Dr. Nigg’s research focuses on the underlying mechanisms and causes in attention deficit hyperactivity disorder (ADHD) across the lifespan. The primary focus is on cognitive and neuropsychological mechanisms. However, studies of genetics, family dynamics, personality and temperament, environmental toxins, and neuroimaging of ADHD are also ongoing.
Joel Nigg
Director, Division of Psychology
Oregon Health and Science University
From this contributor
Insights for autism from attention deficit hyperactivity disorder
Autism and attention deficit hyperactivity disorder show genetic and neurobiological overlap, which may provide clues to the origin of both disorders, says Joel Nigg.
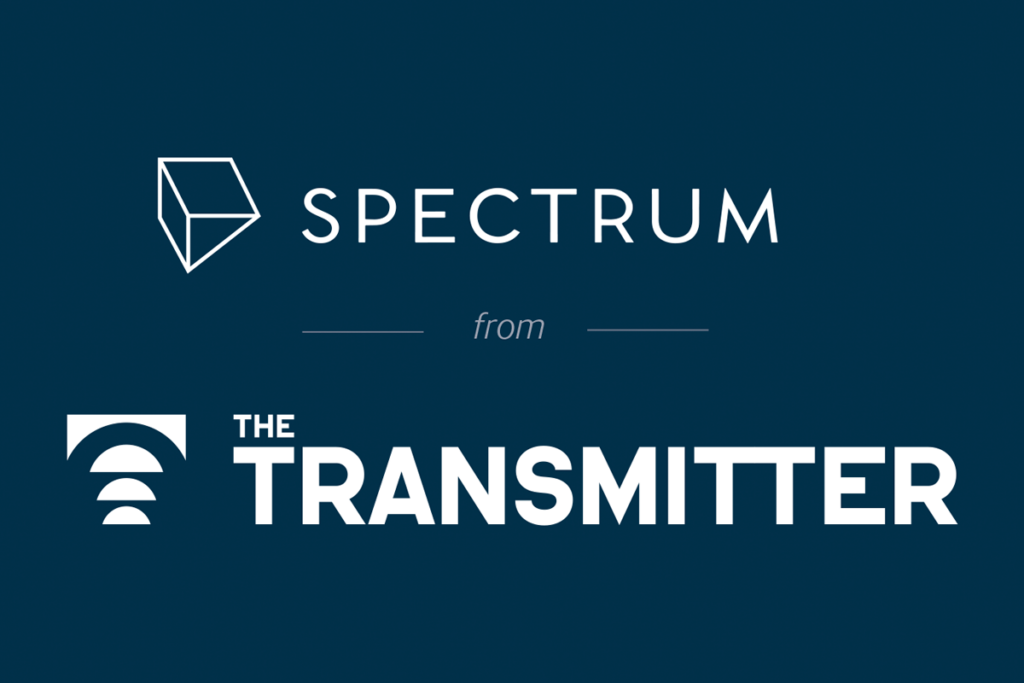
Insights for autism from attention deficit hyperactivity disorder
Explore more from The Transmitter
Sharing Africa’s brain data: Q&A with Amadi Ihunwo
These data are “virtually mandatory” to advance neuroscience, says Ihunwo, a co-investigator of the Brain Research International Data Governance & Exchange (BRIDGE) initiative, which seeks to develop a global framework for sharing, using and protecting neuroscience data.
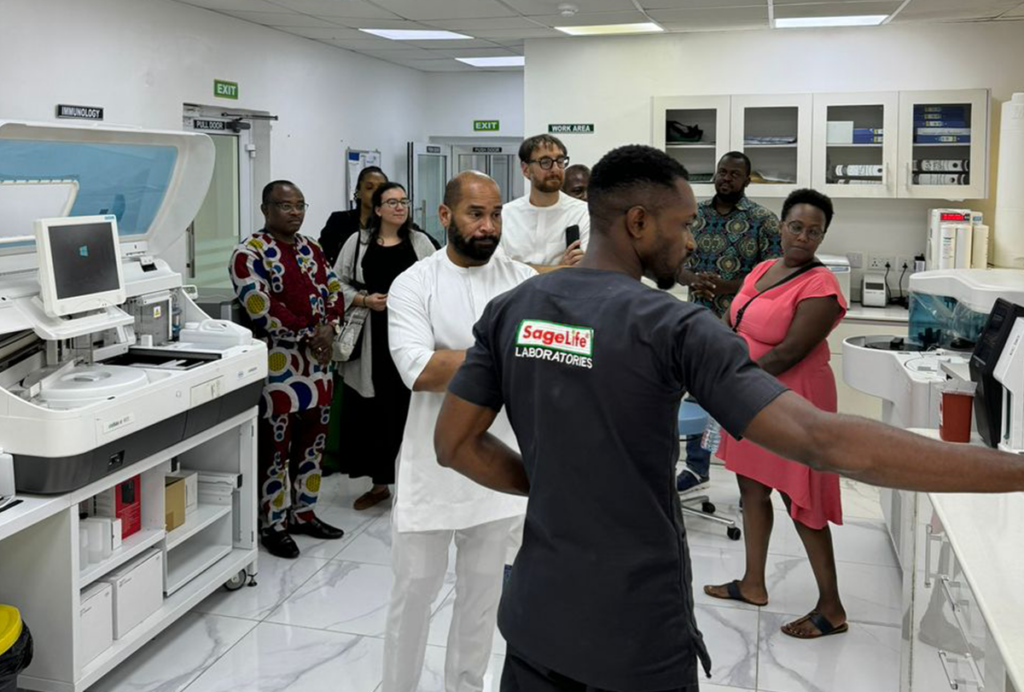
Sharing Africa’s brain data: Q&A with Amadi Ihunwo
These data are “virtually mandatory” to advance neuroscience, says Ihunwo, a co-investigator of the Brain Research International Data Governance & Exchange (BRIDGE) initiative, which seeks to develop a global framework for sharing, using and protecting neuroscience data.
Cortical structures in infants linked to future language skills; and more
Here is a roundup of autism-related news and research spotted around the web for the week of 19 May.
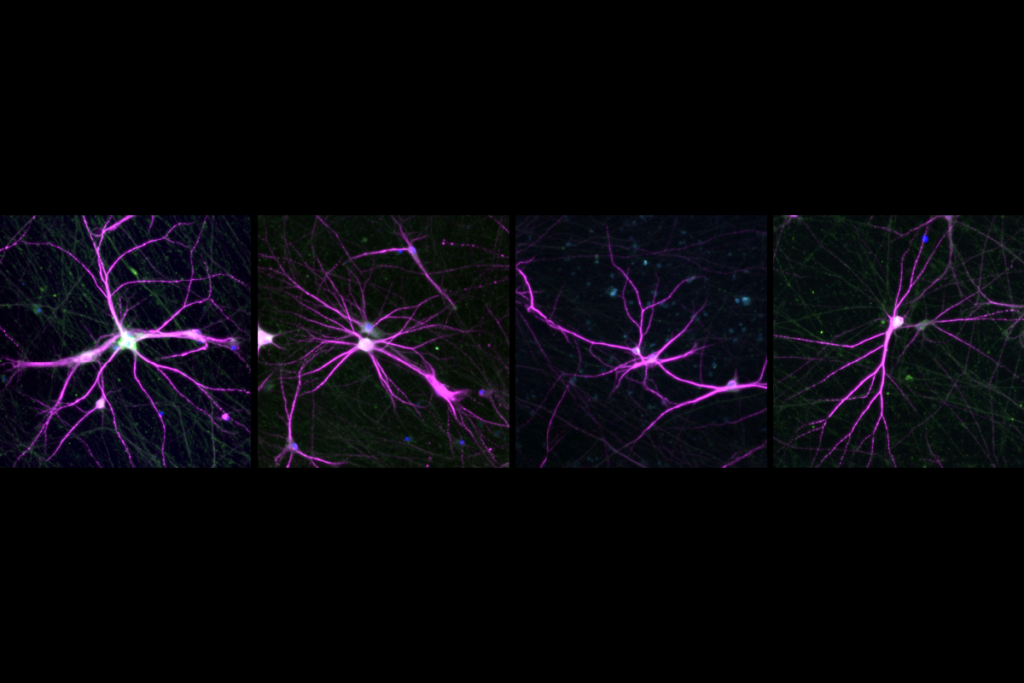
Cortical structures in infants linked to future language skills; and more
Here is a roundup of autism-related news and research spotted around the web for the week of 19 May.
The BabyLM Challenge: In search of more efficient learning algorithms, researchers look to infants
A competition that trains language models on relatively small datasets of words, closer in size to what a child hears up to age 13, seeks solutions to some of the major challenges of today’s large language models.
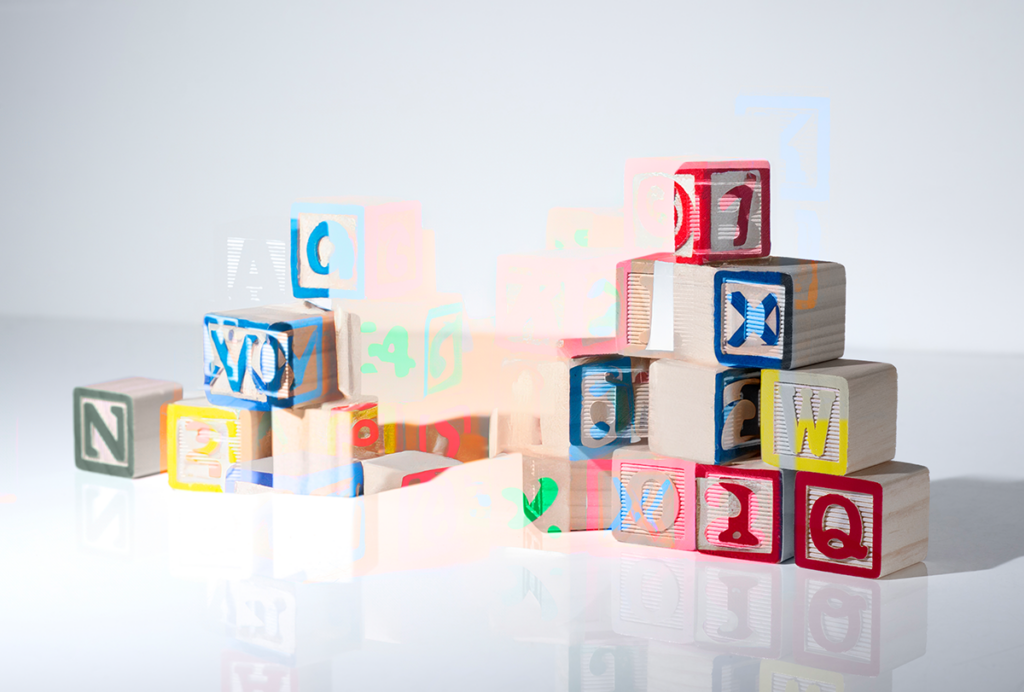
The BabyLM Challenge: In search of more efficient learning algorithms, researchers look to infants
A competition that trains language models on relatively small datasets of words, closer in size to what a child hears up to age 13, seeks solutions to some of the major challenges of today’s large language models.