Lindsay Shea is director of the Policy and Analytics Center at the A.J. Drexel Autism Institute at Drexel University in Philadelphia, Pennsylvania. She is also interim leader of the institute’s Life Course Outcomes Research Program. She focuses on research that is conducted in partnership with and that directly impacts communities and policymakers.
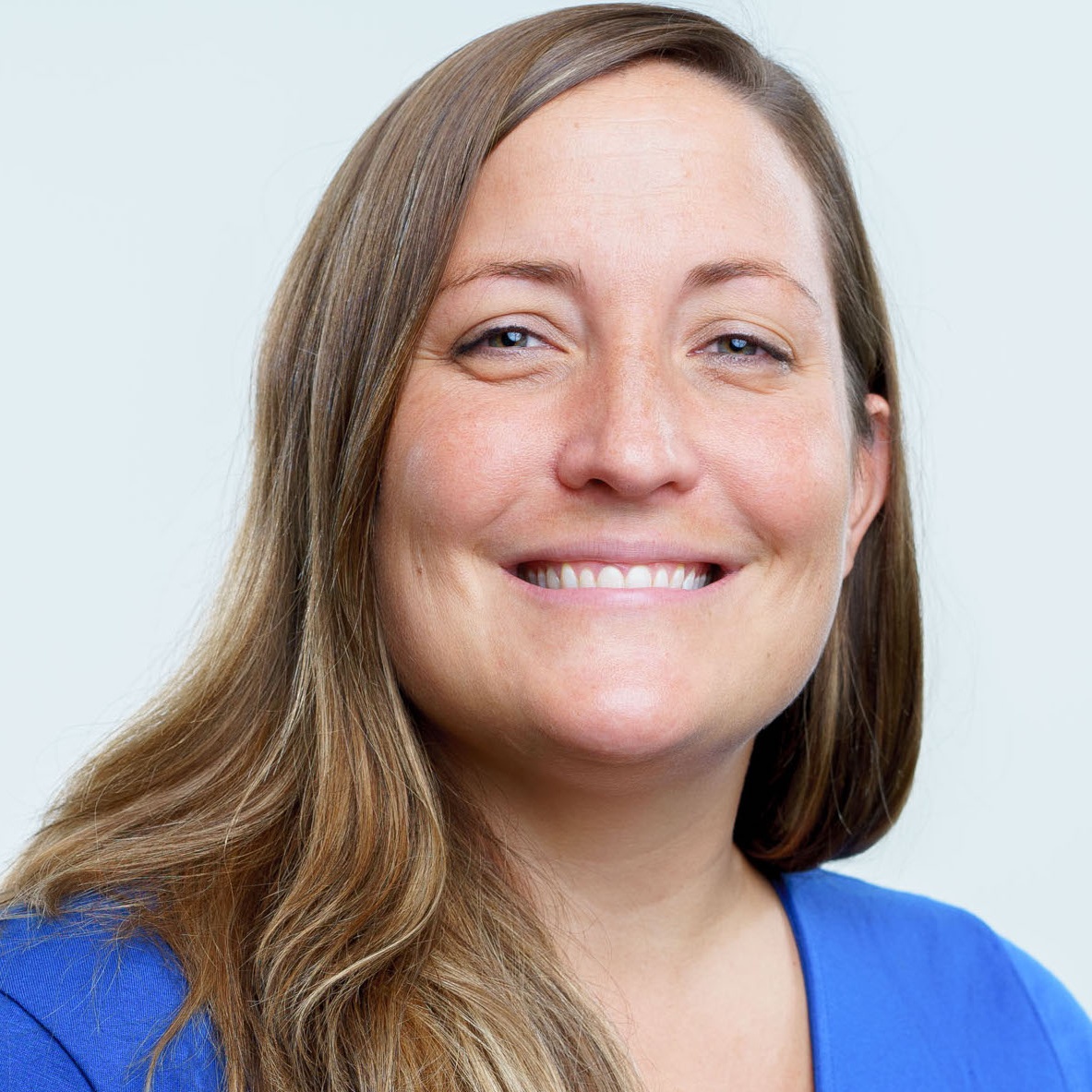
Lindsay Shea
Director, Policy and Analytics Center
A.J. Drexel Autism Institute
From this contributor
Pitfalls in using autism claims data: Q&A with Lindsay Shea
Insurance claims data are useful for autism research, but the field needs to standardize how they are mined, Shea says.
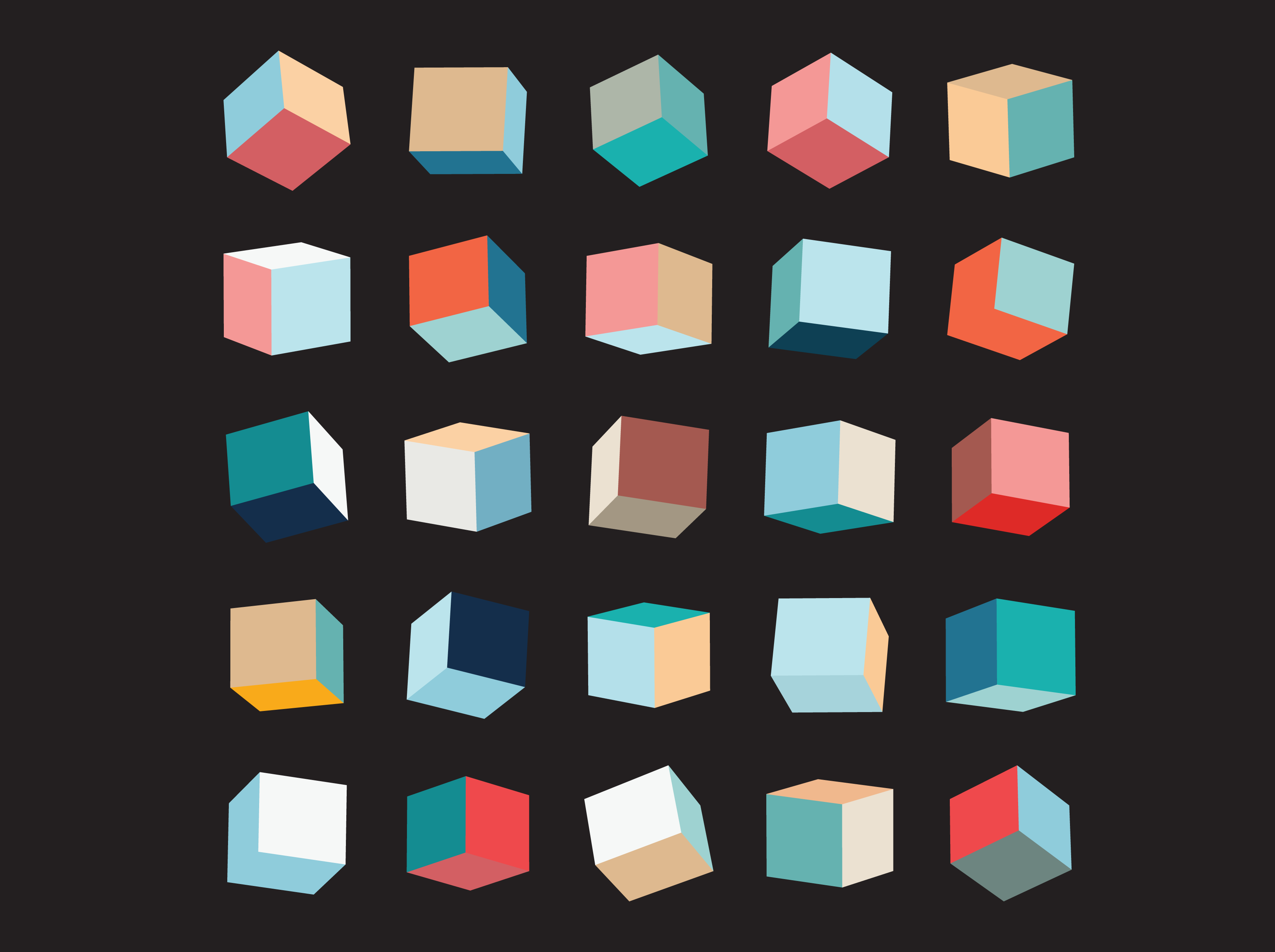
Pitfalls in using autism claims data: Q&A with Lindsay Shea
Explore more from The Transmitter
Federal funding cuts imperil next generation of autism researchers
As the International Society for Autism Research’s annual meeting begins, its next president reflects on a brewing crisis.
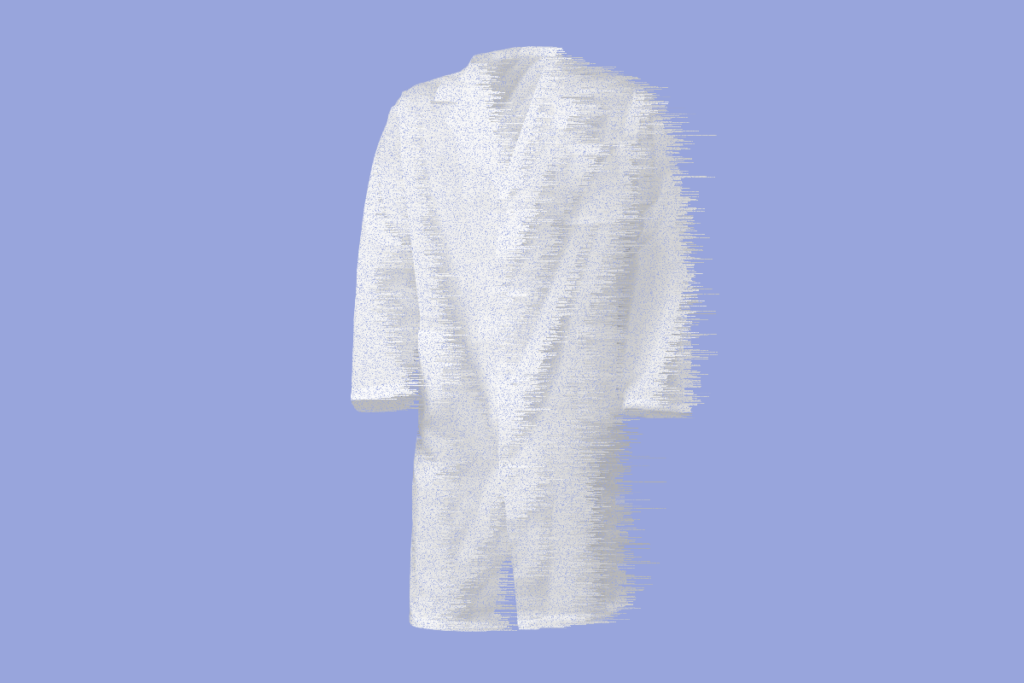
Federal funding cuts imperil next generation of autism researchers
As the International Society for Autism Research’s annual meeting begins, its next president reflects on a brewing crisis.
Null and Noteworthy: Reanalysis contradicts report of immune memory in astrocytes
The analysis, which has not yet been peer reviewed, attributes the finding to misidentified immune cells instead.
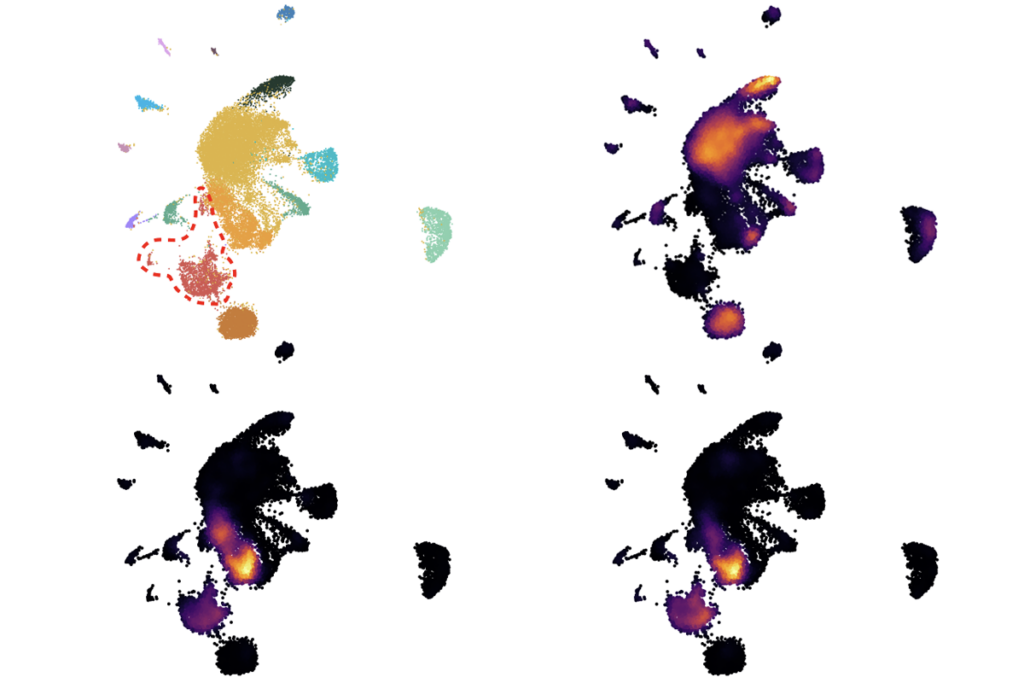
Null and Noteworthy: Reanalysis contradicts report of immune memory in astrocytes
The analysis, which has not yet been peer reviewed, attributes the finding to misidentified immune cells instead.
Documenting decades of autism prevalence; and more
Here is a roundup of autism-related news and research spotted around the web for the week of 28 April.
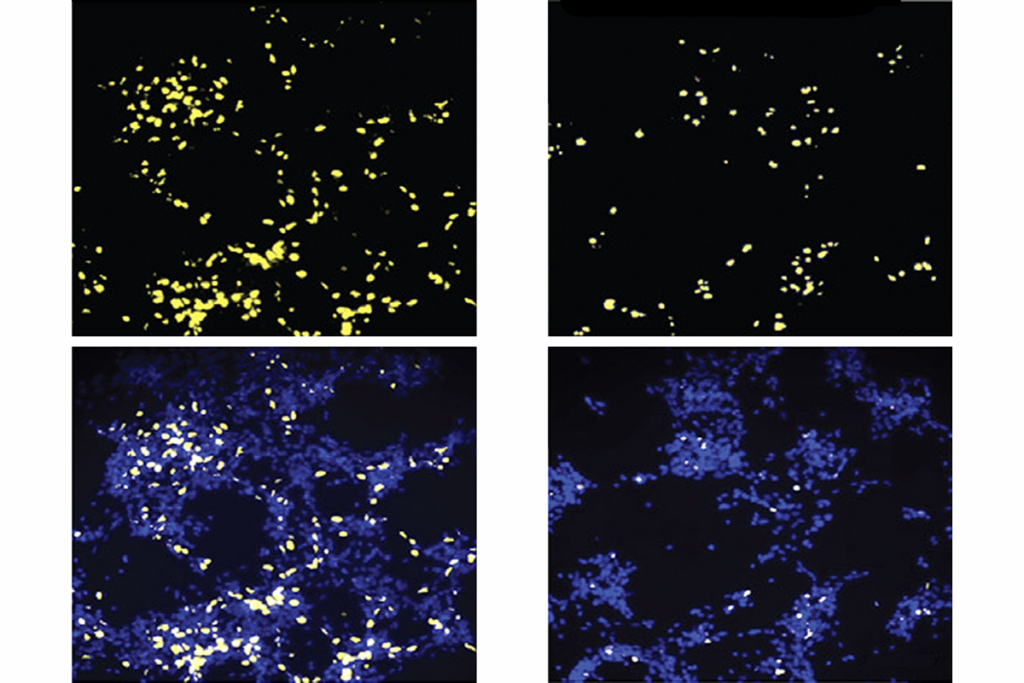
Documenting decades of autism prevalence; and more
Here is a roundup of autism-related news and research spotted around the web for the week of 28 April.