Machine-learning tool shows promise for autism diagnosis
It’s too soon to call it a diagnostic test for autism, but an algorithm that detects patterns in brain waves shows promise as one component of a screening battery for the disorder, say researchers familiar with the work.
It’s too soon to call it a diagnostic test for autism, but an algorithm that detects patterns in brain waves shows promise as one component of a screening battery for the disorder, say researchers familiar with the work.
The method can distinguish between infant siblings of children with autism and typical controls at 9 months of age with 80 percent accuracy, scientists reported in BMC Medicine last week1.
“It’s very interesting and very exciting and tells us a lot about where the field is going in the next few years,” says Timothy Roberts, vice-chair of research in radiology at the Children’s Hospital of Philadelphia. Roberts was not involved in the study.
Applying a computational tool called modified multiscale entropy to data from electroencephalograms (EEG), researchers at Children’s Hospital in Boston tracked the seemingly random waves of neurons firing in the brains of infants aged 3 to 24 months as they sat on their mothers’ laps, watching a research assistant blow bubbles.
Multiscale entropy is a young technique, but one that may be particularly well suited to investigating autism. The method relies on machine learning — a branch of artificial intelligence that uses algorithms to predict behavior based on empirical data. Used by communication engineers to calculate the randomness of signals in complex systems, multiscale entropy has been applied in cardiac research, successfully distinguishing electrical signals in healthy hearts from those in blocked and aging hearts2.
Complex signals:
The method is particularly well suited to autism research because the organ of interest in autism — the brain — is a complex network, communicating with itself and the rest of the body via lightning-fast electrical signals.
“Multiscale entropy is a lovely method for autism and I am extremely excited about the theoretical foundations of the study,” says Emmanuelle Tognoli, research assistant professor at the Center for Complex Systems and Brain Sciences at Florida Atlantic University.
However, this is the first time the method has been applied to EEG and must still be validated, Tognoli notes. She has used sophisticated EEG analyses to look at synchronization between brain regions in autism3.
In the new study, the researchers set out to compare electrophysiological activity in the brains of infant siblings of children with autism compared with those who have no family history of the disorder. Siblings of children with autism are considered to be 3 to 20 percent more likely to develop the disorder than the general population.
Using the algorithm, the researchers identified distinctive patterns of electrophysiological activity in the high-risk group, compared with typical controls. These differences peaked at age 9 months, though the high-risk girls in the study showed distinctive patterns of brain activity by 6 months of age.
After 12 months of age, however, the differences between high- and low-risk groups narrow. This key finding may be yet another example of an endophenotype, or underlying biological trait, shared among family members but not necessarily predictive of autism, researchers say.
“The real test will come when all these kids are 3 years old and you can diagnose them,” says Steven Petersen, chief of the neuropsychology division at Washington University in St. Louis.
Testing strategy:
Over the past two years, several different imaging methods combined with computational tools4 have been touted as diagnostic tools for autism.
Many of these studies are based on functional magnetic resonance imaging (fMRI), which tracks changes in blood flow related to neural activity in the brain. However, infants and young children must be sedated or asleep for fMRI studies, which limits their utility. “If they are asleep, you are not looking at active brain function,” says lead investigator William Bosl, a research scientist at Children’s Hospital, Boston.
EEG, which captures electrical activity along the scalp produced by the firing of neurons in the brain, and its cousin MEG — magnetoencephalography — which records magnetic fields produced by electrical currents in the brain, offer many advantages over fMRI, according to Bosl.
EEG and MEG are both simple, quick, non-invasive and relatively inexpensive, for example. Most importantly, they can monitor brain activity millisecond by millisecond, whereas fMRI tracks slower and more localized phenomena.
However, EEG also offers a less region-specific picture of brain function, something many researchers view as a liability. Individual EEG sensors can pick up signals from all regions of the brain, some weak, some of greater intensity, creating a complex picture of neuronal activity that shifts over time — like a symphony, with every instrument contributing to the overall swell of sound.
Ultimately, researchers say, multiple strategies are likely to be needed for creating a diagnostic battery of tests for autism.
“I would frankly be very surprised if there isn’t some combination [of tests] that work pretty well as a predictor in four years,” Petersen says. “Some of those will also tell us something about the underlying mechanisms, which is part two of the equation.”
References:
Recommended reading
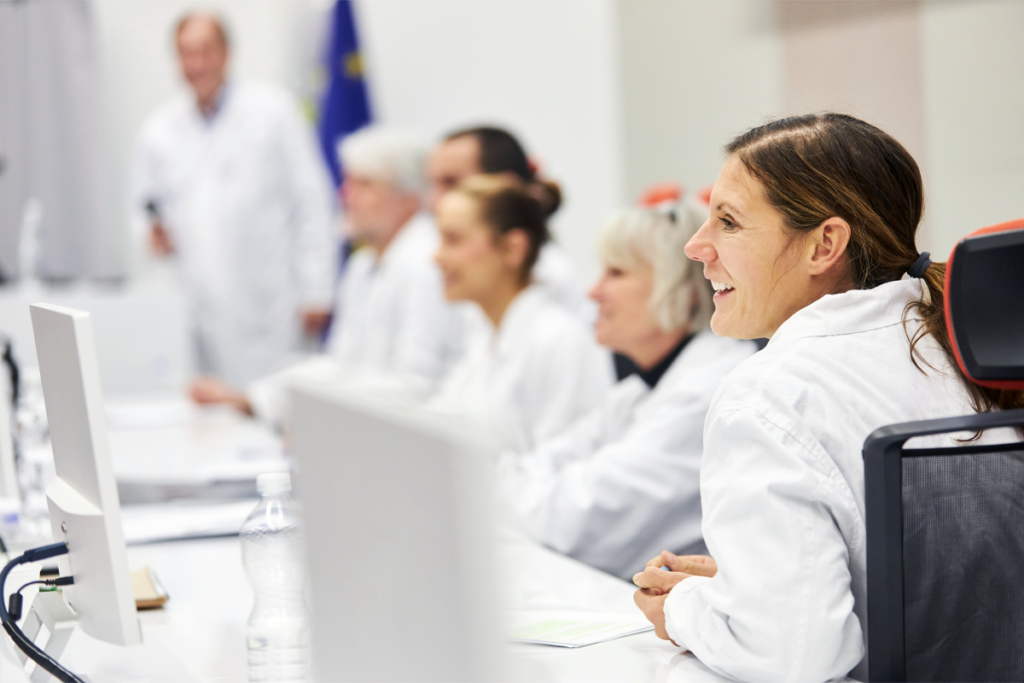
INSAR takes ‘intentional break’ from annual summer webinar series
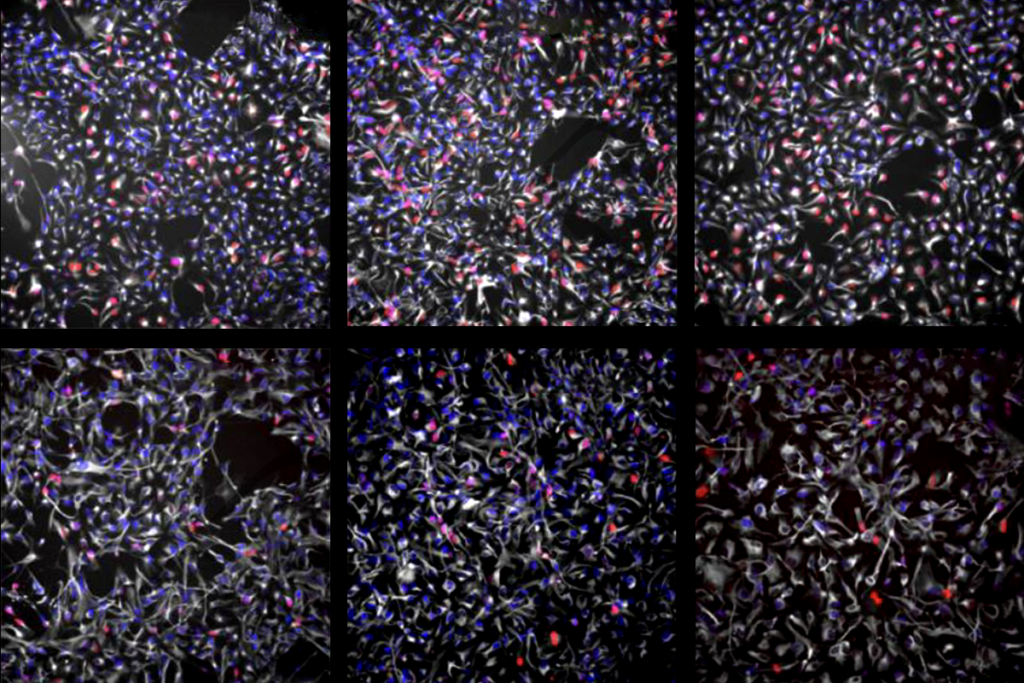
Dosage of X or Y chromosome relates to distinct outcomes; and more
Explore more from The Transmitter
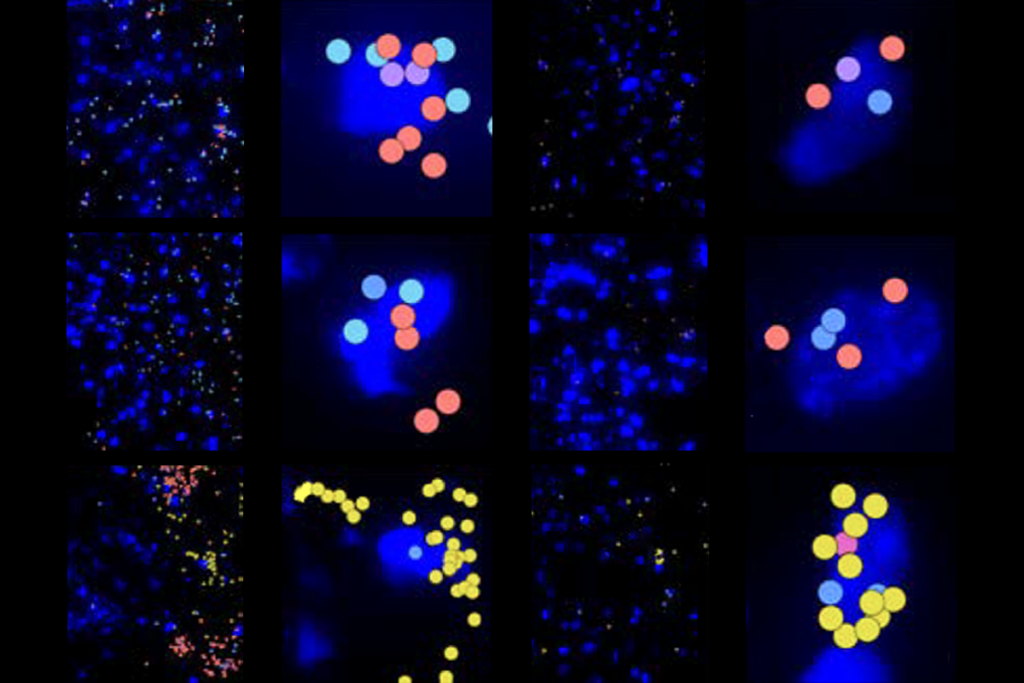
Machine learning spots neural progenitors in adult human brains
Xiao-Jing Wang outlines the future of theoretical neuroscience
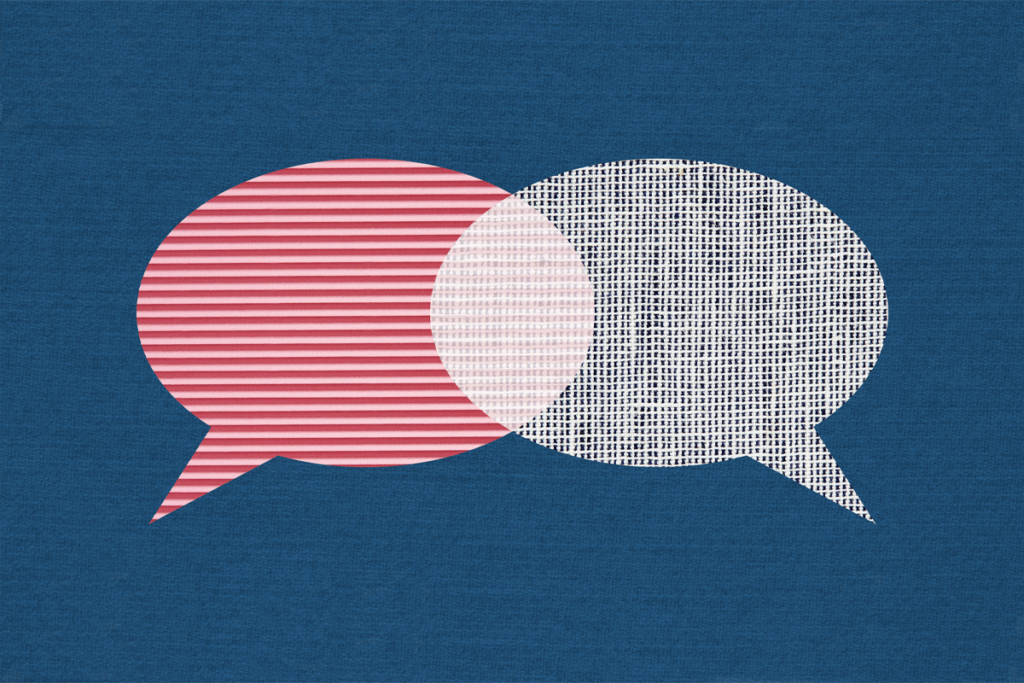