Fixing motion
New methods to deal with motion artifacts in brain imaging data are critically important, says Mike Tyszka.
There’s been a lot of discussion in the last year about the potential impact of head movement on functional magnetic resonance imaging (fMRI) studies. Even tiny differences in how much participants move during a scan can color a comparison between individuals with a disorder and controls.
In an article published in February, my colleagues and I were surprised to find that if we group participants by how much they moved while in the scanner, the effect on brain connectivity is far more pronounced than any of the differences we see when we group them by diagnosis1.
People in the brain imaging community are well aware of this issue and are coming up with different ways to mitigate it. But even the most rigorously designed, tested and validated motion correction leaves its mark on the data — subtle footprints that can be difficult to detect.
A highly influential article published last year showed quite convincingly that connectivity analyses of human brain activity can be biased by residual motion, even following correction2. The researchers’ solution is to eliminate or ‘scrub’ time points in the MRI data at which participants moved the most.
But are there alternatives to simply removing data points? Everyone moves a little in the scanner — but how much is too much? The answer to this question is still relatively open, but papers published over the last few months suggest we may be closing in on some answers.
Vince Calhoun and his collaborators at the University of New Mexico have developed an approach that allows direct measurement of motion’s effect on measured brain activity3. This in turn helps identify and compensate for problem data without excluding the data from analysis.
Kevin Murphy’s team at Cardiff University in the U.K. takes yet another direction to handling physiological and gross motion in resting-state fMRI4.
They manage to squeeze an additional image out of previously unused periods of time in the scan. This allows researchers to directly estimate motion effects and remove them more accurately than by using conventional motion analysis, which is typically performed offline after the scan has finished. Better yet, the additional image is essentially ‘free,’ meaning it hardly increases the overall scan time.
Finally, in a great example of the type of large study made possible by multicenter image collections, Damien Fair and his collaborators at Oregon Health Science University in Portland explored not only the impact of sub-millimeter head motion (‘micro-movements’) on resting-state fMRI data, but also nine procedures for accounting for this motion, including traditional regression of MRI signal with motion parameters and removal of time points associated with excessive movement (scrubbing)5.
Fair and his colleagues analyzed brain scans from more than 450 typically developing children (aged 7 to 14 years) and 193 children with attention deficit hyperactivity disorder (ADHD), making this one of the larger reviews of this type of data in the literature.
Once they had accounted for the subtle effects of motion on the connectivity data, the researchers were able to distinguish between the two most prominent ADHD variants, namely Combined and Inattentive.
Although none of these publications offer the final word on accounting for the motion artifact, they demonstrate the type of attention to detail that’s critically important if we are to hold our fMRI connectivity analyses to the highest standards.
J. Mike Tyszka is associate director of the Caltech Brain Imaging Center. Read more from the special report on connectivity »
References:
1: Tyszka J.M. et al. Cereb. Cortex Epub ahead of print (2013) PubMed
2: Power J.D. et al. Neuroimage 59, 2142-2154 (2012) PubMed
3: Christodoulou A.G. et al. Magn. Reson. Imaging Epub ahead of print (2013) PubMed
4: Bright M.G. and K. Murphy Neuroimage 64, 526-537 (2013) PubMed
5: Fair D.A. et al. Front. Syst. Neurosci. Epub ahead of print (2013) PubMed
Recommended reading
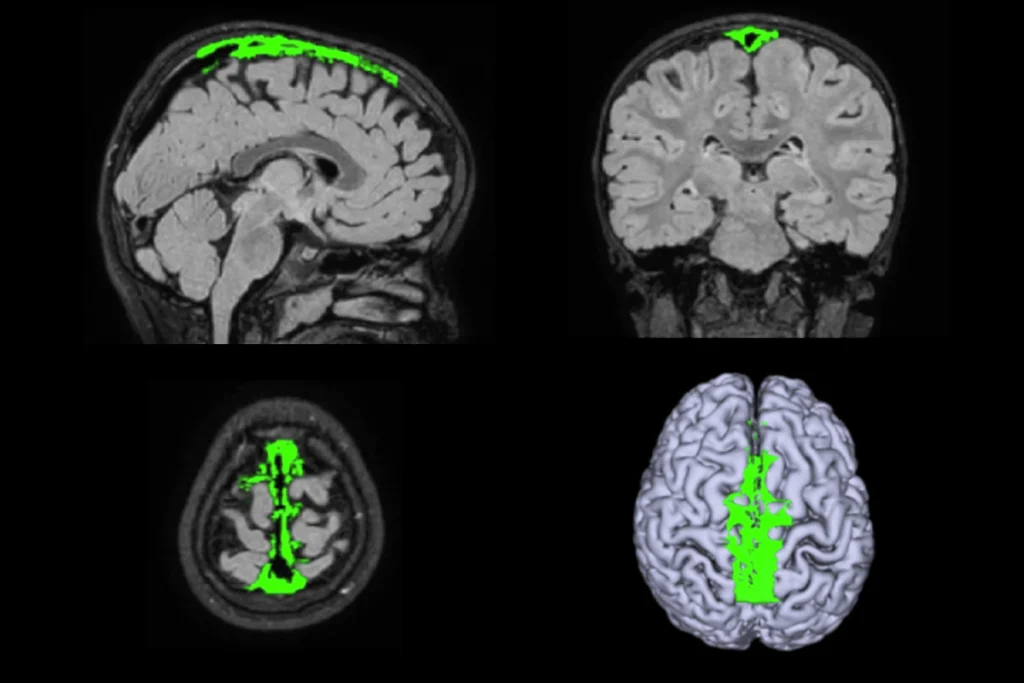
Okur-Chung neurodevelopmental syndrome; excess CSF; autistic girls
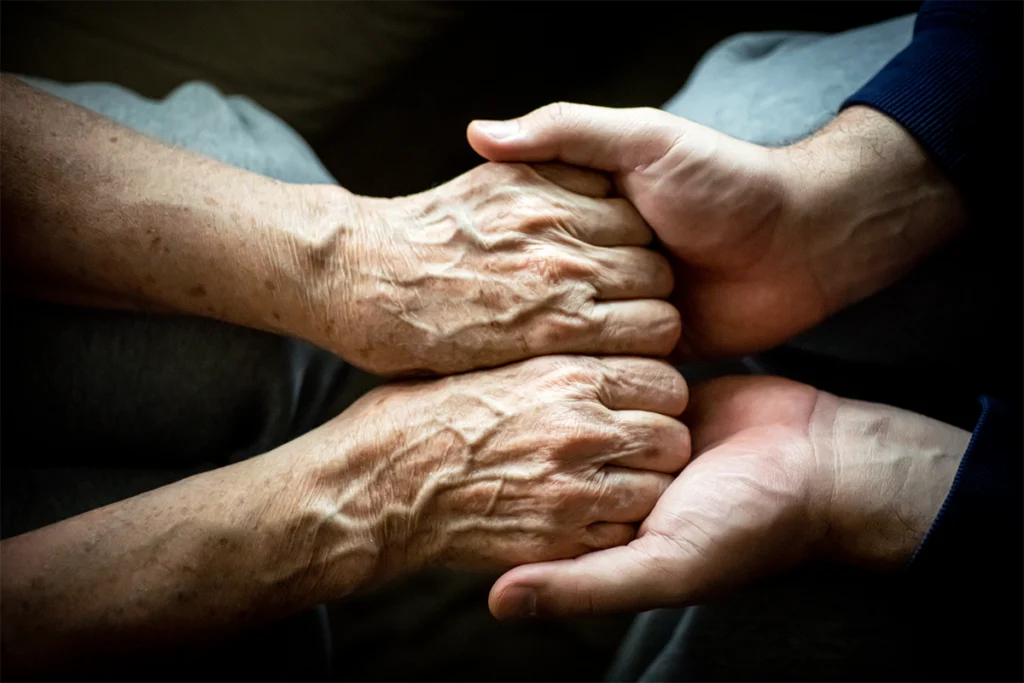
New catalog charts familial ties from autism to 90 other conditions
Explore more from The Transmitter
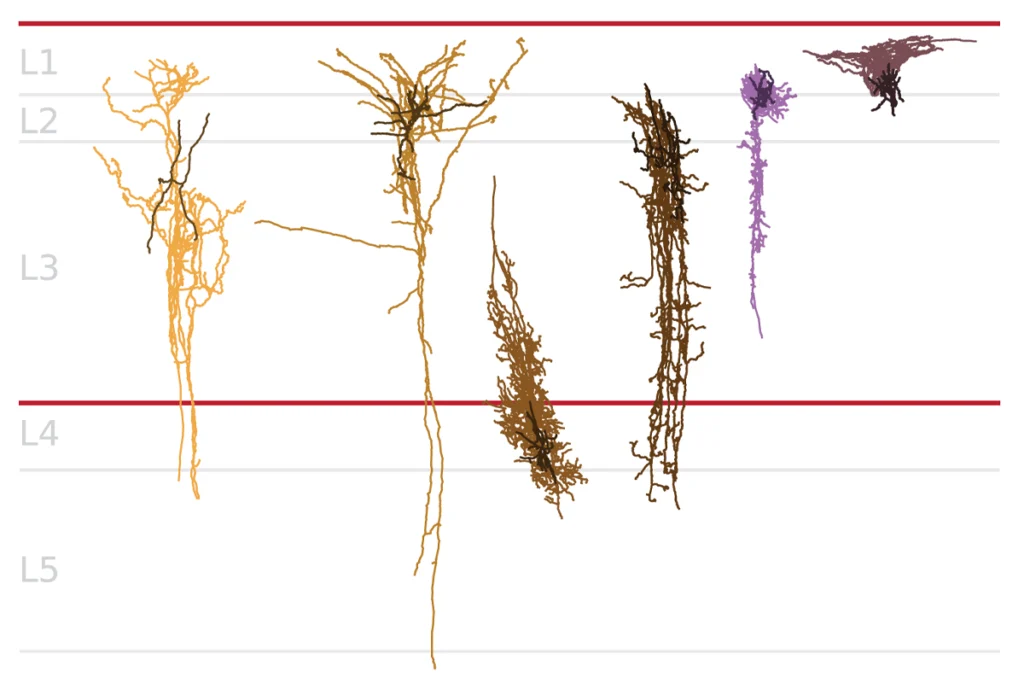