Pooling data points to new potential treatment for spinal cord injury
By gathering raw data from multiple labs, we identified an overlooked predictor of recovery after spinal cord injury. Many more insights remain trapped in scattered data.
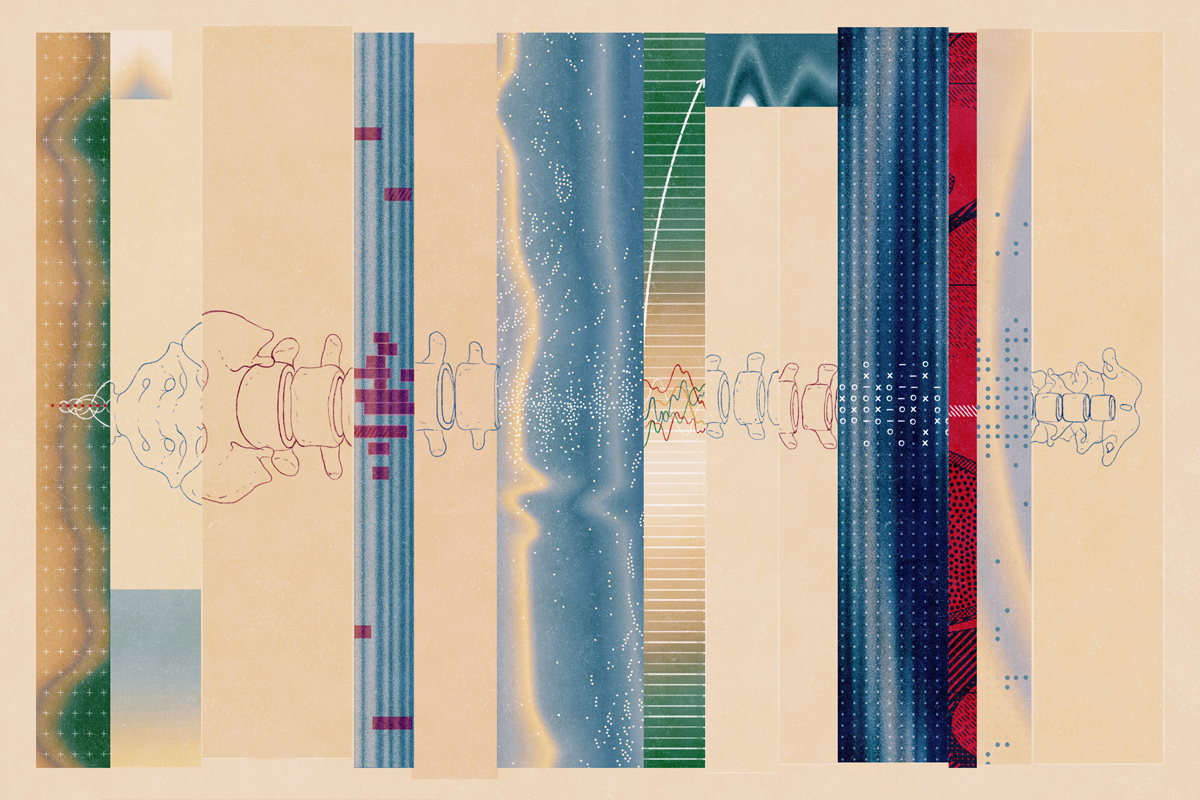
Our team was an early adopter of data-sharing, by necessity. As spinal cord injury researchers, we work on what is essentially an orphan disease — there are far fewer people affected by spinal cord injury than by heart disease, cancer or stroke. Because of the relative rarity of the condition, it is difficult for a single research group, working in isolation, to develop therapeutic approaches and get definitive answers about what treatments work best. Collaborative data-sharing offers important opportunities to enhance the development and testing of new therapies.
We have demonstrated firsthand just how powerful data-sharing can be, identifying a simple and previously overlooked predictor of recovery from spinal cord injury.
Starting in 2010, our team at the Brain and Spinal Injury Center at the University of California, San Francisco began collecting data from a coalition of spinal cord injury researchers to create large pools of raw research data from numerous experimental models and research laboratories. Using advanced analytic strategies, such as artificial intelligence and machine learning (AI/ML), we waded through thousands of variables to uncover a robust predictor of the recovery of walking ability following spinal cord injury: blood pressure. Specifically, our machine-learning tools found that rats with blood pressure that was either too high or too low at the time of the spinal cord injury had much worse recovery of motor function.
We initially wondered whether something as simple and medically treatable as blood pressure could really impact long-term recovery after spinal cord injury. After all, AI/ML tools can sometimes generate questionable results. Large language models such as GPT-3, for example, can produce convincing but completely false responses to text queries, a phenomenon known as “AI hallucination.” In our application, AI/ML models may classify patient groups based on features that do not extend to other patient populations, known in the field as “overfitting.” For an AI finding to be considered true, it must generalize to new data.
To test the generalizability of our findings, we approached members of a multicenter animal spinal cord injury study involving eight institutions. We recovered animal-care records for more than 1,400 rats collected in the 1990s to test our AI-generated hypothesis that blood pressure in the animal operating room at the time of the injury predicts the extent of recovery. To our great relief, the results reaffirmed that blood pressure within a narrow “Goldilocks zone” — not too high, not too low — predicted the best long-term recovery of function in these experimental animals.
B
ut what of human patients? To test our AI-generated blood pressure hypothesis in people, we partnered with two major level 1 trauma centers in the San Francisco Bay Area to mine patient records from the operating room after traumatic spinal cord injury. The results demonstrated that in humans, as in rats, mean arterial blood pressure in the operating room strongly predicts long-term recovery of motor function. The results suggest that tightly controlling blood pressure soon after injury or during spinal cord trauma surgery could improve outcomes. We are in the process of further validating and refining these results to craft clinical care guidelines and protocols.Spinal cord injury is just the beginning. We are applying this approach to other biomedical problems where pooled data is reused, remixed and repurposed for novel discoveries using AI and ML. Low back pain, traumatic brain injury and concussion, dementia and vascular pathologies are all complex problems with a baffling array of biological and non-biological factors that interact to produce symptoms. AI tools able to quickly integrate patient information with records from thousands of previous cases may allow us to understand this complexity for each new patient and help devise the best treatment strategy for their recovery.
Our group, for example, has worked with data — shared from multiple institutions — on traumatic brain injuries and concussions, a particularly challenging set of conditions. Brain injuries are significantly more common than spinal cord injury, but individual cases can vary widely. Differences in severity, location, medical history and treatment center can all lead to vastly different outcomes. Sharing data across centers has substantially aided our efforts to identify variables that can help predict outcomes, even across widely differing patients, using metrics that can be measured in most clinical centers. Recently, members of our team, alongside physician researchers, have been able to identify proteins in the blood and other clinical measures that can aid doctors in diagnosing and predicting a person’s outcome. By including data from multiple centers and thousands of people, we can capture far more of the variability that exists between people, observe patterns that would be impossible to see in smaller data, and identify variables that are either generalizable to all or that help us understand the differences between cases.
Reusing large datasets in combination with AI methods helps us make sense of these complexities and accelerate our ability to treat human diseases at an unprecedented rate. But to fully uncover the power of AI in medicine and biomedical research, institutions need to fully buy in to the new era of open data-sharing. Sharing data in a way that maximizes potential impact will require scientists to come together and establish field-wide standards and reporting procedures, providing common structure and a venue for easy data pooling and reusing. For example, choosing common platforms to store and share data that are accessible and searchable in the same format is far more efficient than every group uploading to their own website. Choosing a platform that can also issue digital object identifiers (DOIs) will transform datasets into citable objects and further incentivize researchers to share their data, knowing they will receive credit much like they do for paper citations.
Open science and data-sharing have come a long way, but we are still in the early days. Only a small fraction of the data produced each year are publicly available, representing a wasted opportunity to accelerate science. To succeed, the field will need to overcome cultural barriers to data-sharing, such as the long-held view of data as a raw material for producing publications in the “publish or perish” culture of academia. Scientists need to be trained on the necessary skills for this new era of big data and data-sharing. The field must overcome technical barriers to efficient research data collection, management and analysis, as well as the cost of efficient data-sharing and archiving, and administrative and legal hurdles to privacy and data ownership.
The good news is that the open-science movement and U.S. federal agencies are working together to provide funding for technology development, data skills training and community support and engagement. Beyond current mandates for data-sharing now imposed by federal agencies, other incentives for sharing data are emerging. For example, some research shows that people who share data are more widely recognized by their peers, with their work reaching a larger audience. And data-sharing through stable, federally supported repositories allows scientists to reuse their own data and continue to garner citations even years after the research has been conducted. Data-sharing is growing, and now is an excellent time to get on board and contribute to setting the rules.
This piece is part of an ongoing series exploring the benefits and challenges of data-sharing in neuroscience.
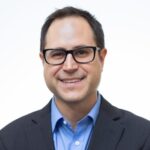
Brain and Spinal Injury Center (BASIC) University of California, San Francisco (UCSF)
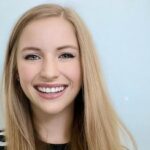
University of California, San Francisco (UCSF)
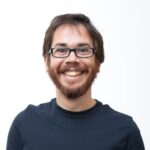
Brain and Spinal Injury Center (BASIC) University of California, San Francisco
Explore more from The Transmitter
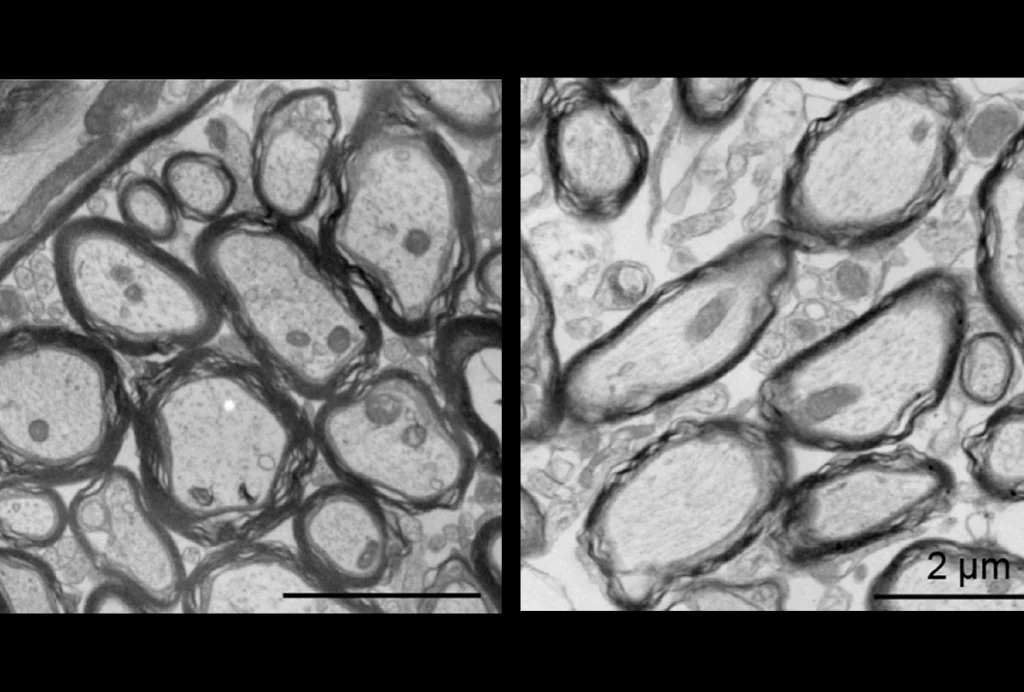
X chromosome inactivation; motor difficulties in 16p11.2 duplication and deletion; oligodendroglia
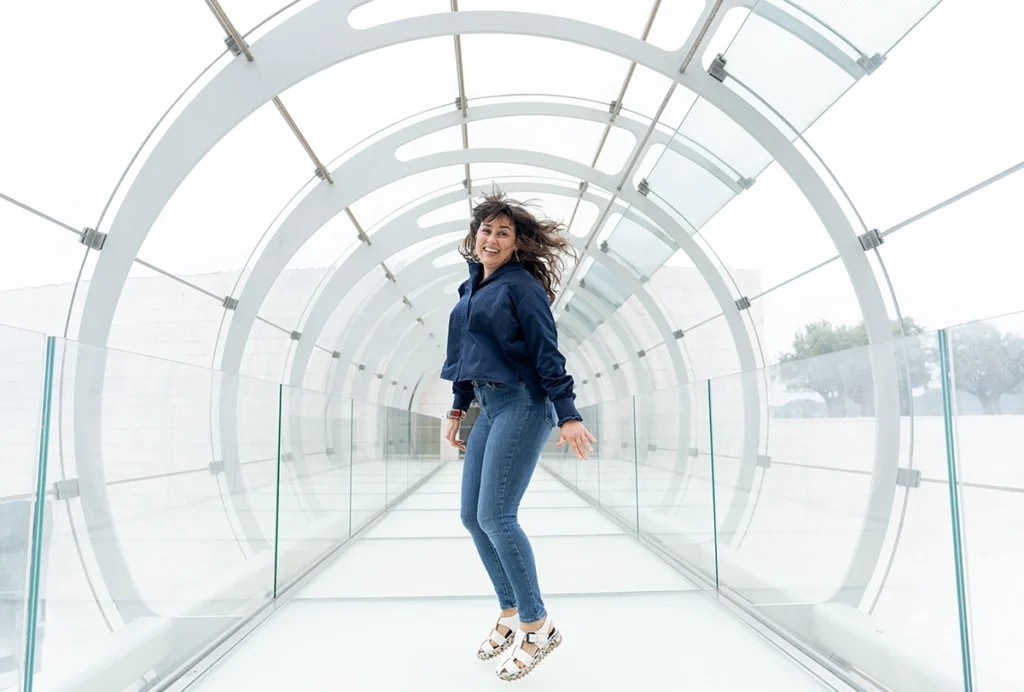
Decoding flies’ motor control with acrobat-scientist Eugenia Chiappe
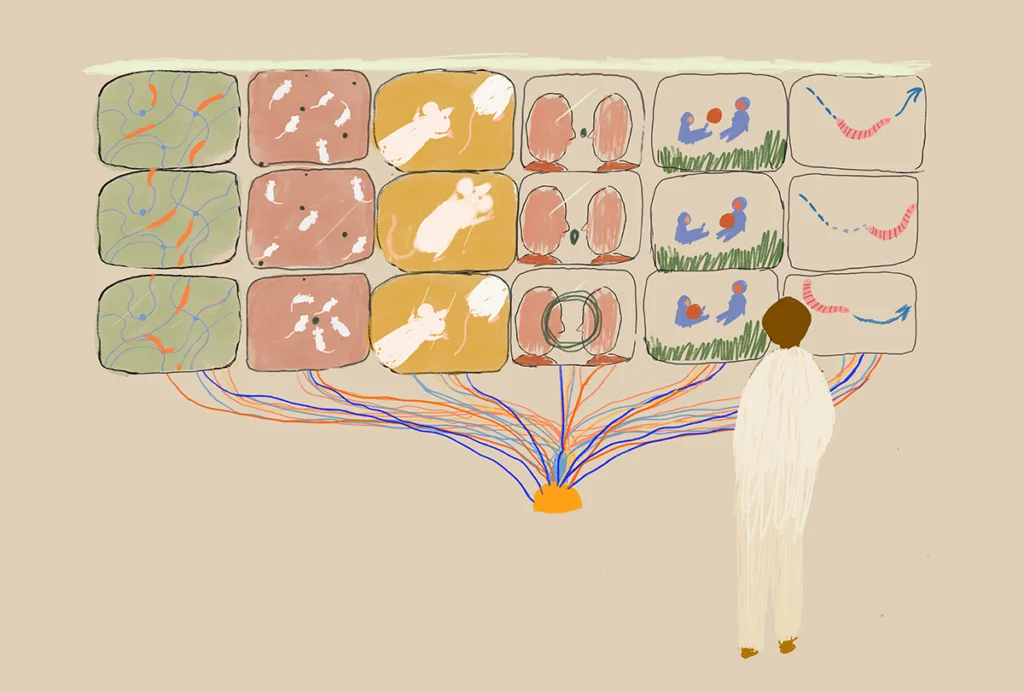