Evan Schaffer is assistant professor of neuroscience at the Friedman Brain Institute at the Icahn School of Medicine at Mount Sinai. His lab uses mathematical tools to understand distributed computations in the brain, identify how these computations change with learning and identify how feedback from the body impacts cognition. Schaffer received his Ph.D. at the Center for Theoretical Neuroscience, in Larry Abbott’s lab at Columbia University. He completed his postdoctoral work in Richard Axel’s Lab at Columbia University
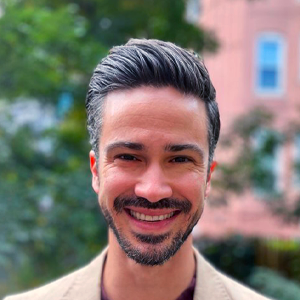
Evan Schaffer
Assistant professor of neuroscience
Icahn School of Medicine at Mount Sinai
Selected articles
- “Inhibitory stabilization of the cortical network underlies visual surround suppression” | Neuron
- “A complex-valued firing-rate model that approximates the dynamics of spiking networks” | PLoS Computational Biology
- “Odor perception on the two sides of the brain: Consistency despite randomness” | Neuron
- “The spatial and temporal structure of neural activity across the fly brain” | Nature Communications
- “Behavioral fingerprinting of the naked mole-rat uncovers signatures of eusociality and social touch” | bioRxiv
Explore more from The Transmitter
More than two dozen papers by neural tube researcher come under scrutiny
One of the studies, published in 2021 in Science Advances, received an editorial expression of concern on 21 May, after the journal learned that an institutional review of alleged image problems is underway.
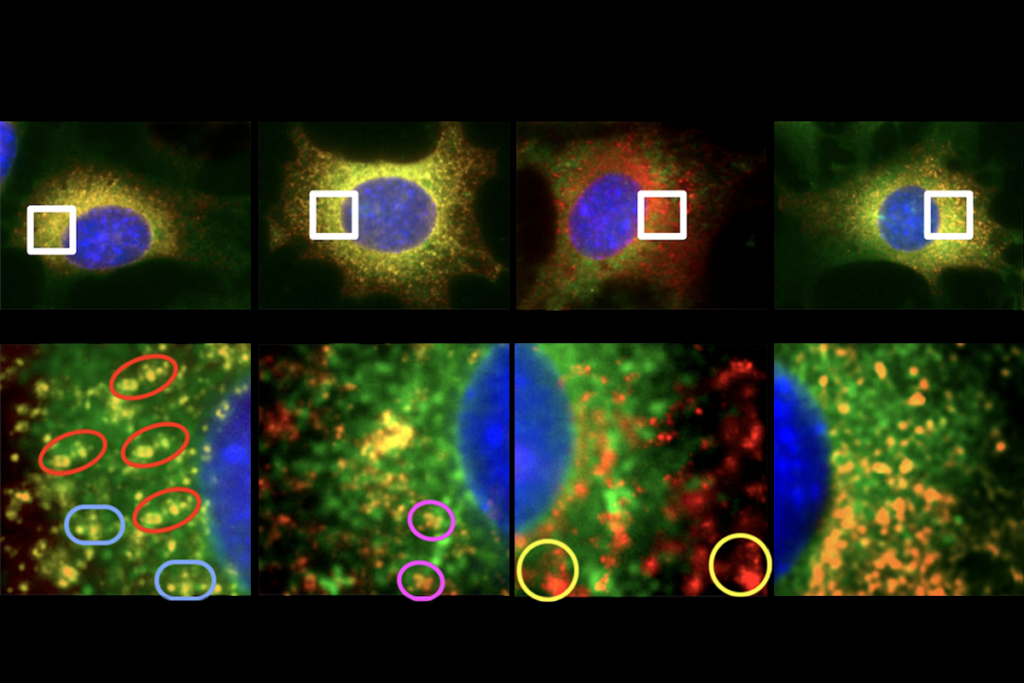
More than two dozen papers by neural tube researcher come under scrutiny
One of the studies, published in 2021 in Science Advances, received an editorial expression of concern on 21 May, after the journal learned that an institutional review of alleged image problems is underway.
On the importance of reading (just not too much)
The real fun of being a neuroscientist, and maybe the key to asking and answering new questions, is to think big and take intellectual risks.
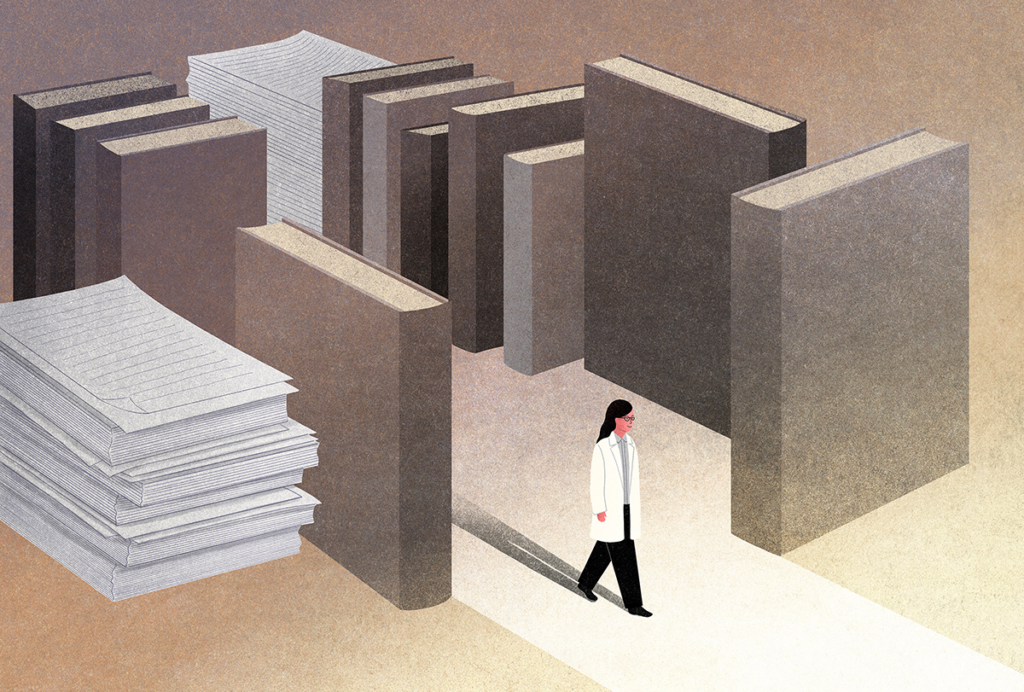
On the importance of reading (just not too much)
The real fun of being a neuroscientist, and maybe the key to asking and answering new questions, is to think big and take intellectual risks.
How developing neurons simplify their search for a synaptic mate
Streamlining the problem from 3D to 1D eases the expedition—a strategy the study investigators deployed to rewire an olfactory circuit in flies.
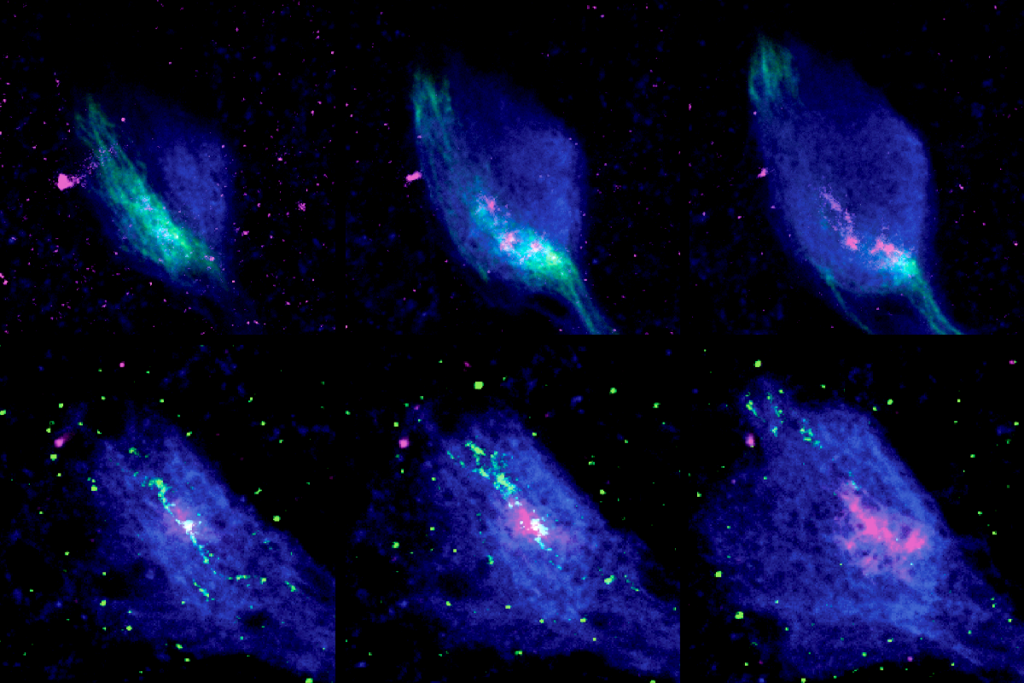
How developing neurons simplify their search for a synaptic mate
Streamlining the problem from 3D to 1D eases the expedition—a strategy the study investigators deployed to rewire an olfactory circuit in flies.