Ingfei Chen is a writer and editor in Northern California who likes telling stories about medicine, science and the environment. Her articles have published in The New York Times, Science, KQED Mindshift, Scientific American and Smithsonian, among others.
Ingfei Chen
Freelance writer
From this contributor
What baby siblings can teach us about autism
Studies of infants at risk for autism have not yielded a test to predict who will eventually be diagnosed. But they have transformed our understanding of the condition.
The gene hunters
Criss-crossing the globe on a quest for unusual DNA, researchers have discovered a rare mutation that promises insights into both epilepsy and autism — and points to a treatment.
Wide awake: Why children with autism struggle with sleep
Half of children who have autism have trouble falling or staying asleep, which may make their symptoms worse. Scientists are just beginning to explore what goes wrong in the midnight hour.
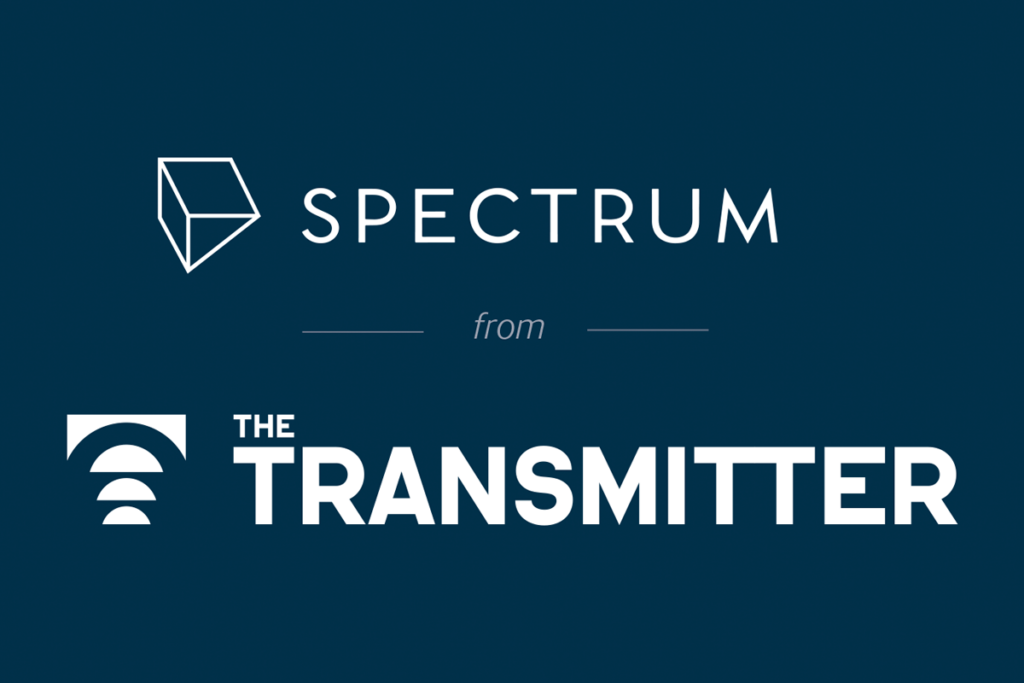
Wide awake: Why children with autism struggle with sleep
Explore more from The Transmitter
On the importance of reading (just not too much)
The real fun of being a neuroscientist, and maybe the key to asking and answering new questions, is to think big and take intellectual risks.
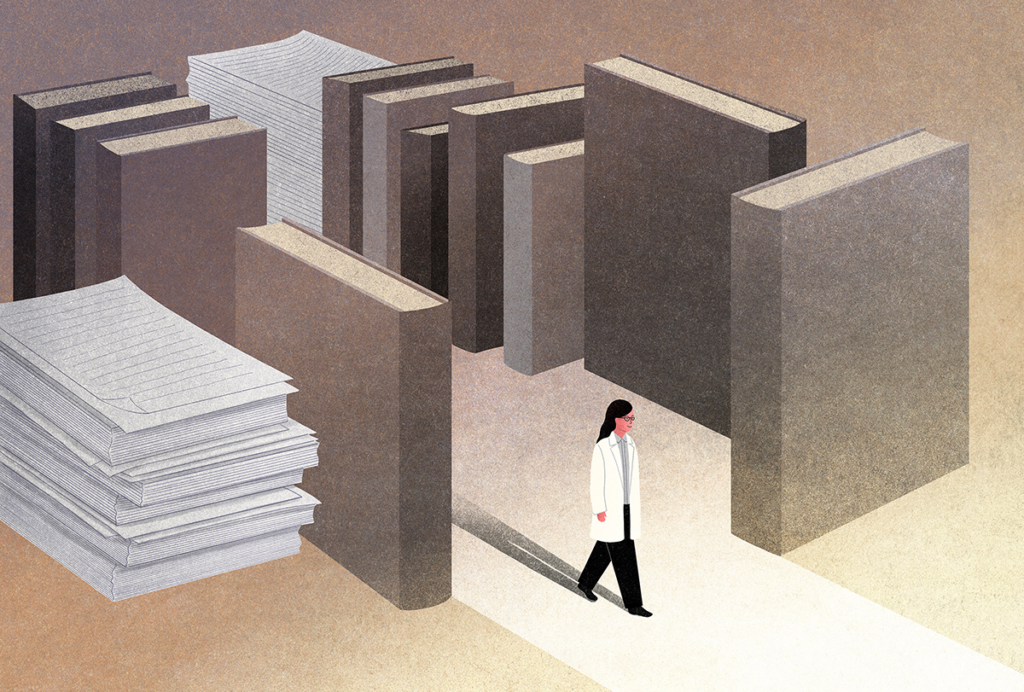
On the importance of reading (just not too much)
The real fun of being a neuroscientist, and maybe the key to asking and answering new questions, is to think big and take intellectual risks.
How developing neurons simplify their search for a synaptic mate
Streamlining the problem from 3D to 1D eases the expedition—a strategy the study investigators deployed to rewire an olfactory circuit in flies.
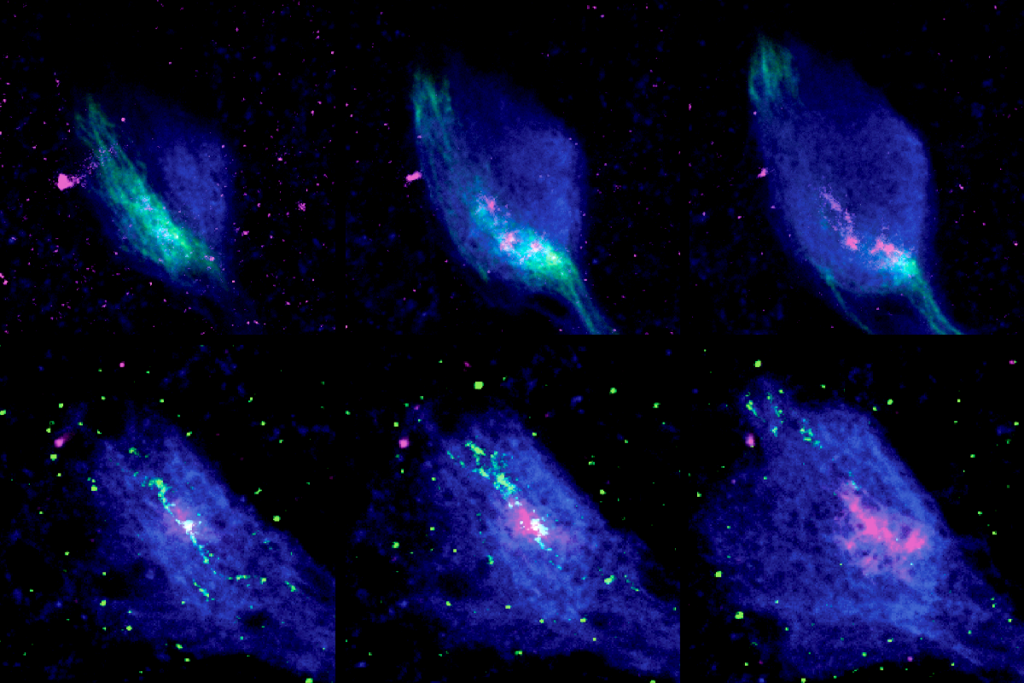
How developing neurons simplify their search for a synaptic mate
Streamlining the problem from 3D to 1D eases the expedition—a strategy the study investigators deployed to rewire an olfactory circuit in flies.
NIH autism database announcement raises concerns among researchers
The U.S. National Institutes of Health announced a plan to pour $50 million into data science projects intended to investigate the condition’s causes, but the initiative’s short timeline and other atypicalities have prompted questions.
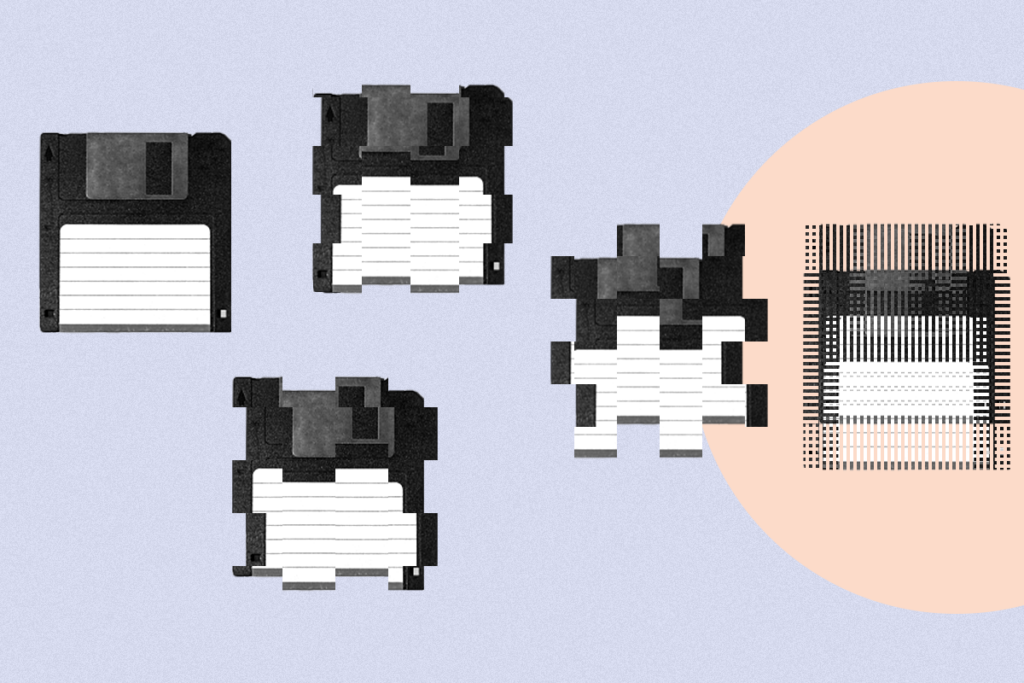
NIH autism database announcement raises concerns among researchers
The U.S. National Institutes of Health announced a plan to pour $50 million into data science projects intended to investigate the condition’s causes, but the initiative’s short timeline and other atypicalities have prompted questions.