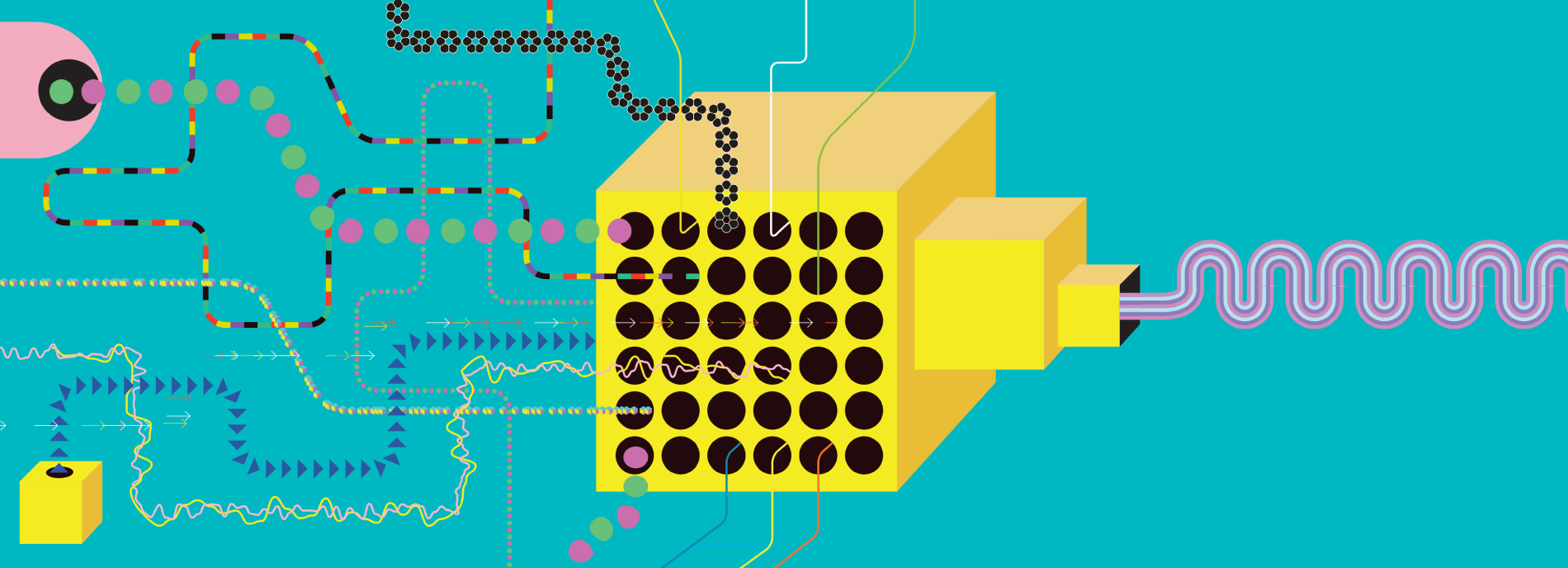
Can a computer diagnose autism?
Machine-learning holds the promise to help clinicians spot autism sooner, but technical and ethical obstacles remain.
Cet article est également disponible en françias.
M
artin Styner’s son Max was 6 by the time clinicians diagnosed him with autism. The previous year, Max’s kindergarten teacher had noticed some behavioral signs. For example, the little boy would immerse himself in books so completely that he shut out what was going on around him. But it wasn’t until Max started to ignore his teacher the following year that his parents enlisted the help of a child psychologist to evaluate him.Max is at the mild end of the spectrum. Even so, Styner, associate professor of psychiatry and computer science at the University of North Carolina at Chapel Hill, wondered if he had been fooling himself by not seeing the signs earlier. After all, Styner has studied autism for much of his career.
Given how complex and varied autism is, it’s not surprising that even experts like Styner don’t always recognize it right away. And even when they do spot the signs, getting an autism diagnosis takes time: Families must sometimes visit the nearest autism clinic for several face-to-face appointments. Not everyone has easy access to these clinics, and people may wait months for an appointment.
That reality has led to a detection gap: Although an accurate diagnosis can be made as early as 2 years of age, the average age of diagnosis in the United States is 4. And yet the earlier the diagnosis, the better the outcome.
Some researchers say delays in autism diagnosis could shrink with the rise of machine learning — a technology developed as part of artificial-intelligence research. In particular, they are pinning their hopes on the latest version of machine learning, known as deep learning. “Machine learning was always a part of the field,” Styner says, “but the methods and applications were never strong enough to actually have clinical impact; that changed with the onset of deep learning.”
Deep learning’s power comes from finding subtle patterns, among combinations of features, that might not seem relevant or obvious to the human eye. That means it’s well suited to making sense of autism’s heterogeneous nature, Styner says. Where human intuition and statistical analyses might search for a single, possibly nonexistent trait that consistently differentiates all children with autism from those without, deep-learning algorithms look instead for clusters of differences.
Still, these algorithms depend heavily on human input. To learn new tasks, they ‘train’ on datasets that typically include hundreds or thousands of ‘right’ and ‘wrong’ examples — say, a child smiling or not smiling — manually labeled by people. Through intensive training, though, deep-learning applications in other fields have eventually matched the accuracy of human experts. In some cases, they have ultimately done better.
“I think these approaches are going to be reliable, quantitative, scalable — and they’re just going to reveal new patterns and information about autism that I think we were just unaware of before,” says Geraldine Dawson, professor of psychiatry and behavioral sciences at Duke University in Durham, North Carolina. Not only will machine learning help clinicians screen children earlier, she says, but the algorithms might also offer clues about treatments.
Not everyone is bullish on the approach’s promise, however. Many experts note that there are technical and ethical obstacles these tools are unlikely to surmount any time soon. Deep learning — and machine learning, more broadly — is not a “magic wand,” says Shrikanth Narayanan, professor of electrical engineering and computer science at the University of Southern California in Los Angeles. When it comes to making a diagnosis and the chance that a computer might err, there are “profound implications,” he says, for children with autism and their families. But he shares the optimism many in the field express that the technique could pull together autism research on genetics, brain imaging and clinical observations. “Across the spectrum,” he says, “the potential is enormous.”
Bigger is better:
T
o make accurate predictions, machine-learning algorithms need vast amounts of training data. That requirement presents a serious challenge in autism research because most data relevant to diagnoses comes from painstaking — and therefore limited — clinical observations. Some researchers are starting to build larger datasets using mobile devices with cameras or wearable sensors to track behaviors and physiological signals, such as limb movements and gaze.In 2016, a European effort called the DE-ENIGMA project started building the first freely available, large-scale database based on the behaviors of 62 British and 66 Serbian children with autism. So far, this dataset includes 152 hours of video interactions between the children and either adults or robots. “One of the primary goals of the project is to create a database where you can train machine learning to recognize emotion and expression,” says Jie Shen, a computer scientist at Imperial College London and DE-ENIGMA’s machine-learning expert.
Dawson’s team at Duke is also collecting videos of children with autism via a mobile app developed for a project called Autism & Beyond. During the project’s initial year-long run in 2017, more than 1,700 families took part, uploading nearly 4,500 videos of their children’s behaviors and answering questionnaires. “We were getting in a year the amount of data that experts might get in a lifetime,” says Guillermo Sapiro, professor of electrical and computer engineering at Duke, who is working on the app’s next iteration.
The group is also training a deep-learning algorithm to interpret the actions in the video clips and detect specific behaviors — something Dawson describes as ‘digital phenotyping.’ At this year’s meeting of the International Society for Autism Research, Dawson presented results from a study of 104 toddlers, including 22 with autism, who watched a series of videos on a tablet. The tablet’s camera recorded the child’s facial expressions and head movements. The algorithm picked up on a two-second delay in the autistic children’s response to someone calling their name. Clinicians could easily miss this slight lag, an important red flag for autism, Dawson says.
One caveat to this kind of an approach is that collecting data outside the structured confines of a lab or doctor’s office can be messy. Sapiro says he was puzzled by the algorithm’s assessment of one participant in the Autism & Beyond project, who showed a mix of developmentally typical and atypical behaviors. When Sapiro watched the videos of that little girl, though, he quickly realized what was going on: Her behavior was typical during the day but atypical at night, when she was tired.
Researchers might be able to interpret these videos more readily by combining them with information from sensors capturing a child’s behavior. A group of scientists at the Georgia Institute of Technology in Atlanta is exploring this approach, which they describe as ‘behavioral imaging.’ One of the scientists, Gregory Abowd, has two sons on the spectrum. “My oldest is a non-speaking individual, and my younger one speaks but has difficulty with communicating effectively,” Abowd says. In 2002, three years after his oldest son was diagnosed with autism at age 2, he says, “I started to get interested in what I could do as a computer scientist to address any of the challenges related to autism.”
The Georgia Tech scientists are investigating sensors to track a range of physiological and behavioral data. In one project, they are using wearable accelerometers to monitor physical movements that could signify problem behaviors, such as self-injury. Another initiative involves glasses fitted with a camera located on the bridge of the nose to make it easier to follow a child’s gaze during play sessions.
The dream, says computer scientist James Rehg, is to train machine-learning algorithms to use these signals to automatically generate a snapshot of a child’s resulting social-communication skills. “I think it’s a really exciting time and an exciting area precisely because of the wealth of signals and different kinds of information that people are exploring,” says Rehg.
Comprehensive behavioral data could also yield clues about conditions that often co-occur with autism, says Helen Egger, chair of the child and adolescent psychiatry at NYU Langone Health in New York City and co-investigator on the Autism & Beyond project. Egger says larger datasets may help make sense of the overlap in behavioral traits between autism and conditions such as anxiety, attention deficit hyperactivity disorder, obsessive-compulsive disorder and depression. “We have to be able to use these tools with the full spectrum of children to differentiate children with autism from those without autism,” she says.
”Across the spectrum, the potential is enormous." Shrikanth Narayanan
The earliest signals:
S
ome research teams hope to train machine-learning models to detect signs of autism even before behavioral symptoms emerge.Styner and his colleagues in the Infant Brain Imaging Study (IBIS), a research network across four sites in the U.S., are using deep learning to analyze the brain scans of more than 300 baby siblings of children with autism. Because these ‘baby sibs’ are known to be at an increased risk of autism, it might be easier to spot signs of the condition in this group. In 2017, IBIS published two studies in which machine-learning algorithms picked up on certain patterns in brain growth and wiring and correctly predicted an autism diagnosis more than 80 percent of the time.
“One key difference between our studies and many machine-learning studies is that ours have been predicting later diagnostic outcome from a pre-symptomatic period,” says Joseph Piven, professor of psychiatry and director of the Carolina Institute for Developmental Disabilities at the University of North Carolina at Chapel Hill and an IBIS investigator. “That will clearly be useful clinically, if replicated.”
Machine learning trained on brain imaging might also provide more than a binary ‘yes’ or ‘no’ prediction about a diagnosis, Styner says. It could also forecast where that child falls along on the autism spectrum, from mild to severe. “That’s what we’re heading for, and I see in our research and in other people’s research that that’s definitely possible,” he says.
One factor limits the volume of brain-imaging data that can be collected: Participants have to find magnetic resonance imaging machines, which are bulky, expensive and tricky to use with children. A more flexible option for detecting early signs of autism may be electroencephalography (EEG), which monitors electrical activity in the brain via portable caps studded with sensors. “It was and still is the only brain measurement tech that can be used widely in clinical care practice,” says William Bosl, associate professor of health informatics, data science and clinical psychology at the University of San Francisco.
Machine-learning algorithms represent just the first part of the equation in working with EEG. The second involves what Bosl describes as the “secret sauce” — additional computer methods that remove noise from these signals and make it easier to detect patterns in the data. In a 2018 study, Bosl and his colleagues used this algorithmic mix to monitor the EEGs of 99 baby sibs and 89 low-risk infants for almost three years. Using EEG data from babies as young as 3 months, the method was able to predict severity scores on the gold-standard diagnostic test, the Autism Diagnostic Observation Schedule (ADOS).
Even when they are promising, algorithms reveal nothing about the biological significance of their predictive findings, researchers caution. “We don’t know what the computer is picking up in the EEG signal per se,” says Charles Nelson, director of research at Boston Children’s Hospital’s Developmental Medicine Center, who co-led the EEG work. “Maybe it is a good predictive biomarker, and as a result we can make a later prediction about a later outcome, but it doesn’t tell us why children develop autism.”
Like researchers working with brain imaging or behavioral data, those focused on EEG are also relying on relatively small datasets, which comes with complications. For example, sometimes an algorithm learns the patterns of a particular dataset so well that it cannot generalize what it has learned to larger, more complex datasets, Bosl says. This problem, called ‘overfitting,’ makes it especially important for other studies — ideally by independent teams — to validate results.
Another common pitfall arises when researchers use training datasets that contain an equal number of children with and without autism, Styner says. Autism is not present in half of all children; it’s closer to 1 in 60 children in the U.S. So when the algorithm moves from training data to the real world, its ‘needle-in-a-haystack’ problem — identifying children with autism — becomes far more challenging: Instead of finding 100 needles mixed in with 100 strands of hay, it must find 100 needles mixed in with 6,000 strands of hay.
Computer assist:
G
iven these challenges, many autism researchers remain hesitant about rushing to commercialize applications based on machine learning. But a few have more willingly engaged with startups — or launched their own — with the goal of bypassing the autism-screening bottleneck.Abowd has served as chief research officer for a Boise, Idaho-based company called Behavior Imaging since 2005, when Ron and Sharon Oberleitner founded the company, almost a decade after their son was diagnosed with autism at age 3. The company offers telemedicine solutions, such as the Naturalistic Observation Diagnostic Assessment app, which allows clinicians to make remote autism diagnoses based on uploaded home videos.
Behavior Imaging is partway through a study that aims to train machine-learning algorithms to characterize behaviors in videos of children. Once they identify the behaviors, they could draw clinicians’ attention to those key timestamps in the video and spare them from having to watch the video from start to finish. In turn, clinicians could improve the algorithm by either confirming or correcting its assessments of those moments. “This is going to be a clinical-decision-support tool that is going to constantly build up the industry expertise of what atypical behaviors of autism actually are,” Ron Oberleitner says.
A more ambitious vision for computer-assisted autism screening comes from Cognoa, a startup based in Palo Alto, California. The company offers a mobile app that provides risk assessments to parents based on roughly 25 multiple-choice questions and videos of their child’s activities. Ultimately, Cognoa’s leaders want U.S. Food and Drug Administration approval for an application that they say will empower pediatricians to diagnose autism and refer children directly for treatment.
Dennis Wall, now a researcher at Stanford University, founded Cognoa in 2013. After two studies published in 2012, he says, he became convinced that his machine-learning algorithms could be trained to make autism diagnoses more accurately and faster than two screening tools, the ADOS and the Autism Diagnostic Interview-Revised (ADI-R). “It was a solid step forward and provided a sound launch pad for future work,” Wall says.
But Wall’s 2012 papers didn’t convince everyone. Several critics, including Narayanan, pointed out in a 2015 analysis that the studies used small datasets and only considered children with severe autism, excluding the most complicated and difficult-to-diagnose forms of the condition. In the real world, they argued, his algorithms would miss many diagnoses a clinician would catch. Wall published a 2014 validation study that he says upheld the algorithm’s performance on an independent dataset, including data from children in the middle of the spectrum. He acknowledges that the 2012 studies used smaller datasets, but says the accuracy of his algorithms holds up in larger datasets used in later studies.
In 2016, Narayanan and some of his 2015 co-investigators described their own efforts to use machine learning to streamline autism screening and diagnosis. In their conclusion, they sounded a note of caution, saying that their algorithms, trained on responses from parents seeking a diagnosis for their child, also performed well but needed more testing in larger and more diverse populations. “I feel that there is a clear potential to fine-tune associated clinical-instrument algorithms with machine learning,” says co-investigator Daniel Bone, senior scientist at Yomdle, Inc., a technology startup based in Los Angeles and Washington, D.C. “However, I’ve not seen clear evidence yet — my own work included — that this approach is a monumental step beyond the traditional statistical methods that have been employed by researchers for decades.”
Merely amassing data to train machine-learning algorithms won’t necessarily help, says Bone’s collaborator, Catherine Lord, director of the Center for Autism and the Developing Brain in White Plains, New York, who developed the ADOS. Sometimes there are obvious but unacknowledged explanations for an algorithm’s apparent success, Lord adds. For example, boys are diagnosed with autism about four times more often than girls. A machine-learning study that appears to succeed in predicting the difference between people with and without autism may in fact be noticing nothing more than gender differences. Likewise, it might be picking up on differences in intelligence. “It isn’t the machine learning’s fault,” Lord says. “It’s the human reviewers and the general idea that if you have enough study subjects you can do anything.”
”“Just because it's couched in mathematics doesn’t mean it's more real.” Fred Shic
Are we there yet?
S
ome teams claim that machine learning can predict autism with accuracies well beyond 95 percent, but those rates are unlikely to hold up under more rigorous test conditions, researchers say. Until the algorithms are that good, they are nowhere near ready for clinical use — and they won’t get that good without more experienced diagnosticians helping to guide their development: It takes clinical expertise to recognize and avoid the more obvious pitfalls in interpreting the available data.“By and large, I think the biggest problem we have is people with data-mining expertise going to datasets they don’t comprehend, because they’re not being guided by a clinical perspective,” says Fred Shic, associate professor of pediatrics at the University of Washington in Seattle. “I think it’s really important to work together when extracting deep truths; we need people with understanding of all sides to sit together and work on it.” Journal editors, too, should find reviewers with machine-learning expertise to look over related autism studies, he says.
Shic is co-investigator on a project that has developed a tablet-based app called the Yale Adaptive Multimedia Screener, which uses video narration to walk parents through questions about their child’s behavior. “I think it has a lot of advantages,” he says, but adds, “I don’t want to oversell it because honestly there are so many ways you can go wrong with these things.” To learn more, he said, researchers need larger studies with long-term follow up.
Shic says he makes a habit of scrutinizing the methods other researchers use and also checks to see if they replicate their algorithm’s accuracy using an independent dataset. “Of course, we will see a lot of advances. We will also see a lot of snake oil,” he says. “So we’ve got to be vigilant and suspicious and critical like we’ve been about everything that comes up; just because it’s couched in mathematics doesn’t mean it’s more real.”
Mathematics will never solve the ethical problems that may come with using machine learning for autism diagnosis, others note. “I really don’t think we should put the power of diagnosis, even early diagnosis, in the hands of machines that would then relay the results from the machine to the family,” says Helen Tager-Flusberg, director of the Center for Autism Research Excellence at Boston University. “This is very profound moment in the life of a family when they are told that their child has the potential to have a potentially devastating neurodevelopmental disorder.”
Styner points to the chance of false positives, or instances when a computer might wrongly identify a child as having autism, as a reason to move slowly. “I actually think something like Cognoa would be doing harm — significant harm — if it incorrectly predicts that the kid would have autism when [he or she] does not,” he says. “Unless you have a rock-solid prediction, I can’t see how this is not something unethical to a certain degree because of those false positives.”
In Styner’s own family, things turned out better than he might have predicted. His son Max, now 11, is academically gifted and benefits from a social-skills class and weekly play group. He is doing so well, in fact, that he may no longer meet the threshold for an autism diagnosis, Styner says.
Given his experience as a parent, though, he understands why families are so eager for earlier screening and diagnosis — and it still motivates him in his efforts to hone the potential of machine learning. “I can really empathize with families and their interest in knowing not just the diagnosis, but also what to expect with respect to severity of symptoms,” he says. “I certainly would have wanted to know.”
Corrections
This article has been modified from the original. A previous version incorrectly referred to Dennis Wall’s 2014 validation study as including an expanded test set rather than an independent dataset. Also, the Autism & Beyond project at Duke University collected nearly 4,500 videos, not 6,000.

Syndication
This article was republished in The Atlantic.
Recommended reading
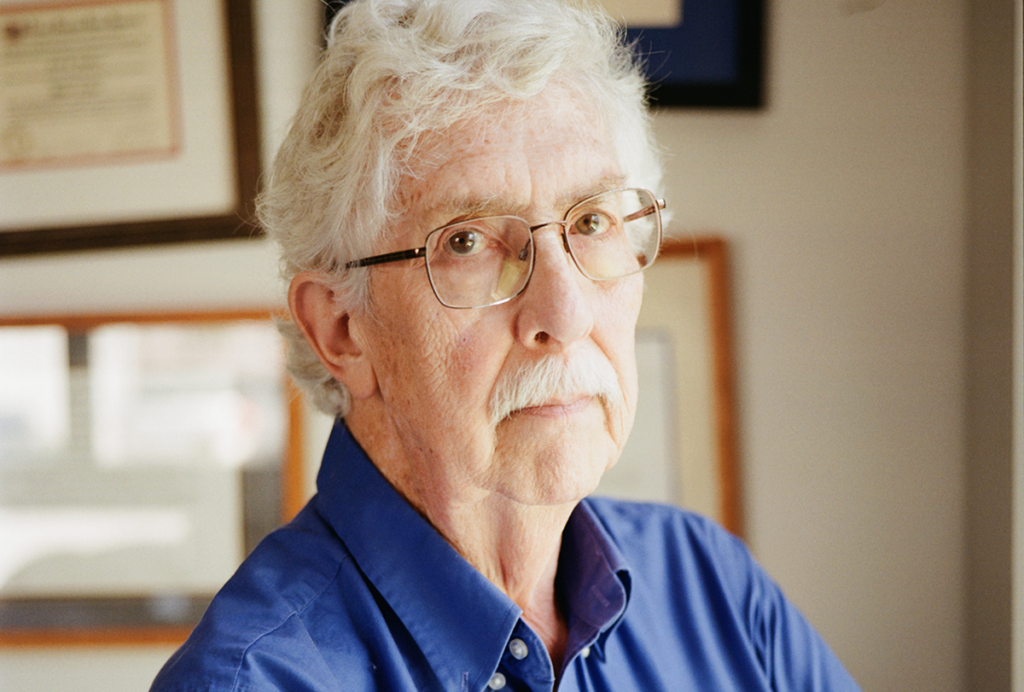
How pragmatism and passion drive Fred Volkmar—even after retirement
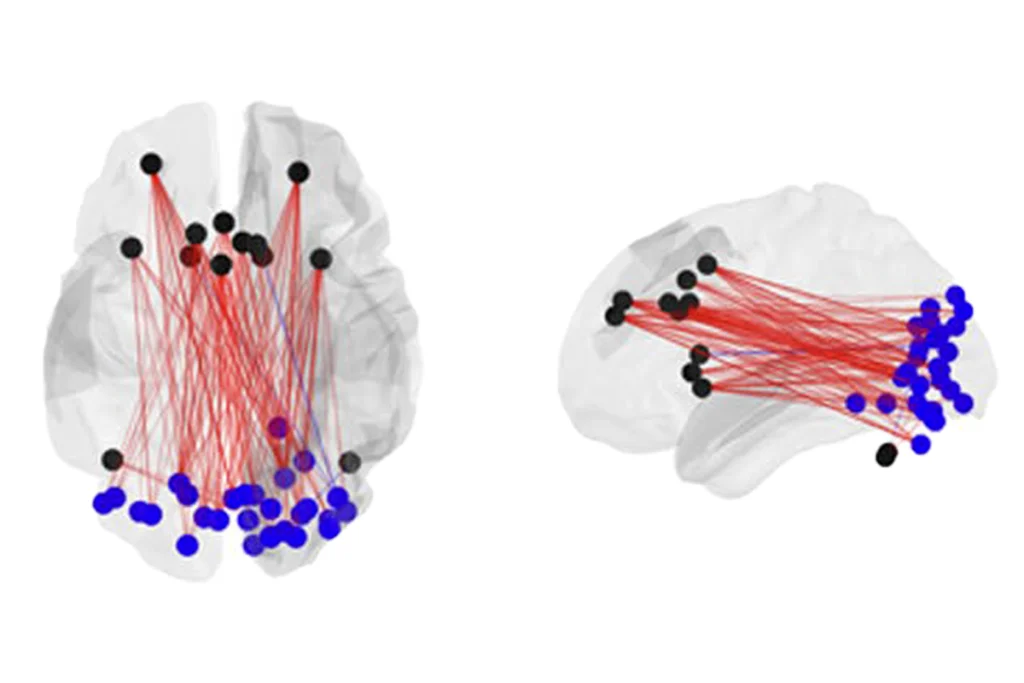
Altered translation in SYNGAP1-deficient mice; and more
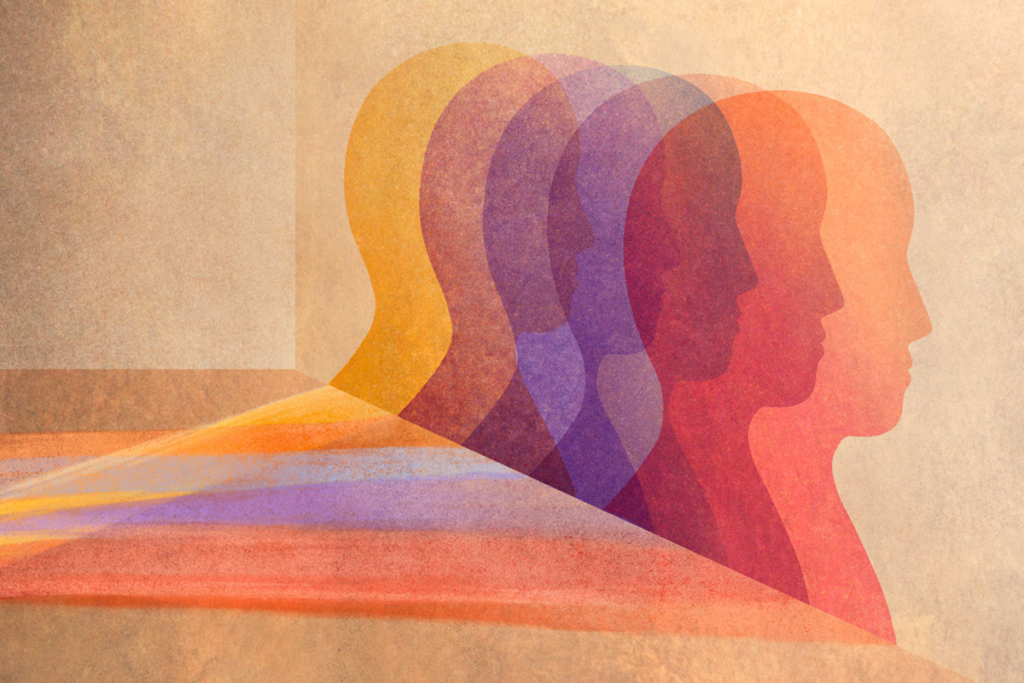
CDC autism prevalence numbers warrant attention—but not in the way RFK Jr. proposes
Explore more from The Transmitter
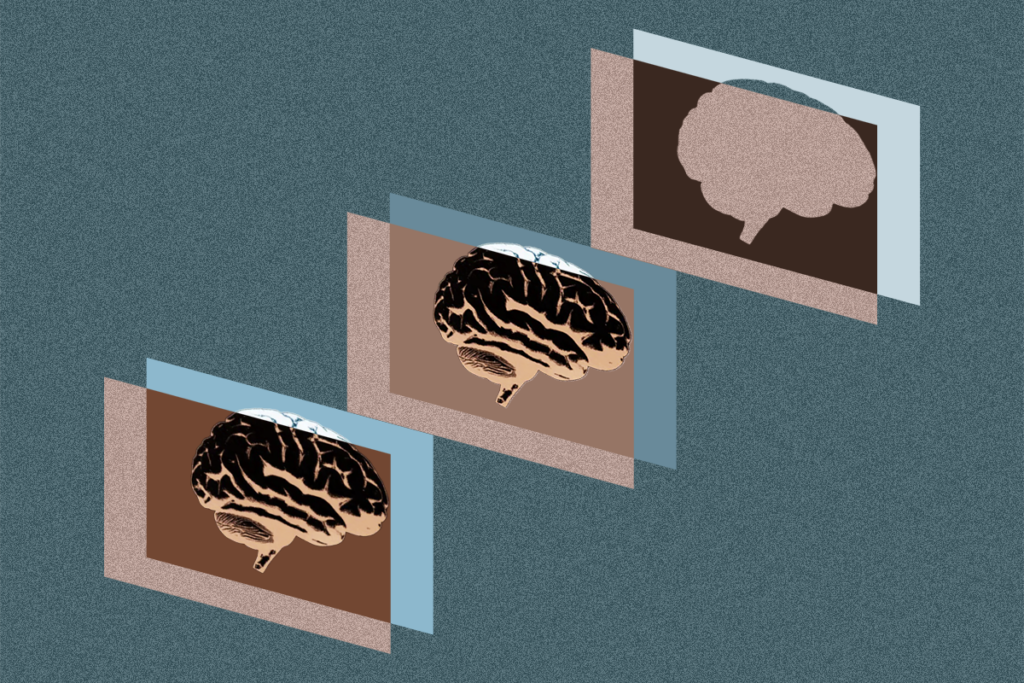