Mike Hawrylycz joined the Allen Institute for Brain Science in Seattle, Washington, in 2003 as director of informatics and one of the institute’s first staff. His group is responsible for developing algorithms and computational approaches in the development of multimodal brain atlases, and in data analysis and annotation. Hawrylycz has worked in a variety of applied mathematics and computer science areas, addressing challenges in consumer and investment finance, electrical engineering and image processing, and computational biology and genomics. He received his Ph.D. in applied mathematics at the Massachusetts Institute of Technology and subsequently was a postdoctoral researcher at the Center for Nonlinear Studies at the Los Alamos National Laboratory in New Mexico.
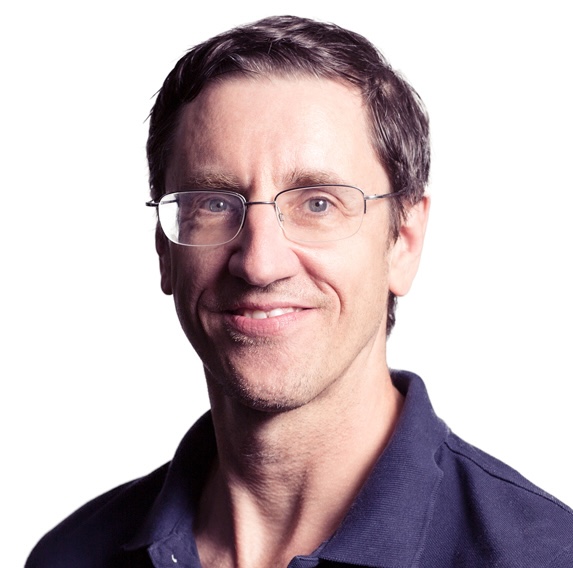
Michael Hawrylycz
Investigator
Allen Institute for Brain Science
From this contributor
Knowledge graphs can help make sense of the flood of cell-type data
These tools, widely used in the technology industry, could provide a foundation for the study of brain circuits.
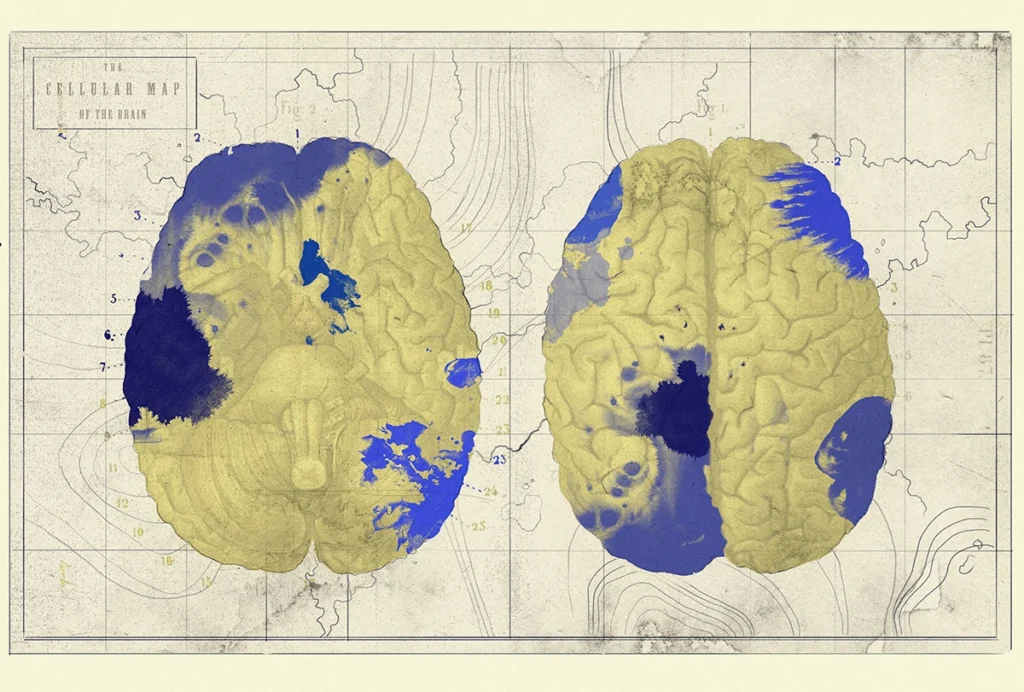
Knowledge graphs can help make sense of the flood of cell-type data
Explore more from The Transmitter
This paper changed my life: Victoria Abraira on a tasty link between circuits and behavior
The findings from Charles Zuker’s lab put the taste system on the map, revealing that some fundamental principles of behavior are hardwired.
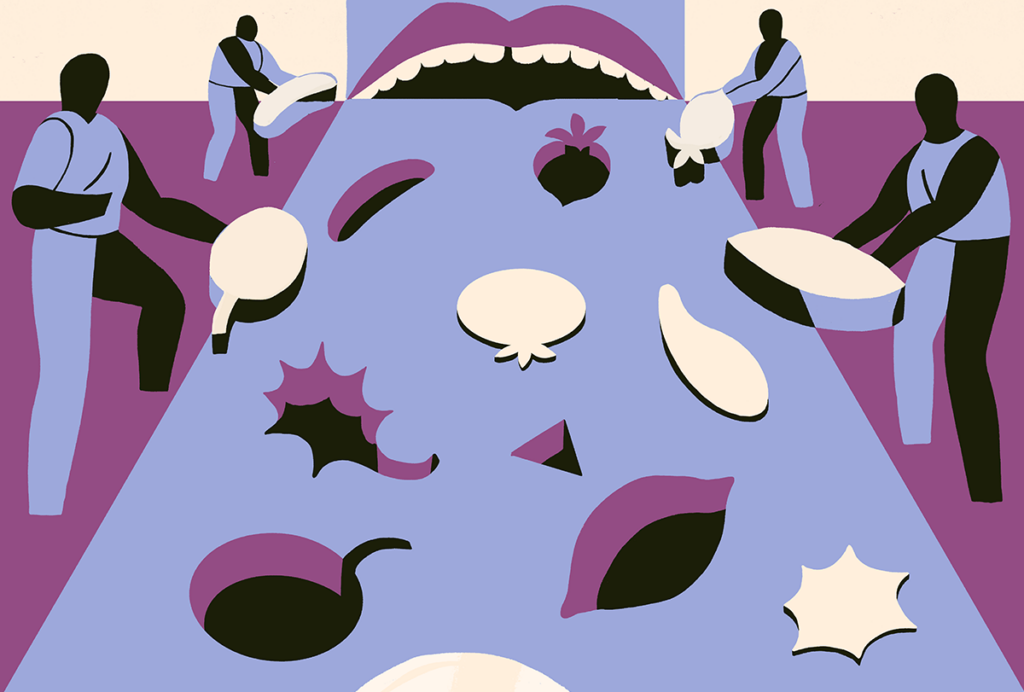
This paper changed my life: Victoria Abraira on a tasty link between circuits and behavior
The findings from Charles Zuker’s lab put the taste system on the map, revealing that some fundamental principles of behavior are hardwired.
Neurophysiologic distinction between autism and schizophrenia; and more
Here is a roundup of autism-related news and research spotted around the web for the week of 21 July.
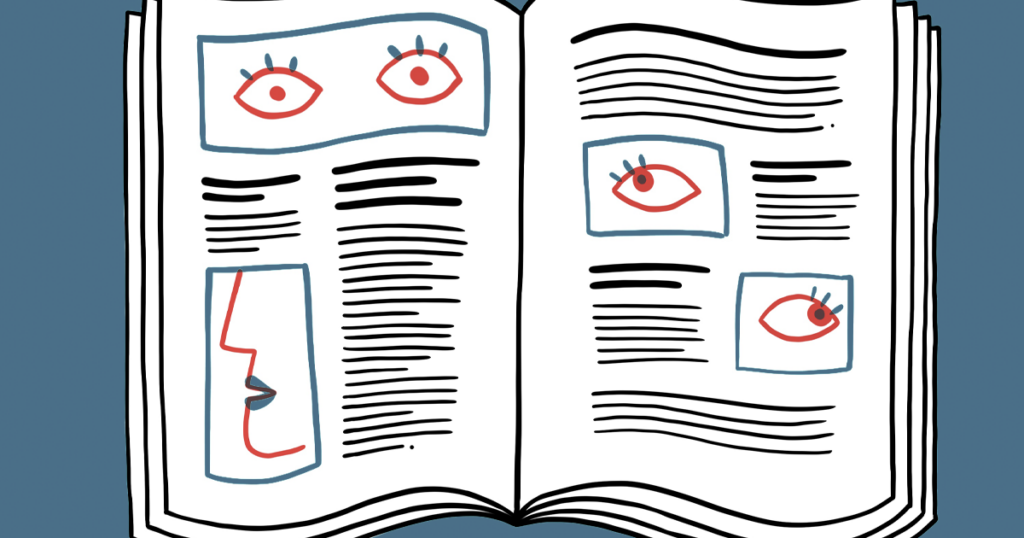
Neurophysiologic distinction between autism and schizophrenia; and more
Here is a roundup of autism-related news and research spotted around the web for the week of 21 July.
Breaking the jar: Why NeuroAI needs embodiment
Brain function is inexorably shaped by the body. Embracing this fact will benefit computational models of real brain function, as well as the design of artificial neural networks.
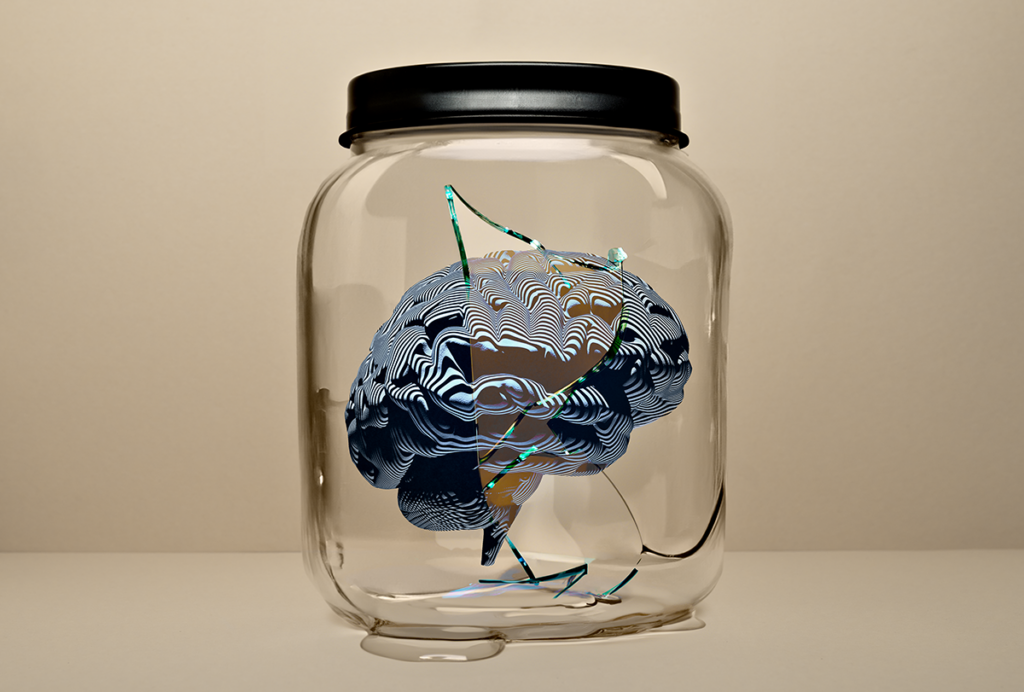
Breaking the jar: Why NeuroAI needs embodiment
Brain function is inexorably shaped by the body. Embracing this fact will benefit computational models of real brain function, as well as the design of artificial neural networks.