Nico Dosenbach is associate professor of neurology at Washington University School of Medicine. His research as a systems neuroscientist is focused on pushing resting-state functional connectivity MRI (RSFC), functional MRI (fMRI) and diffusion tensor imaging (DTI) to the level of individual patients. To create and annotate the connectomes of individuals he is working to improve the signal-to-noise, spatial resolution and replicability of RSFC, DTI and fMRI data.
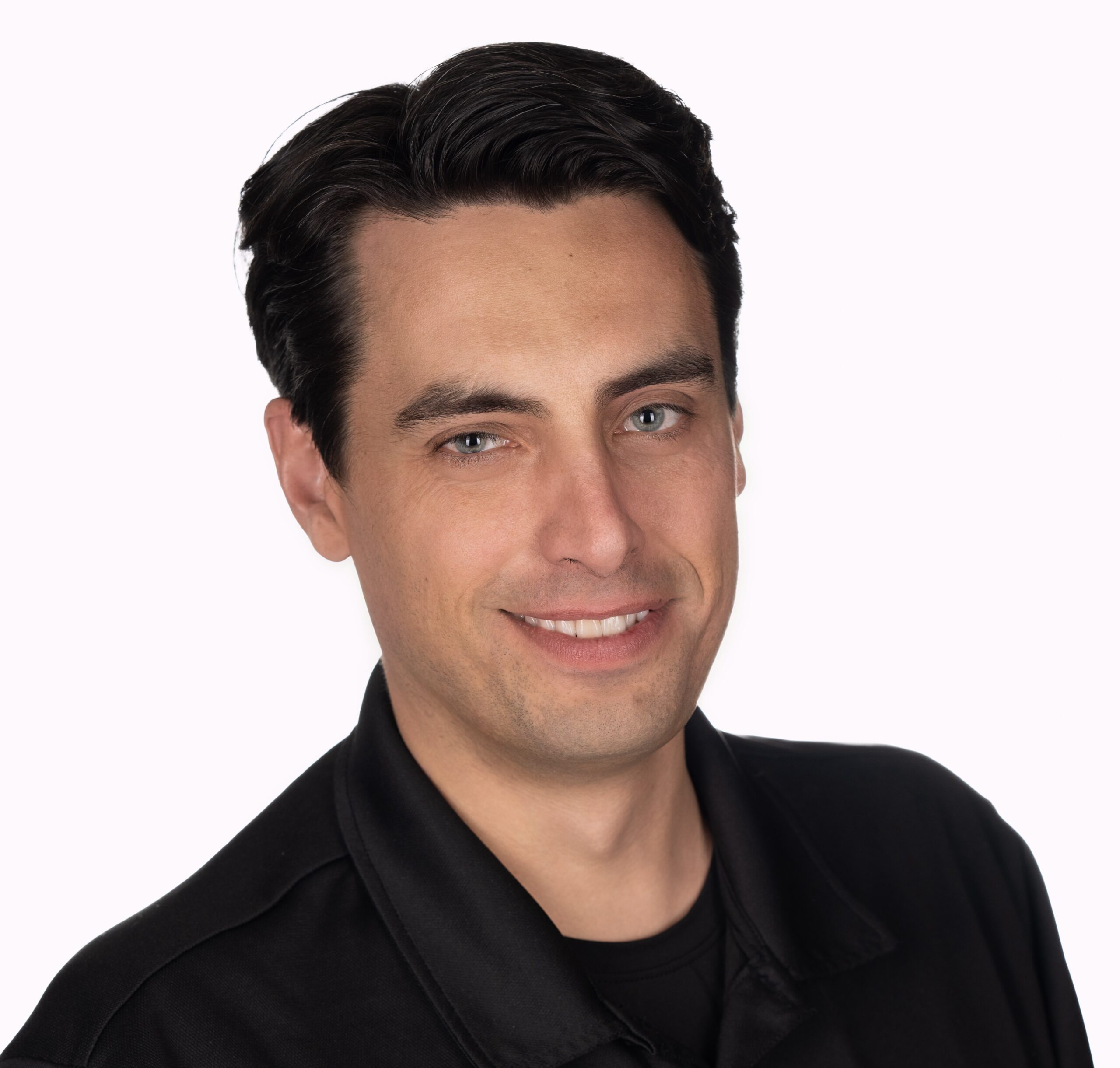
Nico Dosenbach
Associate professor of neurology
Washington University School of Medicine
From this contributor
Breaking down the winner’s curse: Lessons from brain-wide association studies
We found an issue with a specific type of brain imaging study and tried to share it with the field. Then the backlash began.
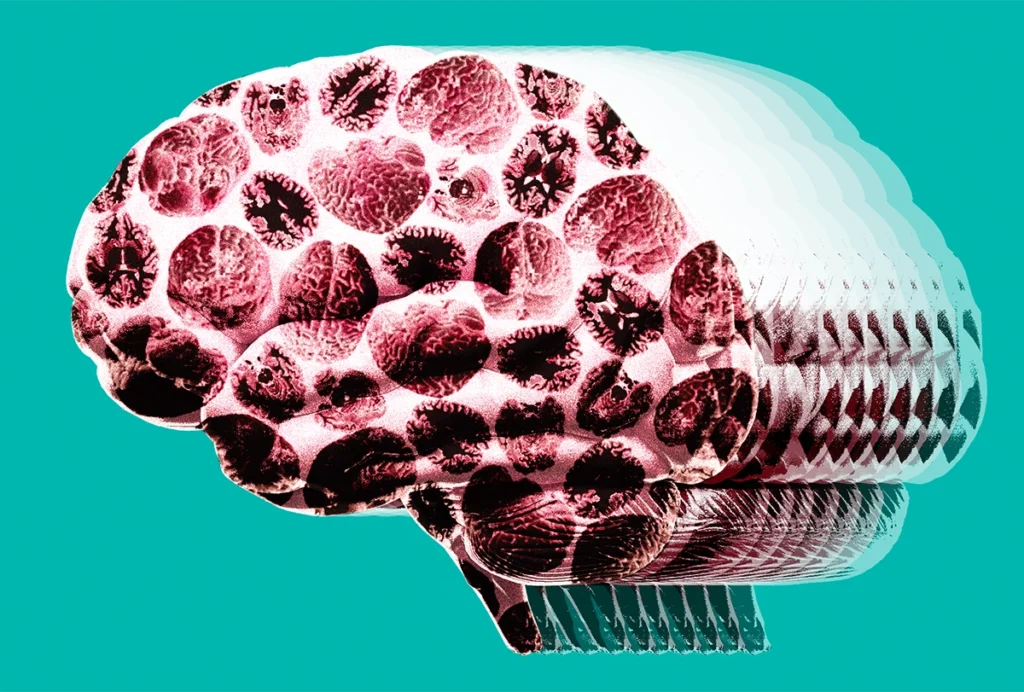
Breaking down the winner’s curse: Lessons from brain-wide association studies
Explore more from The Transmitter
More than two dozen papers by neural tube researcher come under scrutiny
One of the studies, published in 2021 in Science Advances, received an editorial expression of concern on 21 May, after the journal learned that an institutional review of alleged image problems is underway.
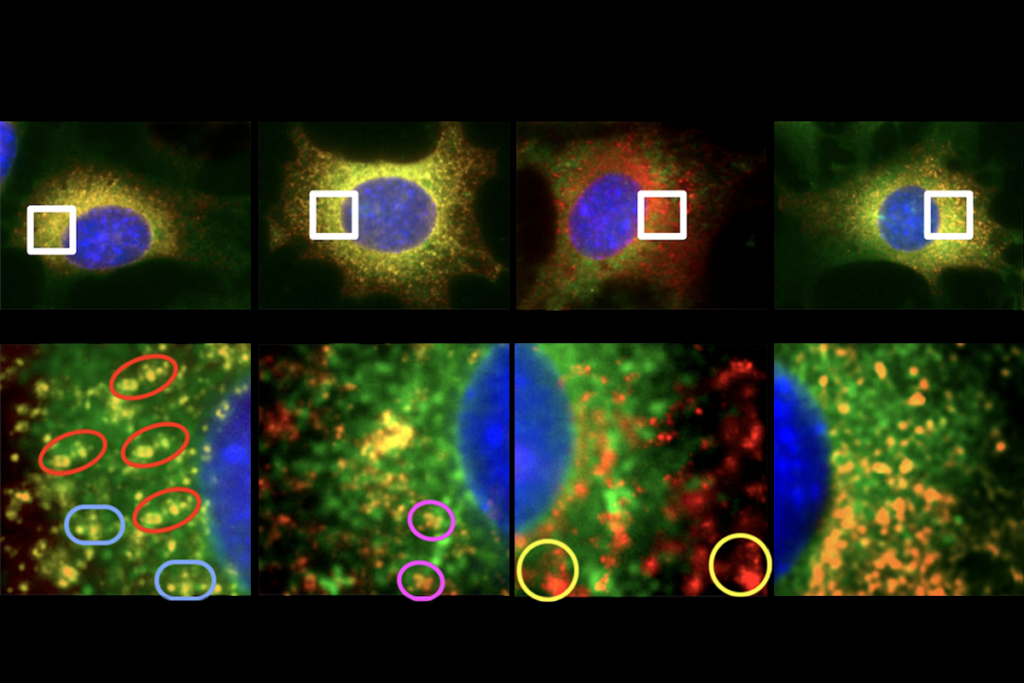
More than two dozen papers by neural tube researcher come under scrutiny
One of the studies, published in 2021 in Science Advances, received an editorial expression of concern on 21 May, after the journal learned that an institutional review of alleged image problems is underway.
On the importance of reading (just not too much)
The real fun of being a neuroscientist, and maybe the key to asking and answering new questions, is to think big and take intellectual risks.
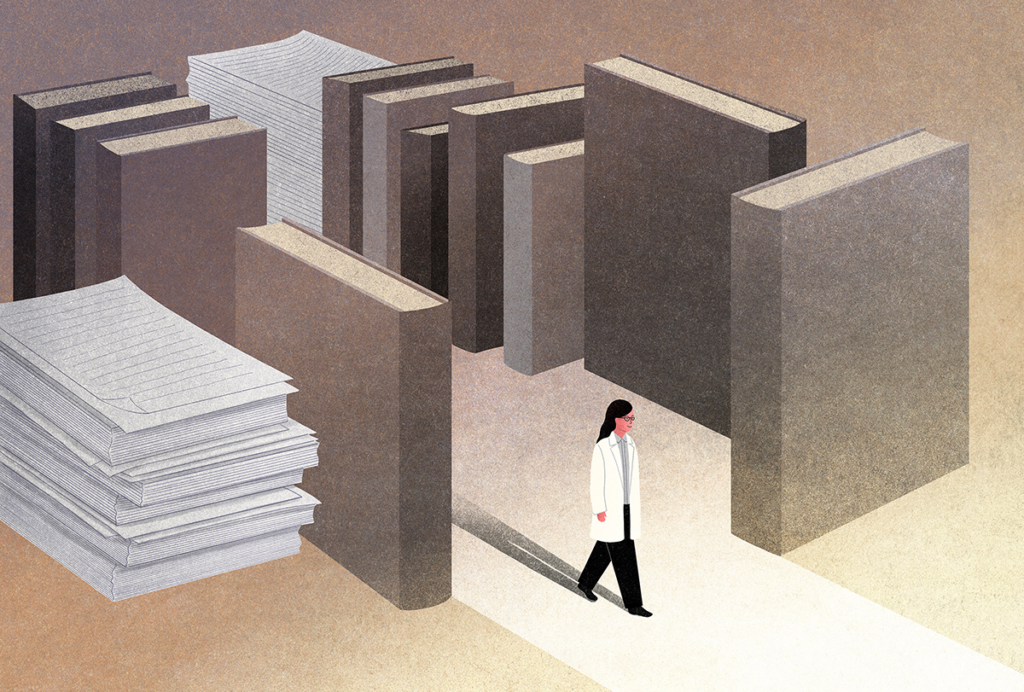
On the importance of reading (just not too much)
The real fun of being a neuroscientist, and maybe the key to asking and answering new questions, is to think big and take intellectual risks.
How developing neurons simplify their search for a synaptic mate
Streamlining the problem from 3D to 1D eases the expedition—a strategy the study investigators deployed to rewire an olfactory circuit in flies.
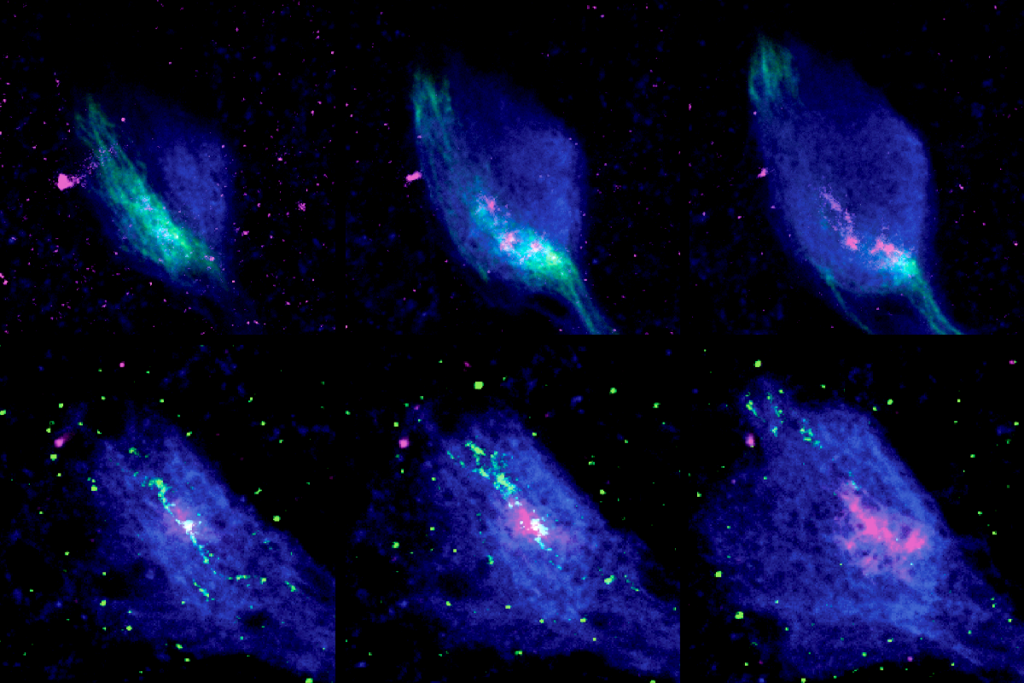
How developing neurons simplify their search for a synaptic mate
Streamlining the problem from 3D to 1D eases the expedition—a strategy the study investigators deployed to rewire an olfactory circuit in flies.