Julie Forman-Kay is program head in molecular medicine at the Hospital for Sick Children in Toronto, Canada. She received her B.Sc. in chemistry from the Massachusetts Institute of Technology and her Ph.D. in molecular biophysics andd biochemistry from Yale University. The major focus of her lab is to provide biological insights into how dynamic properties of proteins are related to function and methodological tools to enable better understanding of dynamic and disordered states. Most recently, her lab has probed the biophysics of protein phase separation and how it regulates cellular condensates and biological function.
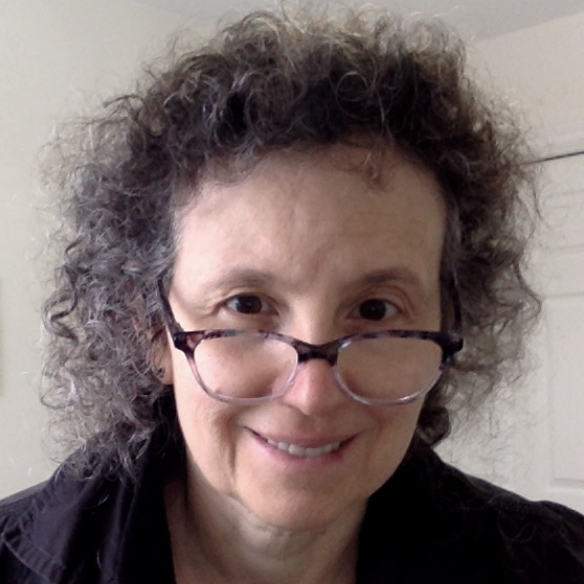
Julie Forman-Kay
Program head
Hospital for Sick Children
From this contributor
How microscopic ‘condensates’ in cells might contribute to autism
A controversial idea about how cells compartmentalize their contents into droplets — like beads of oil in water — could be key to understanding autism, says Julie Forman-Kay.
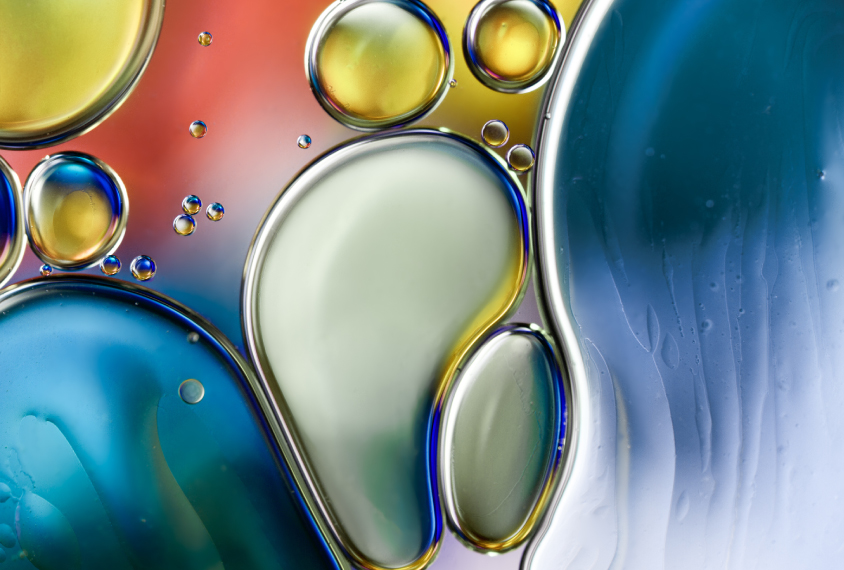
How microscopic ‘condensates’ in cells might contribute to autism
Explore more from The Transmitter
Sharing Africa’s brain data: Q&A with Amadi Ihunwo
These data are “virtually mandatory” to advance neuroscience, says Ihunwo, a co-investigator of the Brain Research International Data Governance & Exchange (BRIDGE) initiative, which seeks to develop a global framework for sharing, using and protecting neuroscience data.
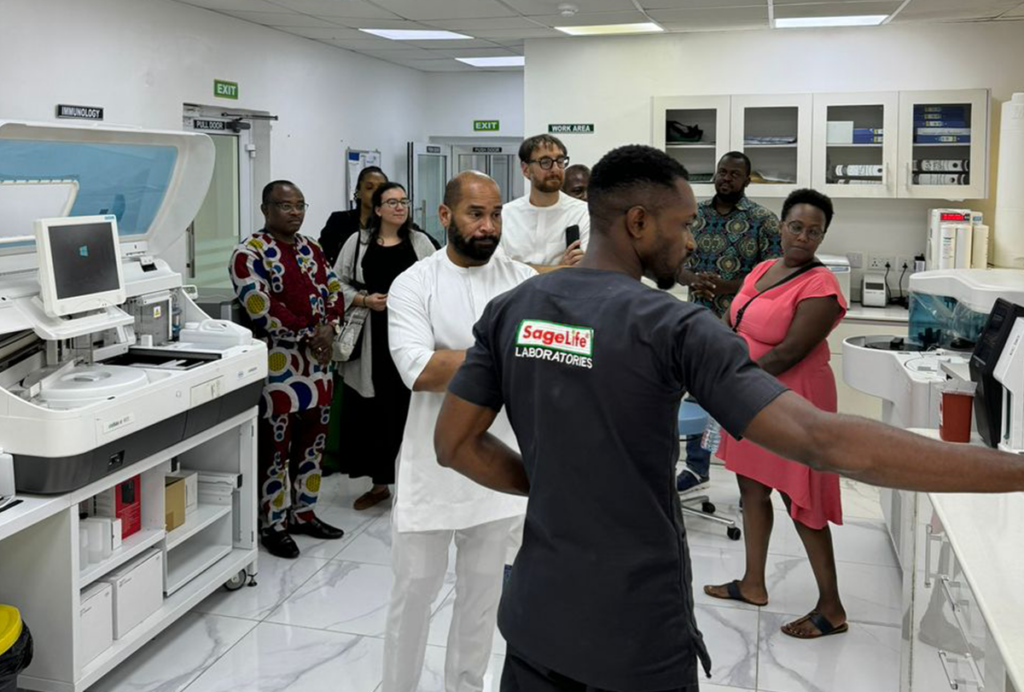
Sharing Africa’s brain data: Q&A with Amadi Ihunwo
These data are “virtually mandatory” to advance neuroscience, says Ihunwo, a co-investigator of the Brain Research International Data Governance & Exchange (BRIDGE) initiative, which seeks to develop a global framework for sharing, using and protecting neuroscience data.
Cortical structures in infants linked to future language skills; and more
Here is a roundup of autism-related news and research spotted around the web for the week of 19 May.
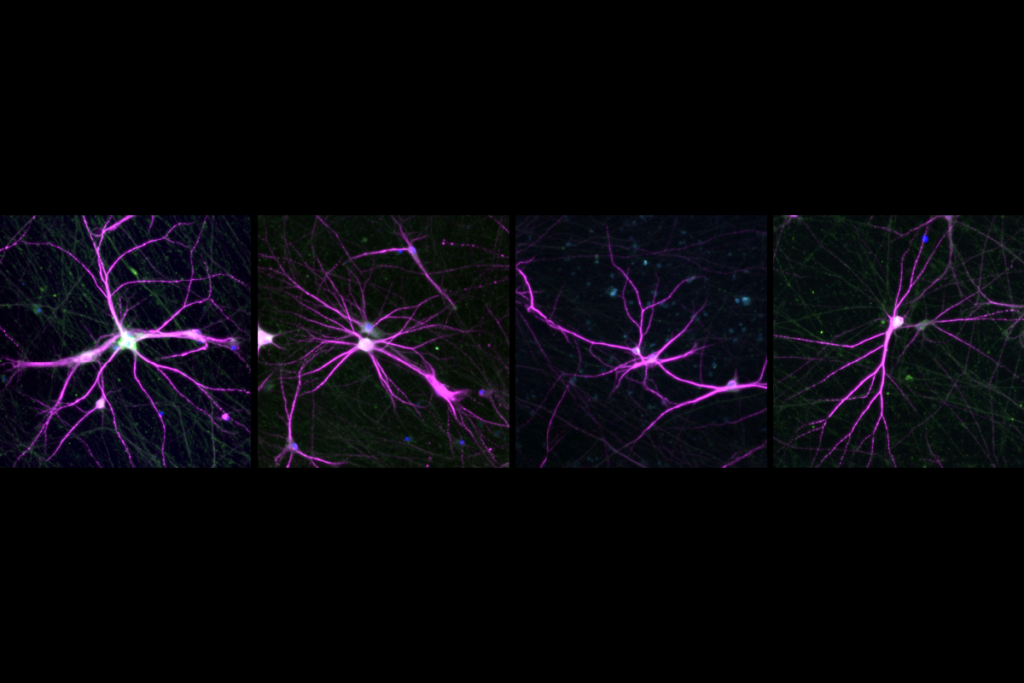
Cortical structures in infants linked to future language skills; and more
Here is a roundup of autism-related news and research spotted around the web for the week of 19 May.
The BabyLM Challenge: In search of more efficient learning algorithms, researchers look to infants
A competition that trains language models on relatively small datasets of words, closer in size to what a child hears up to age 13, seeks solutions to some of the major challenges of today’s large language models.
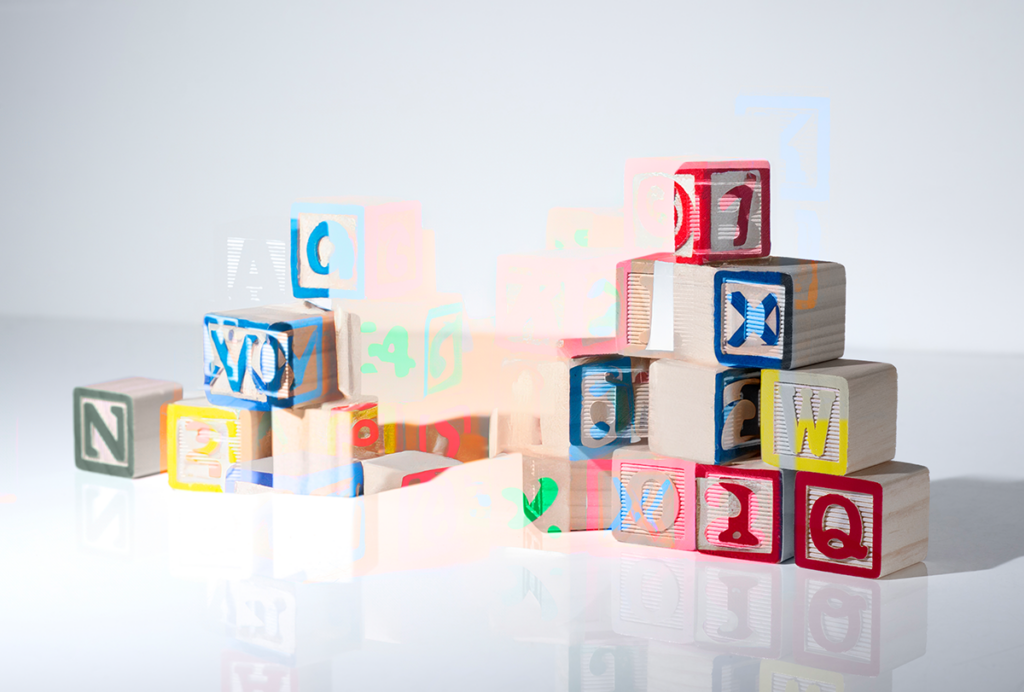
The BabyLM Challenge: In search of more efficient learning algorithms, researchers look to infants
A competition that trains language models on relatively small datasets of words, closer in size to what a child hears up to age 13, seeks solutions to some of the major challenges of today’s large language models.