Brian Lee is associate professor of epidemiology and biostatistics at Drexel University in Philadelphia.
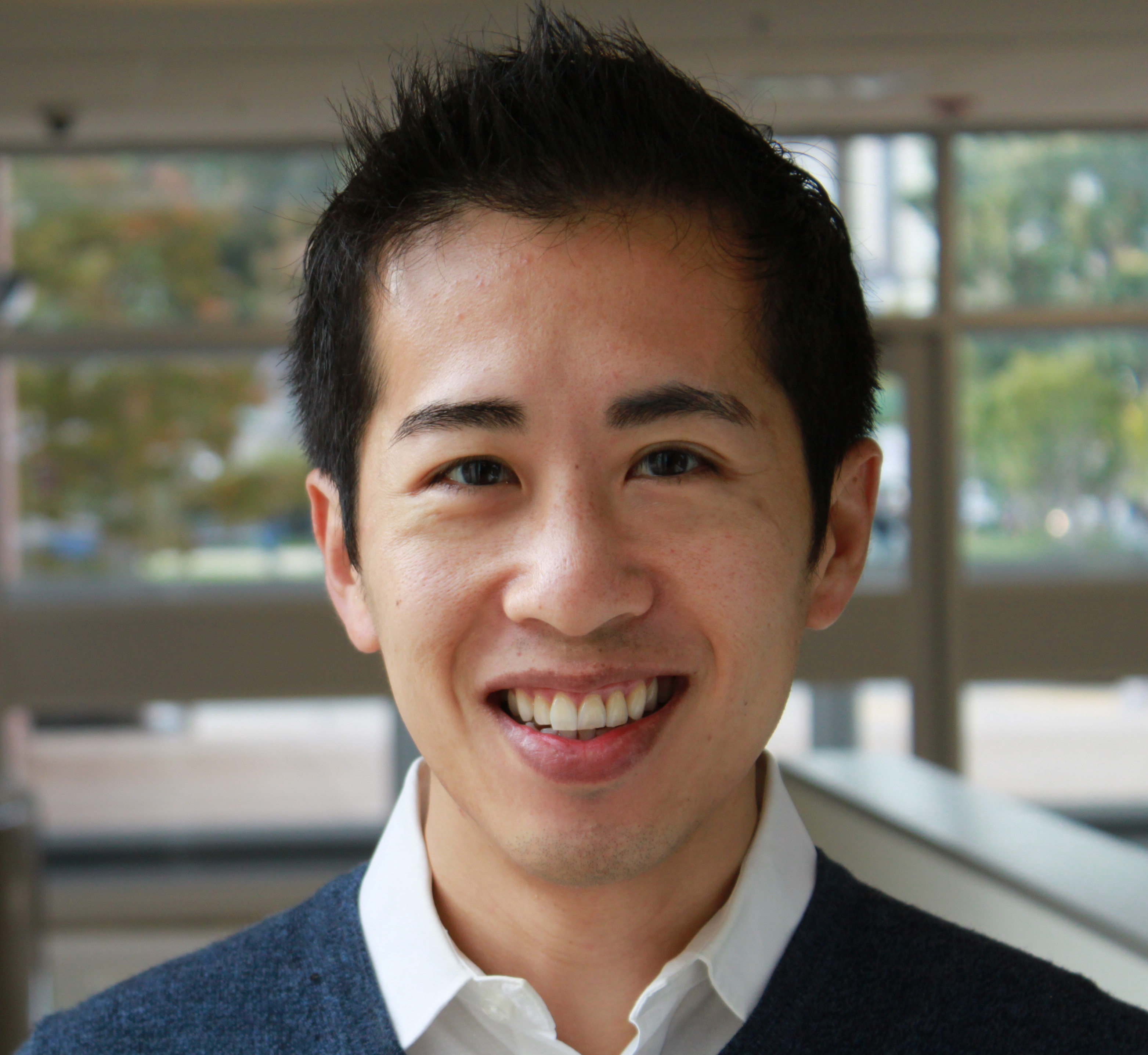
Brian Lee
Associate professor
Drexel University
From this contributor
Journal club: Does lithium in drinking water contribute to autism?
A study published in JAMA Pediatrics suggests that autism is more common among people born in areas with high levels of lithium in drinking water, but it is too soon to say whether prenatal lithium exposure is truly a concern.
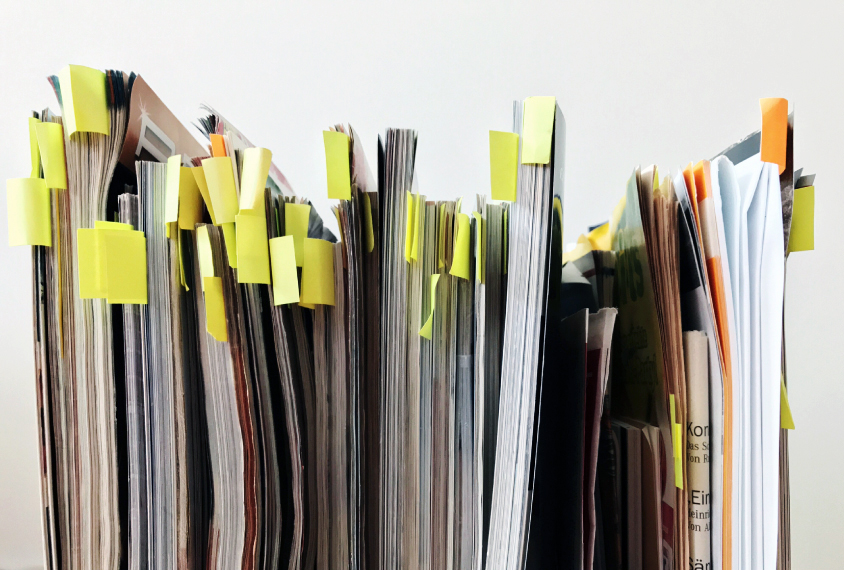
Journal club: Does lithium in drinking water contribute to autism?
Autism heritability: It probably does not mean what you think it means
The question of autism's heritability is compelling for researchers and laypeople alike, but many people in both groups misunderstand its definition.
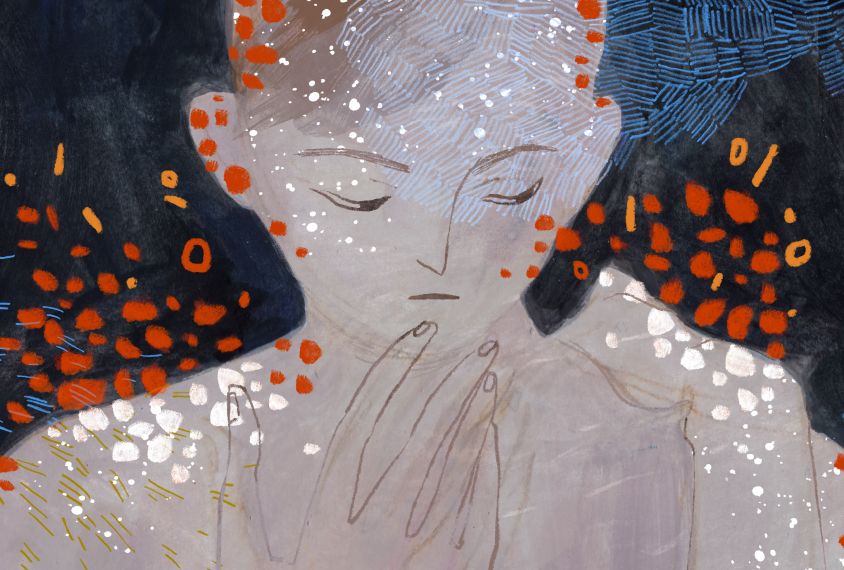
Autism heritability: It probably does not mean what you think it means
Explore more from The Transmitter
Sharing Africa’s brain data: Q&A with Amadi Ihunwo
These data are “virtually mandatory” to advance neuroscience, says Ihunwo, a co-investigator of the Brain Research International Data Governance & Exchange (BRIDGE) initiative, which seeks to develop a global framework for sharing, using and protecting neuroscience data.
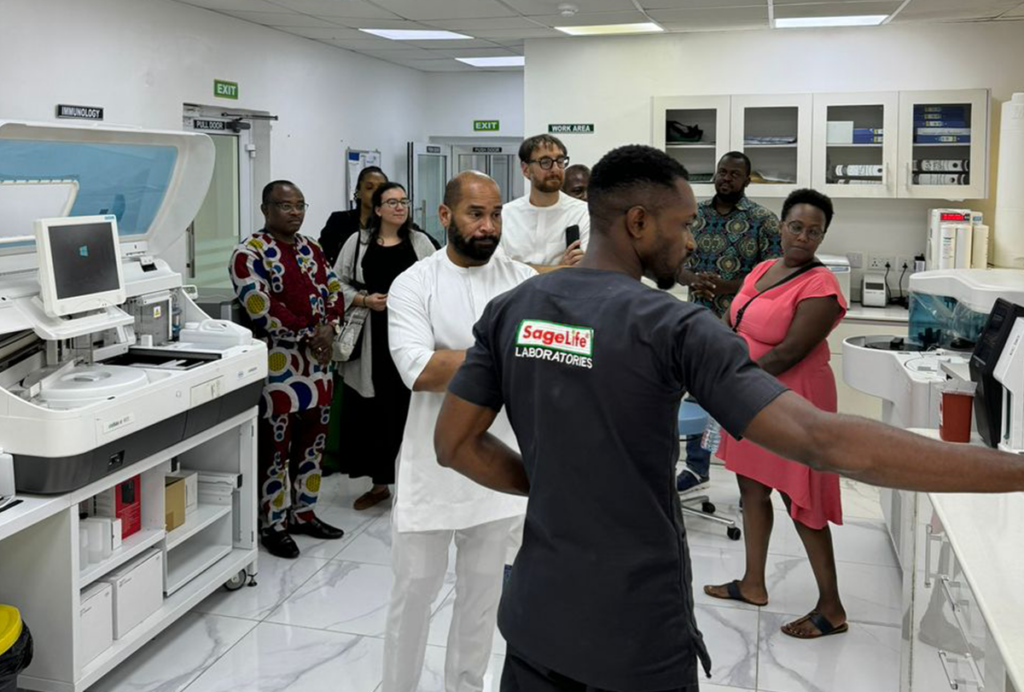
Sharing Africa’s brain data: Q&A with Amadi Ihunwo
These data are “virtually mandatory” to advance neuroscience, says Ihunwo, a co-investigator of the Brain Research International Data Governance & Exchange (BRIDGE) initiative, which seeks to develop a global framework for sharing, using and protecting neuroscience data.
Cortical structures in infants linked to future language skills; and more
Here is a roundup of autism-related news and research spotted around the web for the week of 19 May.
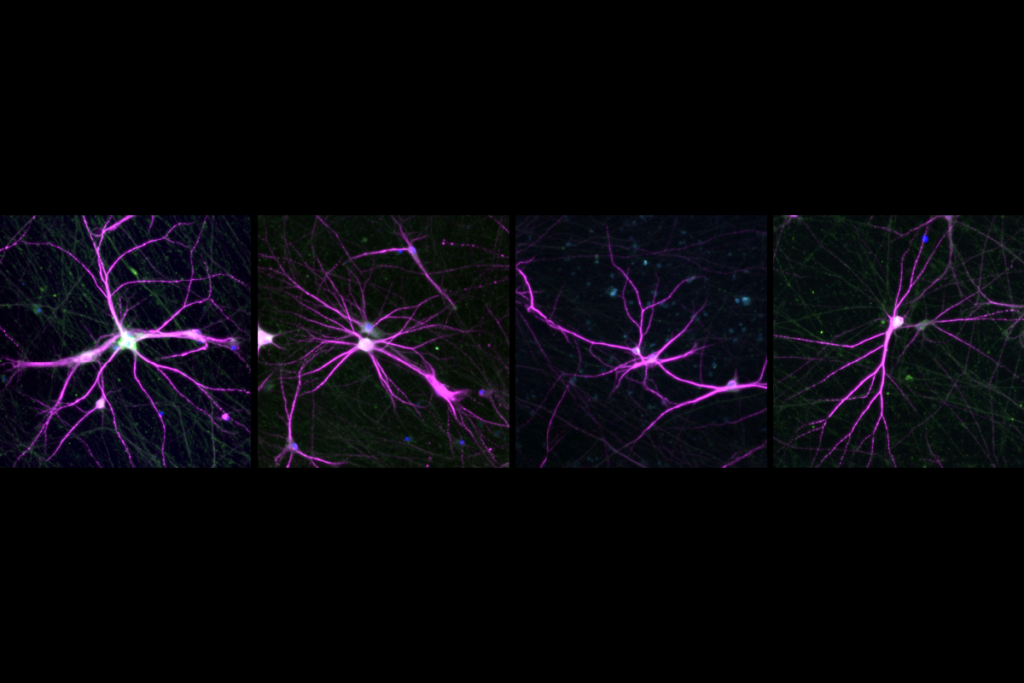
Cortical structures in infants linked to future language skills; and more
Here is a roundup of autism-related news and research spotted around the web for the week of 19 May.
The BabyLM Challenge: In search of more efficient learning algorithms, researchers look to infants
A competition that trains language models on relatively small datasets of words, closer in size to what a child hears up to age 13, seeks solutions to some of the major challenges of today’s large language models.
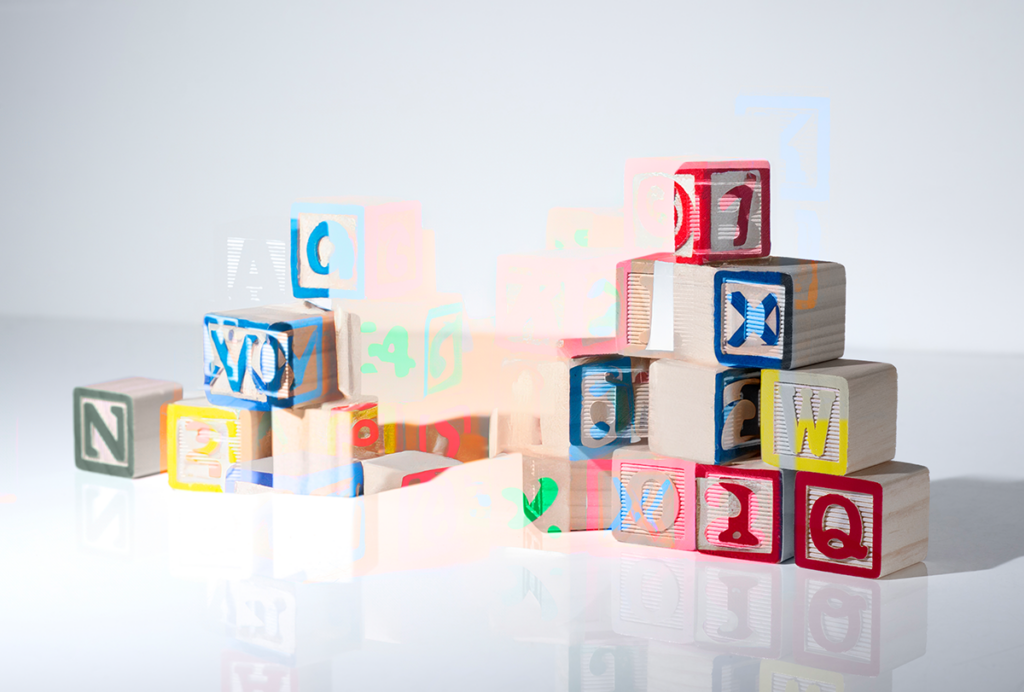
The BabyLM Challenge: In search of more efficient learning algorithms, researchers look to infants
A competition that trains language models on relatively small datasets of words, closer in size to what a child hears up to age 13, seeks solutions to some of the major challenges of today’s large language models.