you measure correlates with the rest of the animal’s profile. And, of course, you can also ask whether that number changes as a function of treatment, which is what we did.
S: How did you go about that?
MS: We looked at an existing dataset from Mark Zylka’s group at the University of North Carolina at Chapel Hill. They used a CRISPR-Cas9 approach to unsilence the silent paternal UBE3A allele to make up for the lack of UBE3A protein in Angelman syndrome model mice. And after doing that, they saw some behavioral improvements in the treated mice.
So we took their behavioral results and ran them through PUMBAA. And what we saw was in line with what they had previously shown: Behavior improved overall in the Angelman mice — their severity scores improved — but it wasn’t fully corrected. It’s a nice proof of concept.
S: What kind of study would benefit from PUMBAA?
MS: The thing about principal component analysis is that it’s only going to work as well as the data going into it. So if you have a model mouse that is not very different from its wildtype littermates, then PCA is not going to magically pull out some meaningful difference.
Where this tool might add some extra value is if you have slight but not super reliable behavioral phenotypes. It will probably work best in mouse models in which you already know the behaviors that are likely to be different for whatever condition you’re studying, and when you’re performing a battery of multiple tests and you want to summarize the overall results of the battery.
In that case, PUMBAA can give each mouse a ‘severity score’ that enables you to more easily compare behavior across model mice and controls, or across treated and untreated mice.
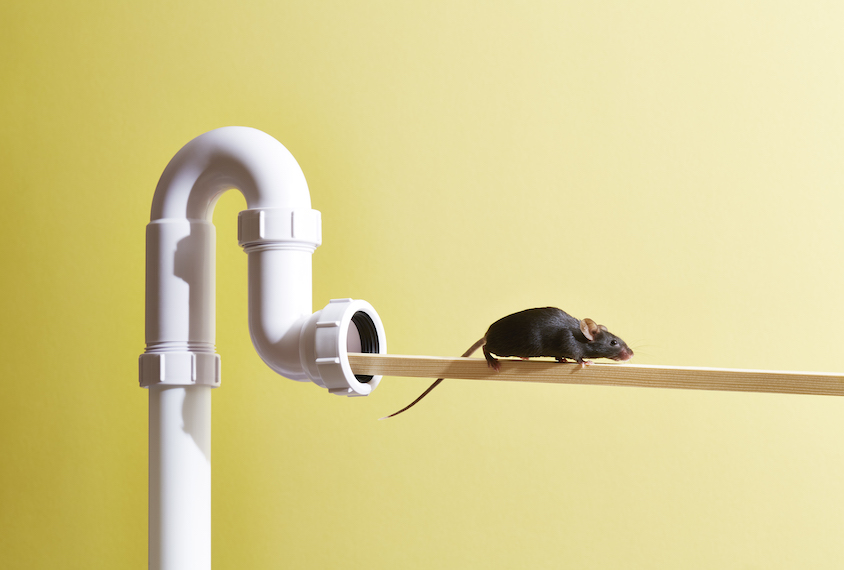
S: One thing you looked at in the paper is how effective PUMBAA is, even if you remove some of the input. Can you tell me why that’s important?
MS: That helps us to generalize. Different labs specialize in different tests. And not every lab testing a treatment, for instance, is going to have the capacity to run every potential behavioral test for a condition.
When we removed behavioral tests from the analysis one by one, it didn’t seem like any one behavior was really driving the effect. It seems like PC1 — at least for Angelman syndrome model mice — was made up of all of the behaviors that went into the analysis.
S: What do you hope other researchers get out of this?
MS: The exact application of PCA is going to be different depending on exactly what you’re trying to study. But we hope that the word gets out and that people will use it. And we’re always looking to collaborate with folks who are studying mouse models of different neurodevelopmental conditions.