At the credit crossroads: Modern neuroscience needs a cultural shift to adopt new authorship practices
Old heuristics to acknowledge contributors—calling out first and last authors, with everyone else in between—don’t work well for large collaborative and interdisciplinary projects, yet they remain the default.
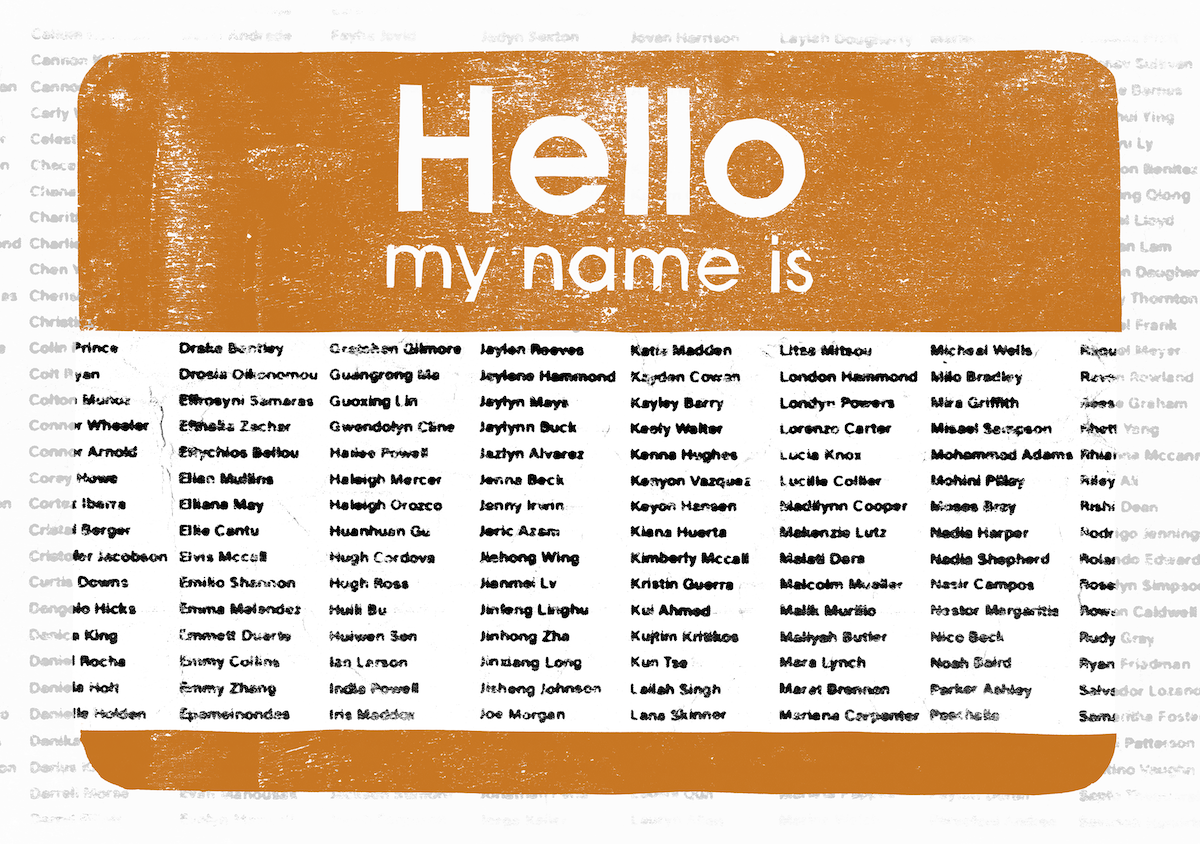
When it comes to how neuroscientists assign credit in academic writing, the field is at a crossroads. Neuroscience has roots in biology and psychology, which have traditionally favored smaller collaborations, and it relies on simple heuristics, such as authorship order, to assign credit: The first author did all the work, the last author supervised, and a few folks in between played various (smaller) supporting roles. But as neuroscience broadens to embrace cognitive and computational neuroscience, artificial intelligence, big data and more, the field is venturing into a Wild West of large consortium science.
With larger, collaborative and increasingly interdisciplinary efforts, the question of who really gets credit for a given scientific output becomes much more complex—and established cultural norms no longer work. Research contributions in neuroscience and psychology are more numerous, more varied and more specialized than ever, and the increasing adoption of open-science practices calls for even more nuanced credit assignment. How can we push forward into this brave new “big science” world while properly recognizing these contributions, both practically and socially?
Researchers have developed several potential solutions to this problem, including new ways to assign both the overall amount of credit and the type of credit each contributor should receive. I’ve suggested a few ideas below. But so far, these clever guidelines have failed to replace the first-author, last-author convention. Why? Personally, I think a true solution will require more than developing ontologically satisfying systems for credit assignment and integrating them into our publishing systems. Instead, we must find better ways to integrate these systems with established cultural norms and—importantly—our very human need for simple, interpretable heuristics.
T
o see why, let’s lay out the scope of the problem.First, what is the appropriate amount of credit to assign to a contributor? Most scientists recognize that assigning a monolithic amount of credit based on the first-author, last-author convention often fails to reflect the volume or criticality of work done by others on the list. We’ve tried a few fixes in neuroscience, including the increasing prevalence of co-first and co-last author arrangements. But I think we all know in our hearts that this doesn’t address the core challenge: People still fight over who is really first on co-first-author lists. (Some citation software still lists “Smith et al., 2005” in American Psychological Association [APA]-style in-text citations, even if the full citation is Smith*, Jones*, Lee, & Lau, with the asterisks denoting equal contribution.)
And how do we get the credits right for large, multi-lab collaborations, in which there are several trainees sharing the work equally and several equal co-principal investigators?
We could take inspiration from other fields, including particle physics and public health, that have applied a remedy at one extreme end of a spectrum: treating an “author” as essentially everybody in a consortium who ever touched some large piece of equipment or who contributed to a particular dataset. As a result, some papers have hundreds or even thousands of authors (see, for example the Large Hadron Collider paper with 5,154 authors, or the COVID-19 vaccination paper with 15,025 co-authors), or circumvent first-authorship stardom by putting the name of the consortium as first in the author list.
Some neuroscience consortia have adopted similar approaches, such as the International Brain Laboratory, which offers complex, community-developed rules for authorship. But some major concerns prevent the blanket adoption of these practices, especially in groups of tens rather than hundreds or thousands of authors. Chiefly, large-list approaches can backfire in some fields, research shows: Rather than spreading the wealth as intended, they may obscure credit, meaning no one gets the recognition they deserve.
In 2022, for example, Clarivate, a large player in data analytics that ranks “highly cited researchers,” began excluding papers with more than 30 authors from its calculation. Because the median number of authors per paper varies highly from field to field, this issue disproportionately affects fields with larger author lists, including neuroscience: As of 2018, roughly 80 percent of papers in psychiatry and psychology—and 91 percent and 74 percent, respectively, in computer science and physics—had one to five authors, compared with about 50 percent of papers in biology and neuroscience. Because modern neuroscience is heavily influenced by fields with fewer authors, we might find it particularly difficult to grapple with longer author lists.
Some have proposed new indices to augment existing practices by indicating “how much” a given author contributed, but cultural uptake of such metrics in our field has unfortunately been slow to nonexistent. A second, perhaps more challenging problem is how to recognize different kinds of contribution—collecting data, performing analyses, conceptualizing a project and acquiring funding—that are qualitatively different from one another.
One solution is the CRediT (Contributor Roles Taxonomy) system, introduced in 2015 as an attempt to provide transparency and accountability in authorship type attribution. This is the system that asks, when you submit to a journal, to tick up to 14 boxes saying who did what: conceptualization, methodology, software, validation, funding acquisition, writing and so on, with the results typically displayed in an “Author contributions” section of the manuscript. Championed as a way to diminish the use of authorship order in assigning credit, the CRediT system has been adopted by more than 40 publishers and journal families, including Public Library of Science (PLOS), Cell Press and Elsevier, and was recently adopted as an American National Standards Institute/National Information Standards Organization standard.
Unfortunately, though, I don’t think CRediT works as well in practice as we would hope. First, CRediT’s limited scope and narrow focus on predetermined traditional authorship roles may mischaracterize the diverse and ever-evolving range of contributions in larger, consortium style research—especially as we move to more complex (and laborious) data annotation and sharing. (For more, see Maryann Martone’s piece from The Transmitter’s “Open neuroscience and data-sharing” essay series.) The restriction of applying a CRediT-type system only to authors also obscures other important contributions that may not surpass a journal’s threshold for authorship, such as from technical staff. The one-size-fits-all categories also don’t always work well for opinion, educational materials, or perspective style pieces—the kinds of pieces we have typically written in large, multi-author groups at Neuromatch, for example.
W
hat about alternatives? Many smart, driven meta-scientists have developed promising innovations beyond CRediT: the expanded Contributor Role Ontology; authorship matrices; storyboarding approaches; and the Contributor Attribution Model, “an ontology-based specification for representing information about contributions made to research-related artifacts.” But uptake of these tools—and a corresponding shift in how we collectively think about and use credit assignment in practice—has proven even more achingly slow than the meaningful adoption of CRediT in daily life. This is also especially important for trainees, whose careers might be most negatively impacted by attempts to adopt idealistic but culturally challenging quick fixes, such as multiple co-first authors or “Consortium et al.” approaches.Here’s a perhaps less obvious but, in my opinion, crucial problem: As the most widely adopted system, CRediT formats author contributions to be machine readable and accessible via API, in addition to being included as “Author contributions” statements on individual papers. This formatting makes CRediT-based contributions useful for meta-analyses, for example, but less useful in aggregate for any end-user scientist like you or me. Sure, we can read all those author contribution statements on every PDF, I suppose. But it is difficult—whether we are trying to evaluate colleagues or applicants in hiring or promotion decisions or simply curious—to use CRediT-based data to figure out what kinds of contributions a particular person has made across their entire career, or even just recently.
ORCID profiles can technically integrate CRediT information from ORCID-linked papers, but they don’t summarize this information in a useful way. (Few people check someone’s ORCID profile page anyway, and even the account owner doesn’t have easy access to their own aggregate metrics.) So rather than relying on these contributions, it’s simply much easier for us all to continue characterizing our fellow scientists by counting their first-authorships and high-profile outlets on a CV or looking at their h-index on Google Scholar, even though we all know better.
To put it plainly, the challenge we’re facing in credit assignment isn’t just ontological—it’s deeply cultural, psychological and practical. And so far, simply recognizing that the issue exists hasn’t been enough to make us change our ways.
I think our path forward is clear: We need better methods for meaningfully integrating new credit assignment systems into our existing workflows in ways that make the information obvious, transparent and accessible in daily life. Heuristics become entrenched for a reason—we keep falling back on the “first author, last author” shortcut because it’s easy and makes sense—so let’s make ourselves some cheat sheets that better reflect our values. ORCID profile contribution badges across all linked papers could be a good start; a new category of summary statistics to accompany the h-index and i-index section on Google Scholar or cute graphical ways to display our identities as researchers on our CVs might also help. I’m sure you have other ideas, too. But no matter which version actually catches on, the collective goal should be a shift in our focus toward prioritizing the sociological utility of credit assignment, rather than simply capturing the data. Hopefully, a cultural shift will come along for the ride, and we’ll all be better at appropriately recognizing the valuable diversity of “big science” authorship contributions in modern neuroscience.
Editor’s note
Recommended reading
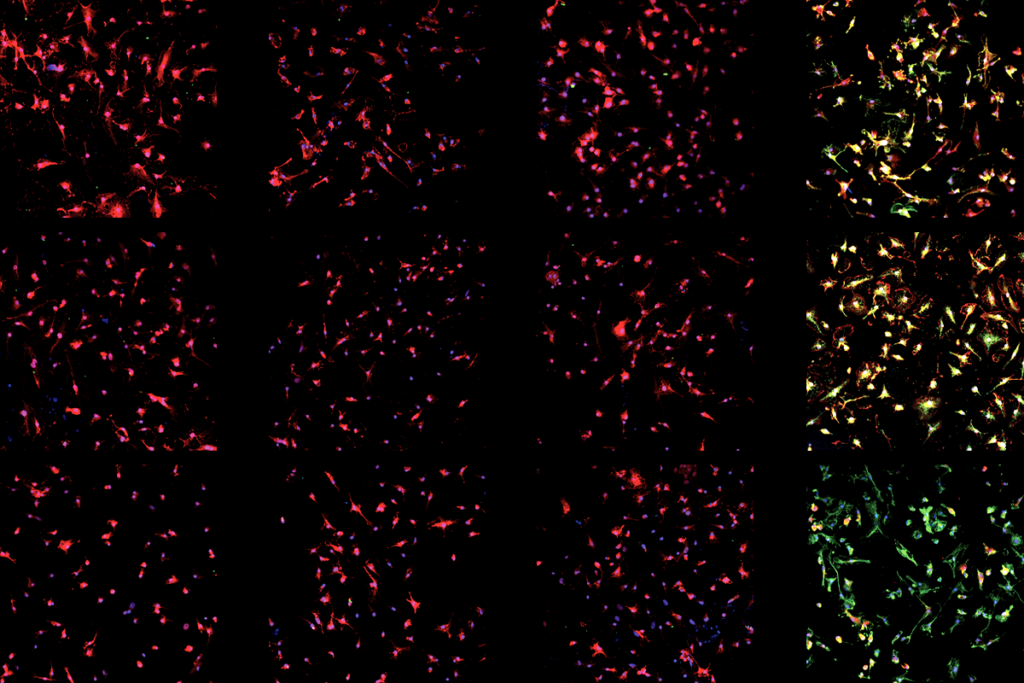
Authors correct image errors in Neuron paper that challenged microglia-to-neuron conversion
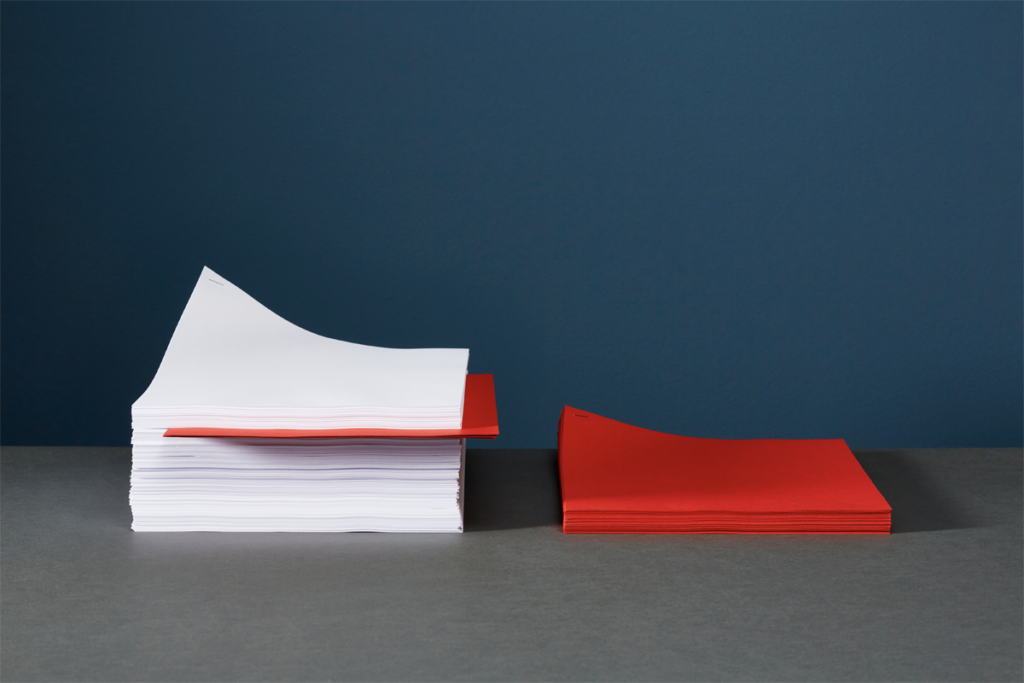
Exclusive: Issues with dozens of papers prompt inquiry into prolific stroke researcher
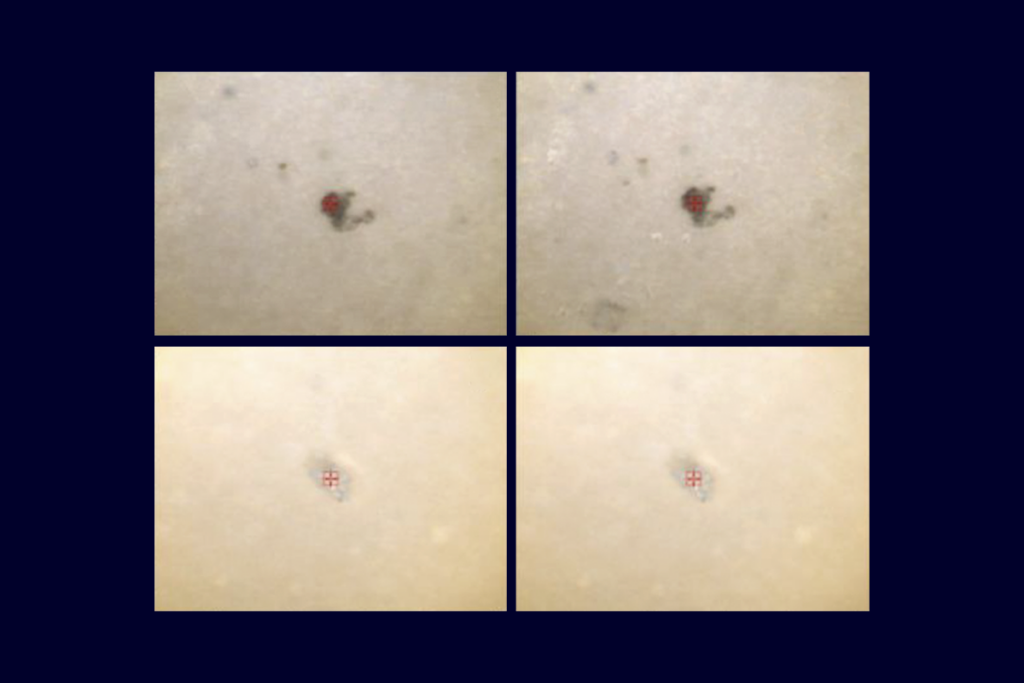
‘Spoonful of plastics in your brain’ paper has duplicated images
Explore more from The Transmitter
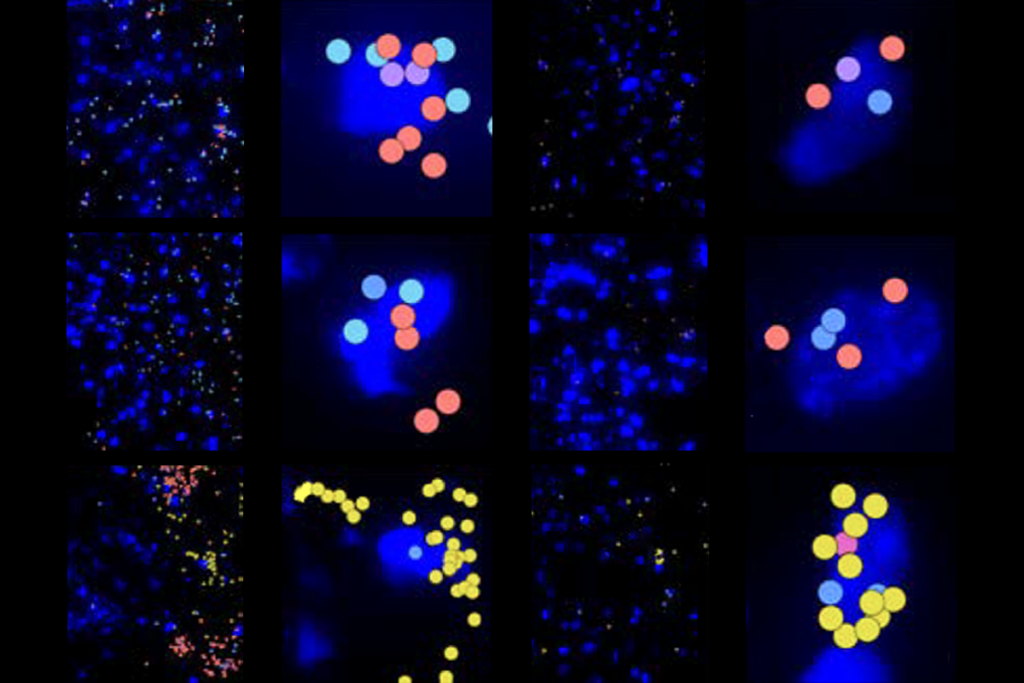
Machine learning spots neural progenitors in adult human brains
Xiao-Jing Wang outlines the future of theoretical neuroscience
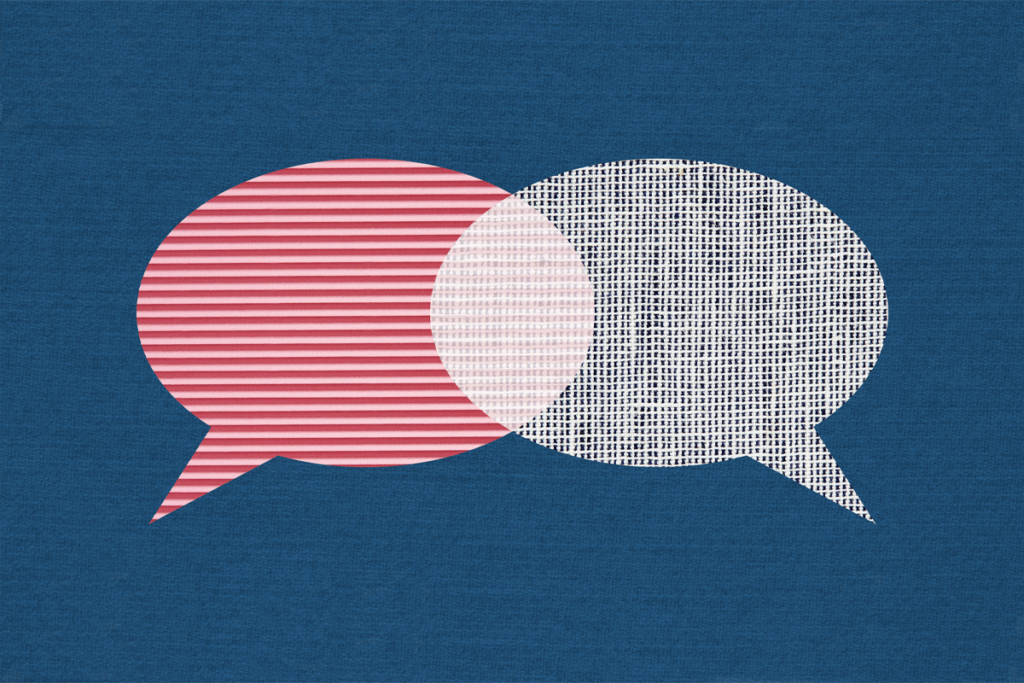