
‘Smart’ system efficiently tracks mouse behavior
A sophisticated new system with two video cameras and a depth sensor helps scientists quantify social behavior in mice.
A new system uses two video cameras and a depth sensor to track mice as they interact in a cage1. The sophisticated setup is especially useful for autism researchers exploring social behavior in mice.
“We had no way up until now to automatically quantify social behaviors, particularly those that occur when animals are in close proximity to each other, like mating, fighting, sniffing and social investigation,” says lead researcher David J. Anderson, professor of biology at Caltech University in Pasadena, California.
Most efforts to measure mouse behavior rely on a single video camera that produces two-dimensional images. The lack of depth perception makes it difficult to distinguish between two mice when they come into close contact. Experiments designed to assess social behavior also typically involve a researcher handling the mice. This intrusion can introduce anxiety and other variables that may alter the mice’s behavior.
To solve the perspective problem, Anderson and his colleagues used two strategically placed video cameras: One overlooks the top of the cage and the other is aimed at the front. They also developed software to integrate information from the cameras with that from an infrared depth sensor.
Together, the three readings can distinguish between two mice even when one climbs on top of the other. The researchers described the results 9 September in the Proceedings of the National Academy of Sciences.
The new system allows researchers to evaluate mice as they interact freely in a cage, without causing the mice to become anxious. Watching and annotating an hour of footage can take a person up to six hours. The new setup comes with software that can automatically identify mouse behaviors from the footage, saving valuable research hours.
Infinite patience:
The researchers taught the system to extract 27 features, such as the direction the mice face, their speed and their body position. The researchers then trained the algorithm with 150,000 frames of footage of two mice fighting, mating or involved in ‘close investigation,’ in which one animal examines the body of the other.
Based on this information, the software ‘learns’ which visual features are characteristic of specific behaviors. For example, a fight between two feisty mice might look like rapid chaotic movement that changes the luminance of video pixels rapidly from frame to frame.
The method accurately identified a mouse attacking or mounting its cage-mate 99 percent of the time, as compared against researchers’ annotations. For close investigation — a more subtle form of interaction — it was accurate 92 percent of the time.
The software is likely to catch mouse activities that people miss, says Pietro Perona, professor of electrical engineering and computation and neural systems at Caltech, who developed the expert system. (Perona co-led the study with Anderson.)
“Humans miss a lot because they lose patience and they skip forward,” he says. “The machine has infinite patience, so in the long term, it will be better than humans.”
An automated system can also classify mouse behaviors more consistently than people can. Researchers may disagree on what constitutes a certain interaction — for example, how long mice need to follow each other for it to be a ‘chase.’ The new system relies on standardized assessments that hold up across laboratories. It also allows researchers to easily reanalyze data if their understanding of a behavior changes.
The new approach is “elegant,” but training the software and learning how to use it can be time-consuming, says Jacqueline Crawley, professor of psychiatry at the University of California, Davis, who was not involved in the study. “Conducting the data analyses and learning how to fully utilize the software may take considerably longer than scoring videos,” she says.
On videos of BTBR mice — a naturally occurring strain of mice that are unusually antisocial — the system performed as expected. It indicated that these mice are less likely to approach and initiate contact with other mice than controls are.
Social subtleties:
So far, however, the system has only assessed interactions between mice that have different coat colors, which allows the cameras to distinguish between them. This is a limitation for autism research, experts say, in which researchers compare mice with a mutation to otherwise identical controls. Perona says researchers could add identifying marks to the mice.
The automated system may miss some social nuances that people pick up on, notes Mu Yang, assistant professor of psychiatry and behavioral sciences at the University of California, Davis, who was not involved in the work.
In pups, which don’t show aggression or mating behaviors, most interactions resemble ‘close investigation’ but have a range of meanings. For instance, a nose-to-nose sniff is like a gentle ‘hello,’ she says, whereas one mouse pushing its snout under another mouse is akin to “boys elbowing each other on the basketball court.”
To resolve interactions at this level of detail, the system would need to recognize thousands of subtle features, something that is not yet practical, says Perona.
For now, he and his colleagues are working to integrate the system with methods that allow researchers to peer into — and even control — the brains of freely moving mice, using techniques such as optogenetics. If the researchers succeed, they will be able to easily match up the inner workings of a mouse brain with the rodent’s choices.
References:
- Hong W. et al. Proc. Natl. Acad. Sci. USA 112, e5351-5360 (2015) PubMed
Recommended reading
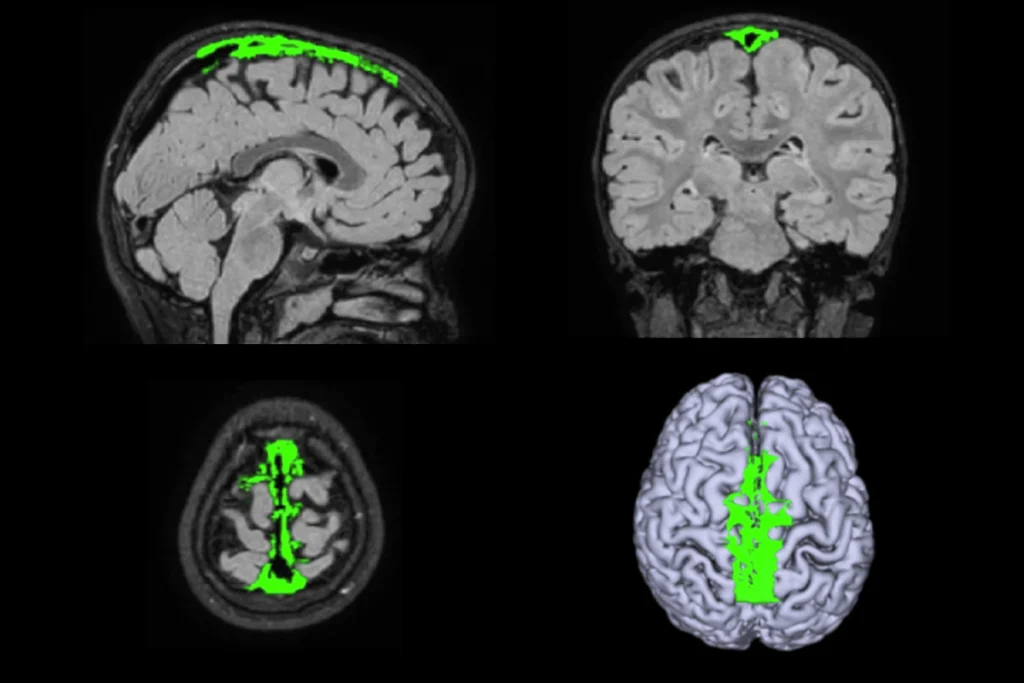
Okur-Chung neurodevelopmental syndrome; excess CSF; autistic girls
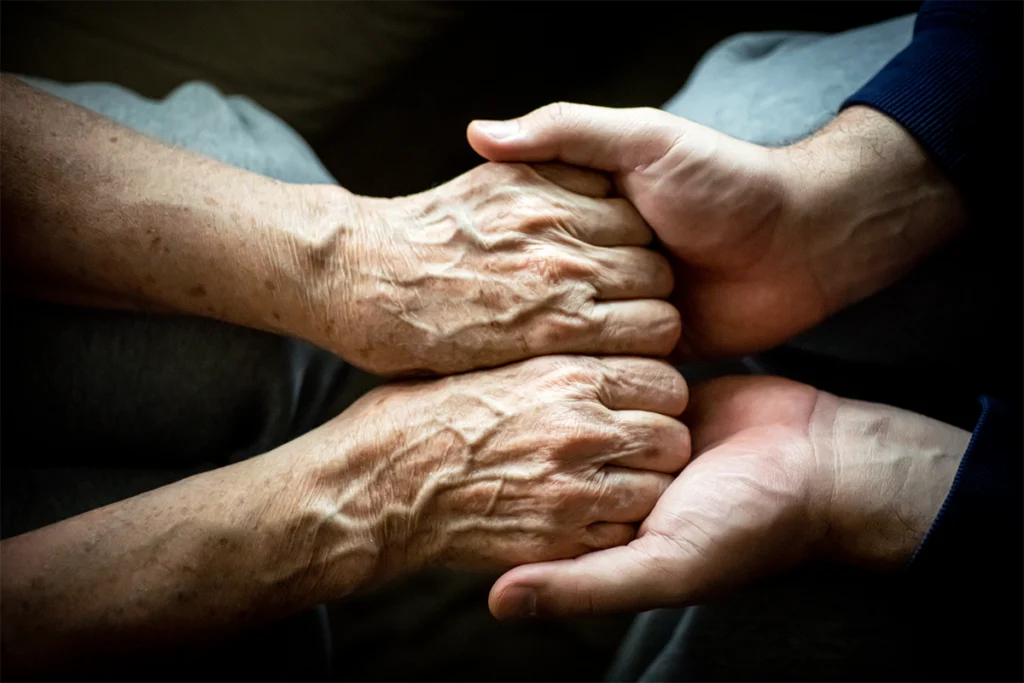
New catalog charts familial ties from autism to 90 other conditions
Explore more from The Transmitter
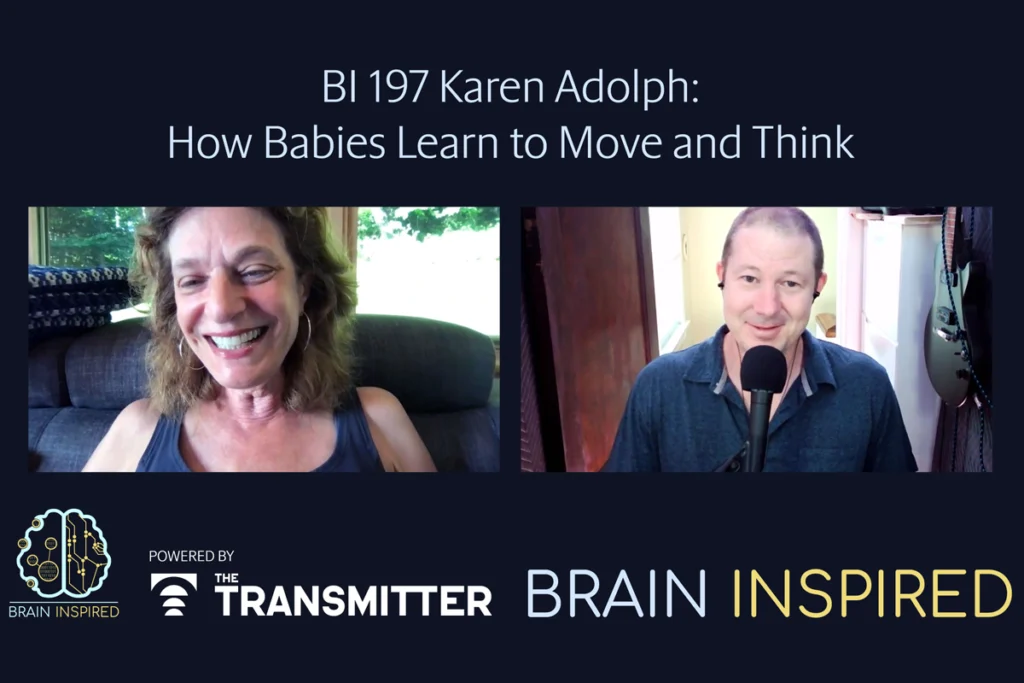
Karen Adolph explains how we develop our ability to move through the world
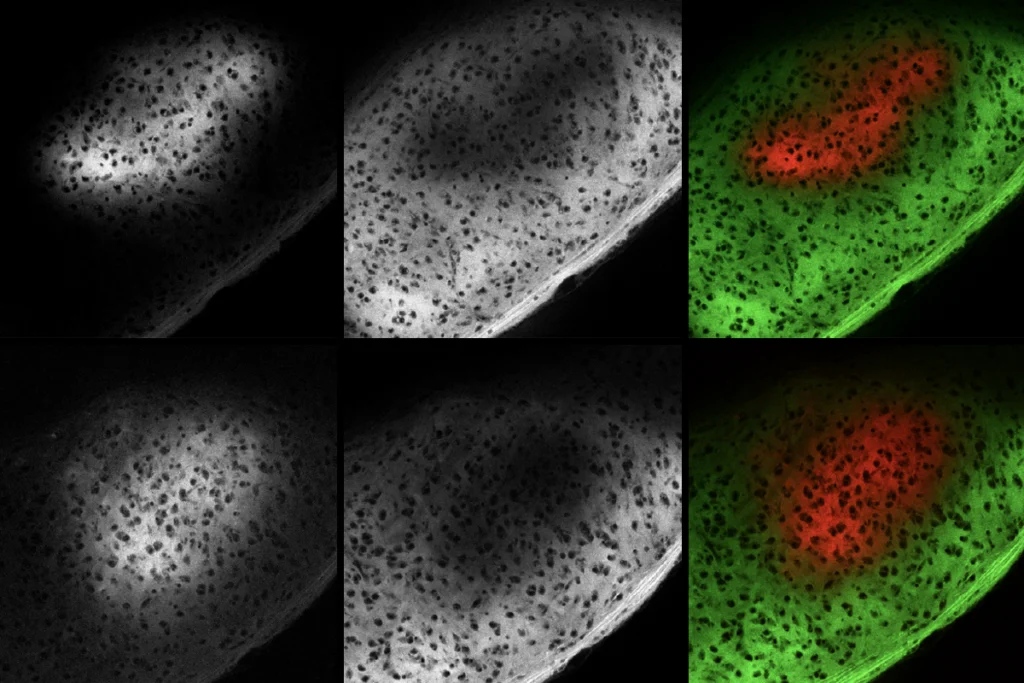
Microglia’s pruning function called into question
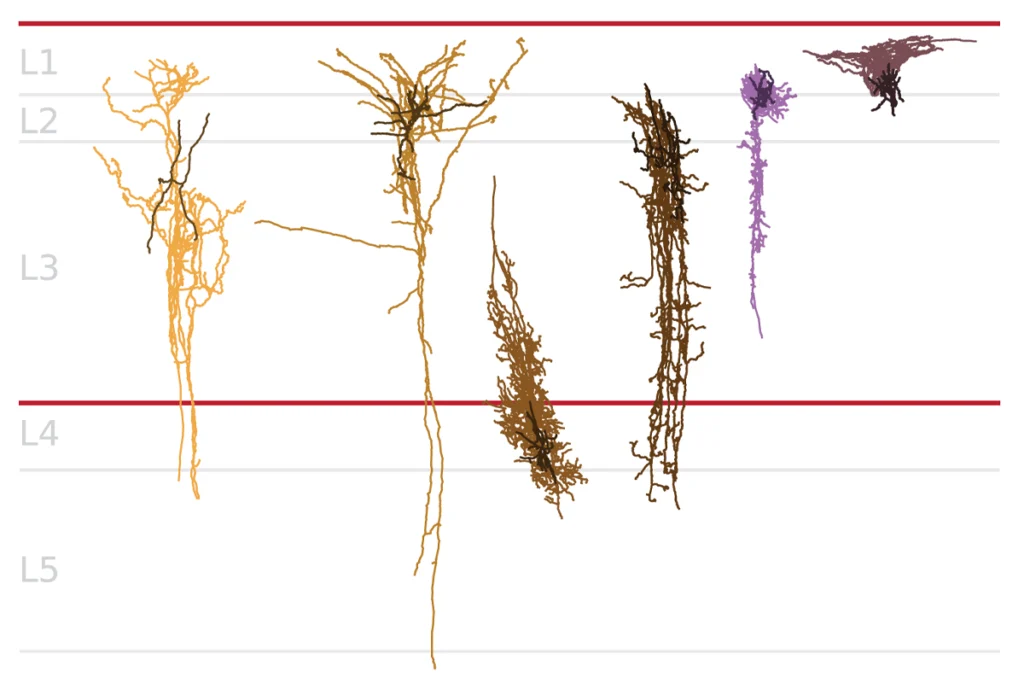