Mayada Elsabbagh is associate professor of neurology and neurosurgery at the Montreal Neurological Institute at McGill University in Canada. Her research focuses on understanding the root causes of autism and tracing its developmental pathways. The approach combines innovative research with the mission of accelerating the translation of scientific discoveries into community impact. Elsabbagh’s contributions include the discovery of early brain-function markers for autism prior to the onset of behavioral signs. She has supported the successful launch of several collaborative research and translational networks aimed at accelerating the pace of discovery in autism. This includes the Transforming Autism Care Consortium, a Québec research network supported by the Fonds de recherche du Québec-Santé and several community partners. She is also active in global efforts to improve evidence-based practice in the community and capacity-building in low- and middle-income countries. The public value and social relevance of Elsabbagh’s research has been recognized through various awards, including the Neville Butler Memorial Prize and the British Psychological Society Neil O’Conner Prize.
Mayada Elsabbagh
Assistant Professor
McGill University
From this contributor
Autism therapies blur boundary between clinic, everyday life
Modern treatments for autism are often led by parents and integrated into a child’s daily life.
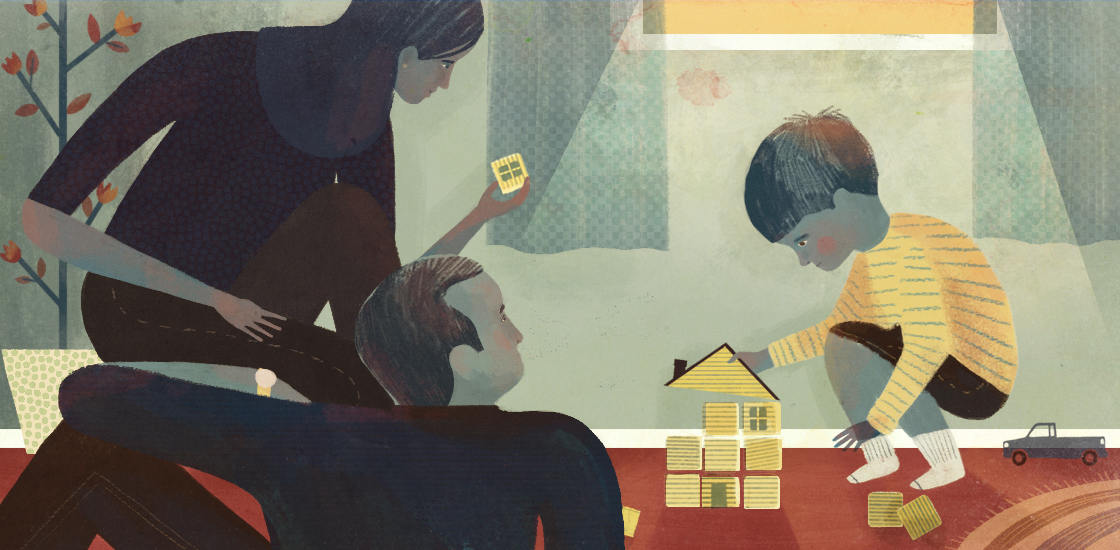
Autism therapies blur boundary between clinic, everyday life
To screen or not to screen: That is not the question
Instead of debating about whether to screen all children for autism, we should be looking for better ways to identify children at risk and help them access services.
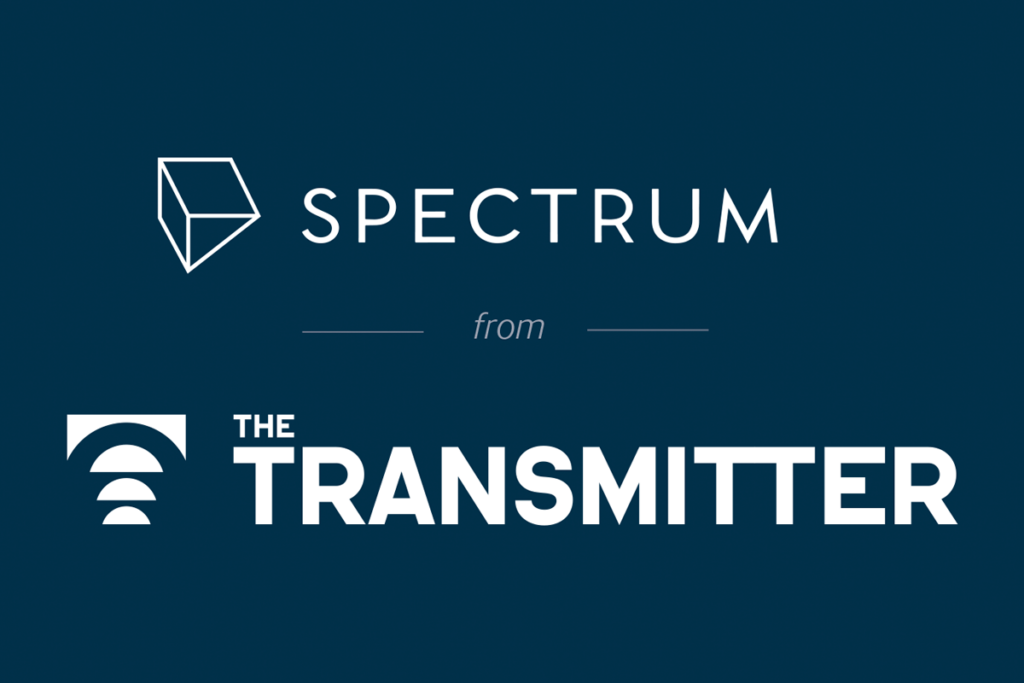
To screen or not to screen: That is not the question
Why inferring autism’s causes from epidemiology is dangerous
Epidemiological 'just-so' stories, which infer causes of autism from general trends in prevalence, are in danger of repeating the mistakes of social Darwinism, says Mayada Elsabbagh.
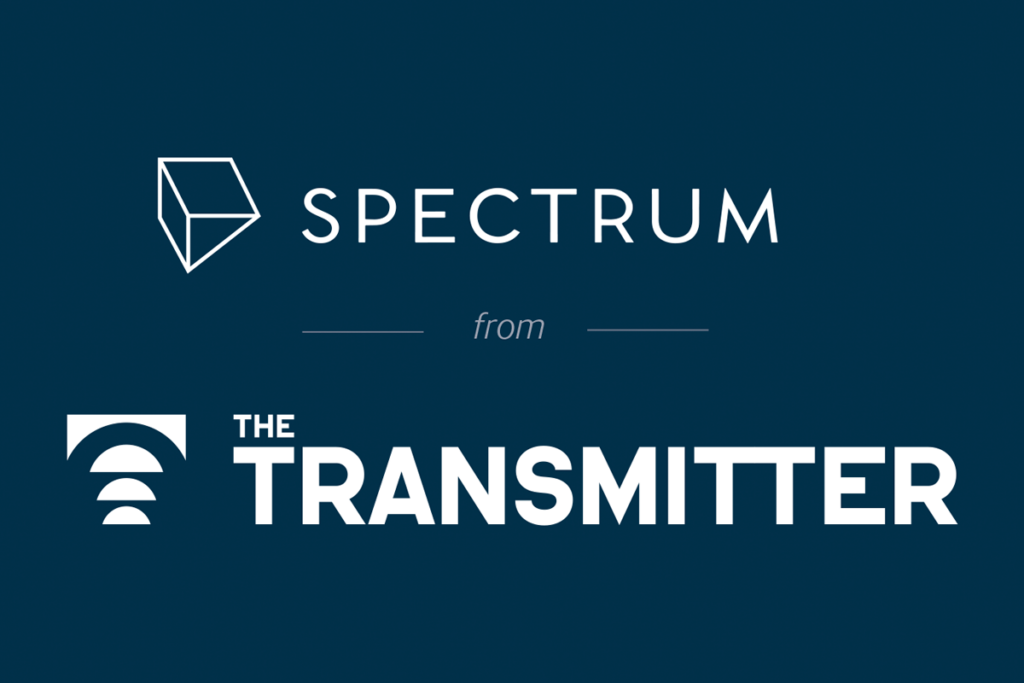
Why inferring autism’s causes from epidemiology is dangerous
A global vision for autism with community solutions
A global approach to understanding autism that respects the uniqueness of different communities is not a choice, but a necessity, says Mayada Elsabbagh.
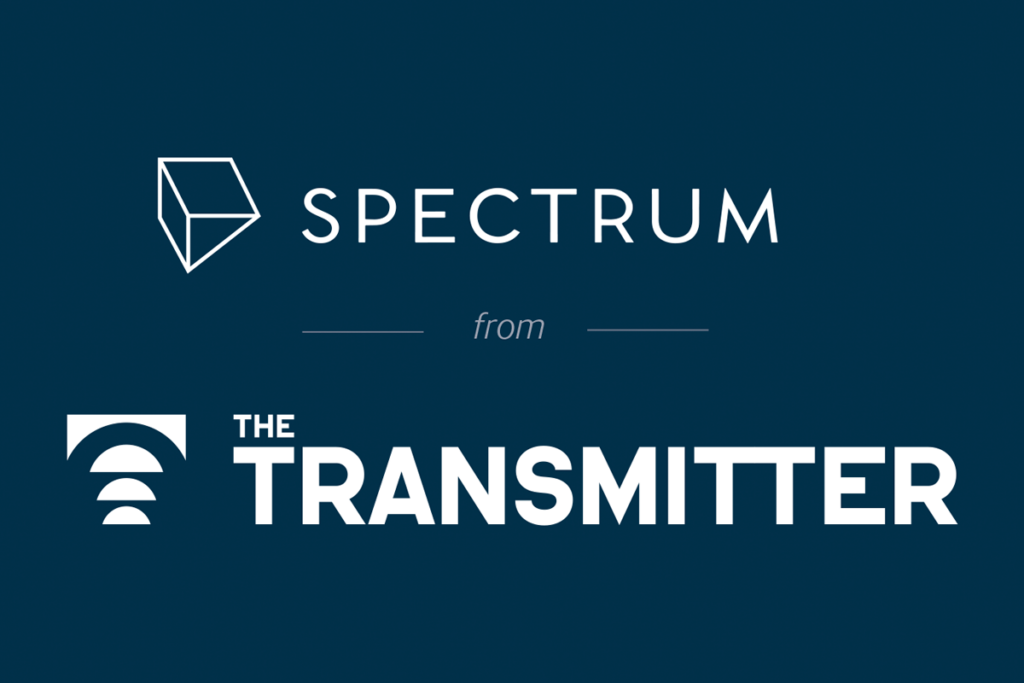
A global vision for autism with community solutions
Explore more from The Transmitter
Sharing Africa’s brain data: Q&A with Amadi Ihunwo
These data are “virtually mandatory” to advance neuroscience, says Ihunwo, a co-investigator of the Brain Research International Data Governance & Exchange (BRIDGE) initiative, which seeks to develop a global framework for sharing, using and protecting neuroscience data.
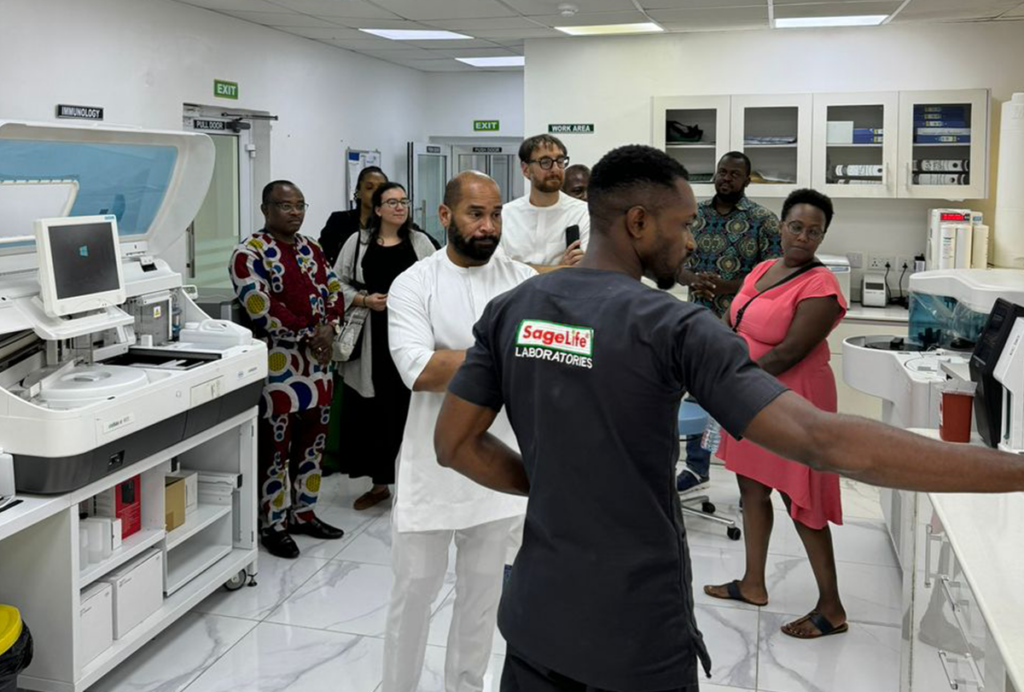
Sharing Africa’s brain data: Q&A with Amadi Ihunwo
These data are “virtually mandatory” to advance neuroscience, says Ihunwo, a co-investigator of the Brain Research International Data Governance & Exchange (BRIDGE) initiative, which seeks to develop a global framework for sharing, using and protecting neuroscience data.
Cortical structures in infants linked to future language skills; and more
Here is a roundup of autism-related news and research spotted around the web for the week of 19 May.
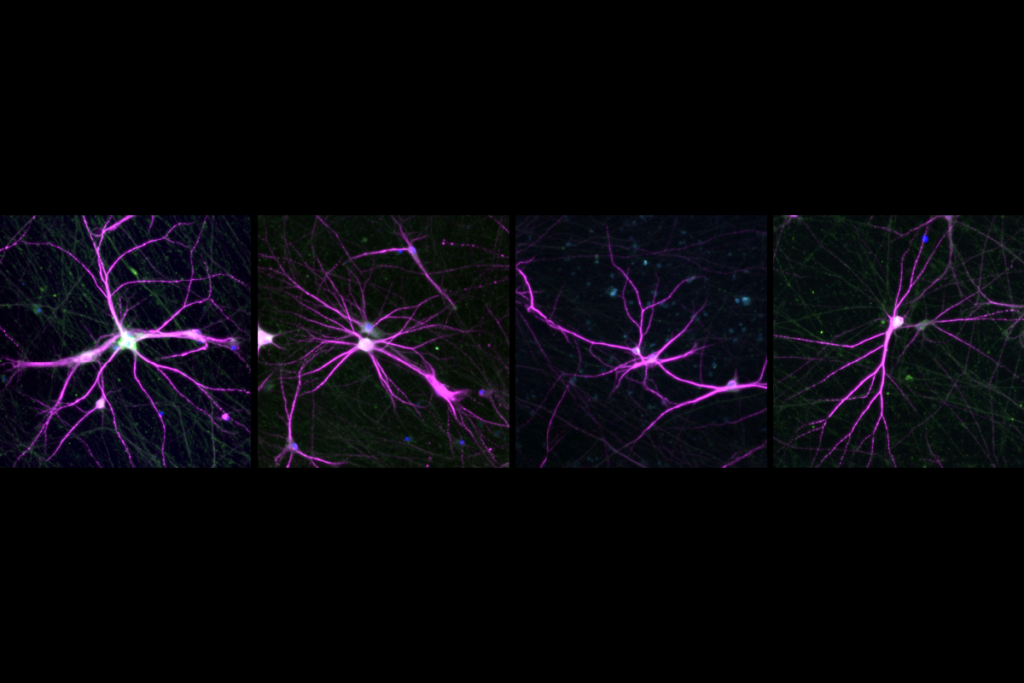
Cortical structures in infants linked to future language skills; and more
Here is a roundup of autism-related news and research spotted around the web for the week of 19 May.
The BabyLM Challenge: In search of more efficient learning algorithms, researchers look to infants
A competition that trains language models on relatively small datasets of words, closer in size to what a child hears up to age 13, seeks solutions to some of the major challenges of today’s large language models.
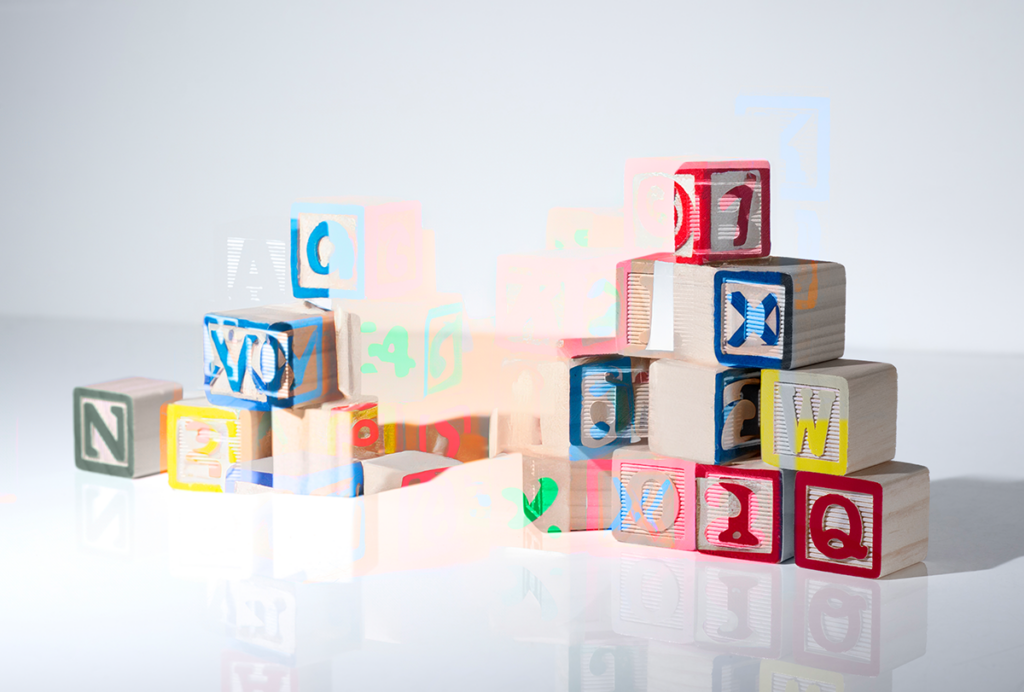
The BabyLM Challenge: In search of more efficient learning algorithms, researchers look to infants
A competition that trains language models on relatively small datasets of words, closer in size to what a child hears up to age 13, seeks solutions to some of the major challenges of today’s large language models.