Great sequencing power — great responsibility
Chris Gunter and Daniel MacArthur discuss guidelines for assessing the evidence that a genetic variant causes autism or another disorder.
The increasingly widespread availability of DNA sequencing technologies has accelerated reports of genetic variants ‘causing’ diseases. Without clear statistical and experimental guidelines for determining the causality of these variants, however, researchers and clinicians run the risk of making incorrect diagnoses and treatment recommendations.
In a report published 24 April in Nature we presented the consensus statement from a workshop sponsored by the National Human Genome Research Institute1. The workshop’s participants —27 geneticists and other researchers from 31 institutions worldwide — agreed upon guidelines for assessing the evidence that a genetic variant causes autism or another disorder.
There is a clear need for more statistically rigorous approaches to inferring cause by variants, and for enhanced data-sharing. We were inspired to create this working group after seeing multiple cases of false-positive reports linking genetic variants to disease.
For example, a 2011 study examined 406 separate reports of ‘severe disease mutations’ observed in 104 newly sequenced individuals2. The study reported that 122 (27 percent) of these mutations are in fact either common variants — or polymorphisms — or that there is no direct evidence for their disease-causing potential.
False-positive reports such as these can have serious consequences, including incorrect diagnoses, unnecessary or ineffective treatments and misinformed reproductive decisions (such as embryo termination).
We argue for a careful two-stage approach for assessing the evidence for the degree to which a specific variant contributes to disease.
Careful steps:
The first step involves ‘gene-level implication,’ or evaluating how much overall support there is that the affected gene causes the set of observed characteristics of the disease or disorder. Supporting evidence could be data from animal models or gene expression patterns, or genetic information such as the number of mutations expected to be seen by chance in this gene, given the study design.
Next, we suggest ‘variant-level implication,’ or an assessment of the probability that the variants carried by an individual do indeed play a causal role in that person’s disorder. Here, the data can be genetic, experimental or bioinformatic, such as a variant’s score from algorithms that calculate the conservation of variants through evolution.
With these guidelines, we propose a process for integrating multiple types of evidence. Our working group strongly feels that for detecting pathogenic variants, researchers must use proper statistical and quantitative frameworks to evaluate the observed variation in a gene, compared with a carefully chosen null model specific to the hypothesis being considered.
One example comes from the field of autism, in which a team of researchers used an appropriate statistical model and avoided mistakenly implicating a gene found by sequencing studies.
In 2012 and 2013, four studies reported results from sequencing the exomes — the protein-coding portions of the genome — of 945 families with a child who has autism3, 4, 5, 6. The studies found four independent de novo, or spontaneously occurring, mutations in the gene TTN. Without applying appropriate statistical models, the researchers could easily have suggested that TTN was worthy of further investigation.
However, they did not consider TTN to have a causal role in autism because they knew TTN has the largest coding sequence of any gene in the genome (hence the name of its encoded protein, TITIN). Their observation of four de novo TTN mutations was not significantly different from the number that would be predicted by chance.
We are fortunate to be part of a genomics community with a tradition of open discussion for proposing solutions to dilemmas created by advances in technology. In keeping with that tradition, we are encouraged by the fact that a diverse group of researchers was able to reach consensus across a wide range of issues in this report.
In fact, this entire effort began as a conversation between the two of us on Twitter, and quickly spread to involve other researchers who we knew shared similar concerns. We hope this paper is a starting point for a far broader conversation.
Chris Gunter is associate professor of pediatrics at Emory University School of Medicine in Atlanta. Daniel MacArthur is assistant professor of medicineat Harvard Medical School.
References:
1:MacArthur D.G. et al. Nature 508, 469-476 (2014) PubMed
2: Bell C.J. et al. Sci. Transl. Med. 3, 65ra4 (2011) PubMed
3: Sanders S.J. et al. Nature 485, 237-241 (2012) PubMed
4: O’Roak B.J. et al. Nature 485, 246-250 (2012) PubMed
5: Neale B.M. et al. Nature 485, 242-245 (2012) PubMed
6: Iossifov I. et al. Neuron 74, 285-299 (2012) PubMed
Recommended reading
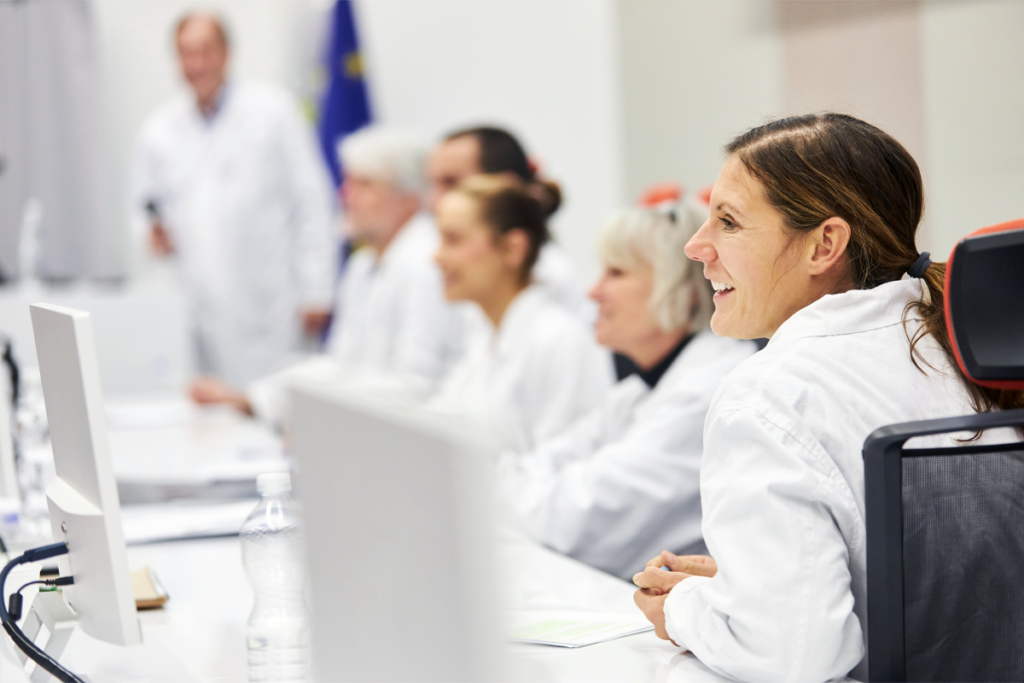
INSAR takes ‘intentional break’ from annual summer webinar series
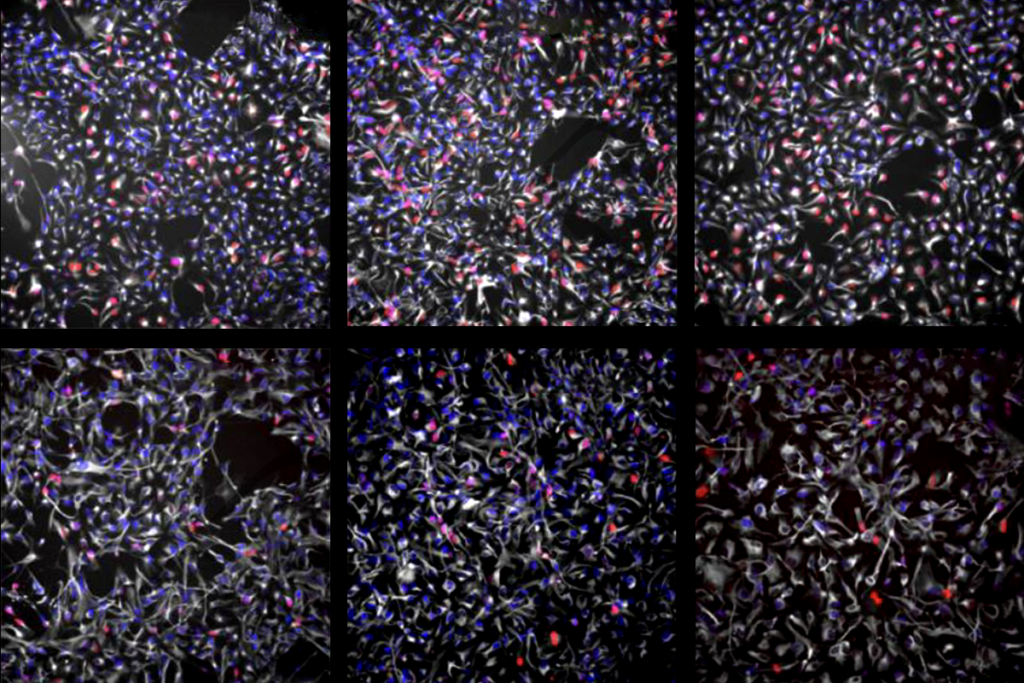
Dosage of X or Y chromosome relates to distinct outcomes; and more
Explore more from The Transmitter
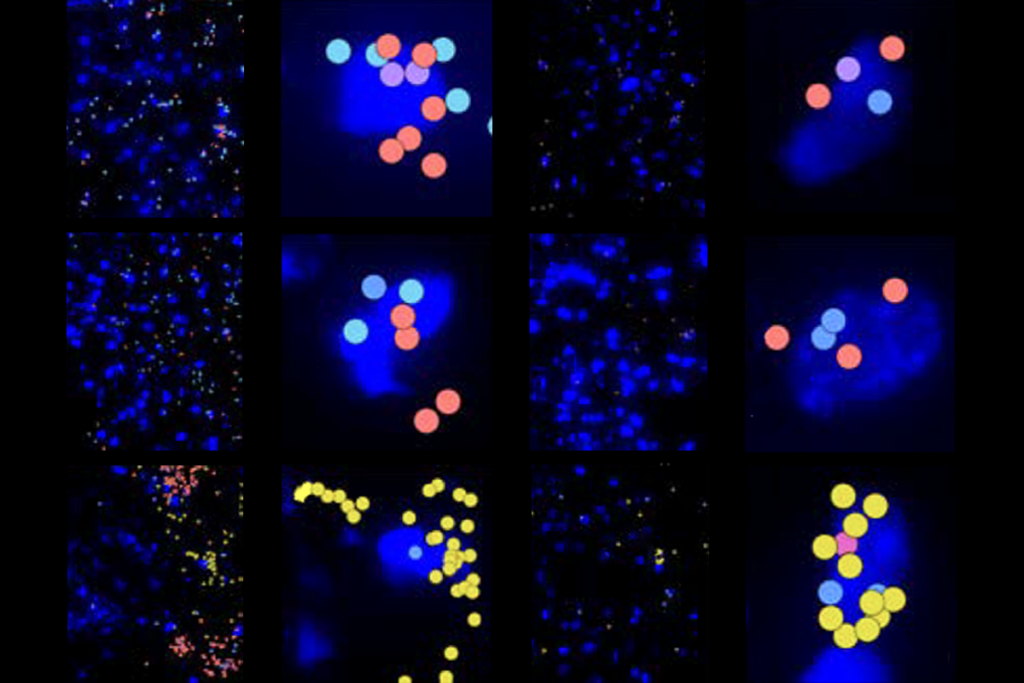
Machine learning spots neural progenitors in adult human brains
Xiao-Jing Wang outlines the future of theoretical neuroscience
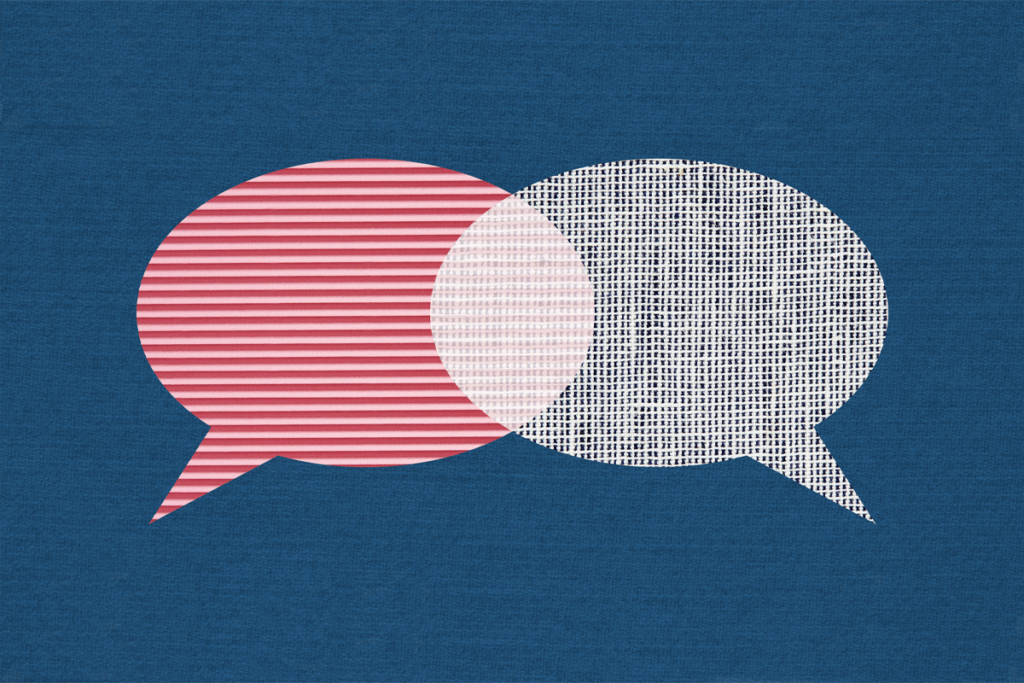