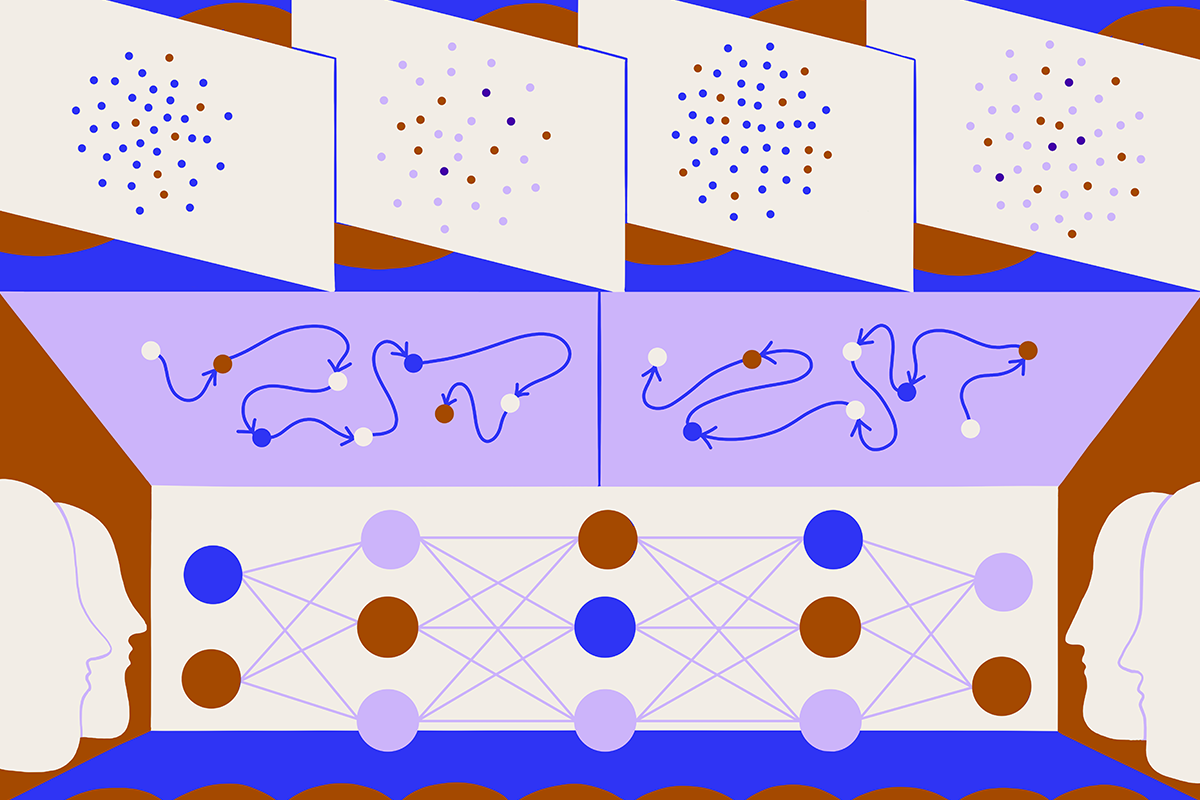
This paper changed my life: Marino Pagan recalls a decision-making study from four titans in the field
Valerio Mante and David Sussillo, along with their mentors Krishna Shenoy and Bill Newsome, revealed the complexity of neural population dynamics and the power of recurrent neural networks.
Answers have been edited for length and clarity.
What paper changed your life?
Context-dependent computation by recurrent dynamics in prefrontal cortex. Mante V., Sussillo D., Shenoy K.V. and Newsome W.T. Nature (2013)
In this paper, Valerio Mante, David Sussillo, Krishna Shenoy and Bill Newsome—four titans of the field of systems and computational neuroscience—investigated the neural mechanisms underlying cognitive flexibility and decision-making in macaque monkeys. This is one of the most important papers to come out in systems and computational neuroscience in the past 10 to 20 years.
They used a random dot motion task in which monkeys are shown a field of moving dots and have to accumulate evidence to decide the primary direction of motion. This paper adds a twist by incorporating a second feature to the dots: color. In some trials, the researchers asked the monkeys to determine the prevalent motion of the dots, and in other trials, they asked the animals to identify the predominant color of the moving dots. This required the monkeys to pay attention to the relevant feature and ignore the irrelevant one. Adding this element grounded the study of decision-making into a much more realistic regime. Just like in real life, you have a lot of things going on, and you have to figure out which ones matter.
The team found that decision-making in the monkey prefrontal cortex can be understood in terms of a dynamical system, where the neural population response drives the monkey toward one decision or another, depending on the task. By comparing these neural responses with a recurrent neural network, the researchers identified a novel mechanism underlying the selection and integration of sensory inputs.
When did you first encounter this paper?
I was finishing my Ph.D. in the lab of Nicole Rust at the University of Pennsylvania. At the time, I was training monkeys, so any paper that trained monkeys on hard tasks was definitely something that would draw my attention.
Why is this paper meaningful to you?
What’s remarkable to me about this paper is that it introduced a lot of revolutionary methods to attack the hard problem of how to interpret neural activity data recorded from many neurons during a complex task. The key idea was to study the dynamics of the population activity as a whole, rather than simply a collection of individual neurons, by analyzing the population trajectory as a vector in a high-dimensional space, where each axis represents the firing rate of one neuron. These trajectories can then be projected down to a low-dimensional space where they can be readily visualized and interpreted—a method first introduced with great success by Krishna Shenoy and others to study the motor cortex and movement. Here, instead, this approach was used to study cognition, providing a new, powerful tool to map the decision-making process on the neural population responses.
The other crucial innovation was to use recurrent neural networks (RNNs) to model brain computation. Traditionally, neural network models were often “hand-tuned” to reproduce the results observed in biological brains. In this case, the authors took a completely different approach by training RNNs to solve the same task as the monkeys, without imposing any constraints on the mechanisms these artificial networks would use. They then “opened up” the RNNs to reveal novel insights about how this computation could be performed by a neural network. This is now a standard approach used to generate hypotheses about computation mechanisms that are not bound by the imagination of the experimenter.
How did this paper change how you think about neuroscience or challenge your previous assumptions?
This paper dispelled the intuition at the time for how the brain was solving these kinds of tasks, which was based on the concept of “feature attention.” In the case of visual attention, for instance, imagine you’re playing “Where’s Waldo?” The idea was that your visual system is going to enhance anything that looks like a stripy hat because you are looking for that stimulus. You would expect that neurons that encode stimuli for a stripy hat are going to amplify their firing rate, and neurons that encode other things are going to have their firing rate go down.
That is not at all what the researchers found. Instead, they found that the amplitude of the neural signal in the macaque prefrontal cortex in response to relevant and irrelevant inputs was about equally strong. This means that the brain does not simply suppress the activity of neurons encoding irrelevant information—for example, silencing neurons encoding “color” during the “motion” task. Instead, they established that changes in neural population dynamics—rather than modulation of signal amplitude—are what control decision-making during these tasks.
How did this paper influence your career path?
This paper couldn’t have had a stronger impact on my career. After finishing my Ph.D., I joined the lab of Carlos Brody at Princeton University. There, I wanted to try to train rats on a task that was analogous to the one used in this paper. We decided on an auditory task because it is an easier modality to use in rats compared with vision, swapping sound location for random dot motion and auditory tone frequency for color. We were able to succeed at the daunting task of training rats to solve such a complex task thanks in part to advice from the authors, who we interacted with at meetings at the Simons Collaboration on the Global Brain. This became my main postdoctoral project, and the results went into a paper published last year in Nature. Our work would not have been possible without the pioneering work by Mante and Sussillo, and it feels very satisfying to build a deeper understanding of flexible decision-making from the original ideas that were first laid out in their paper. (The Transmitter and the Simons Collaboration on the Global Brain share the same parent organization, the Simons Foundation.)
Is there an underappreciated aspect of the paper you think other neuroscientists should know about?
This is tricky. This is not an obscure, unknown paper. It has almost 2,000 citations, and it is referenced at COSYNE every year.
I think more neuroscientists should appreciate how paradigm-shifting this paper was in using an RNN model. I read it for the first time in early 2014, and I feel like I did not fully understand it until maybe 2017 or 2018. It took me time to grasp how powerful RNNs are in helping us understand brain mechanisms.
What paper changed your life?
Recommended reading
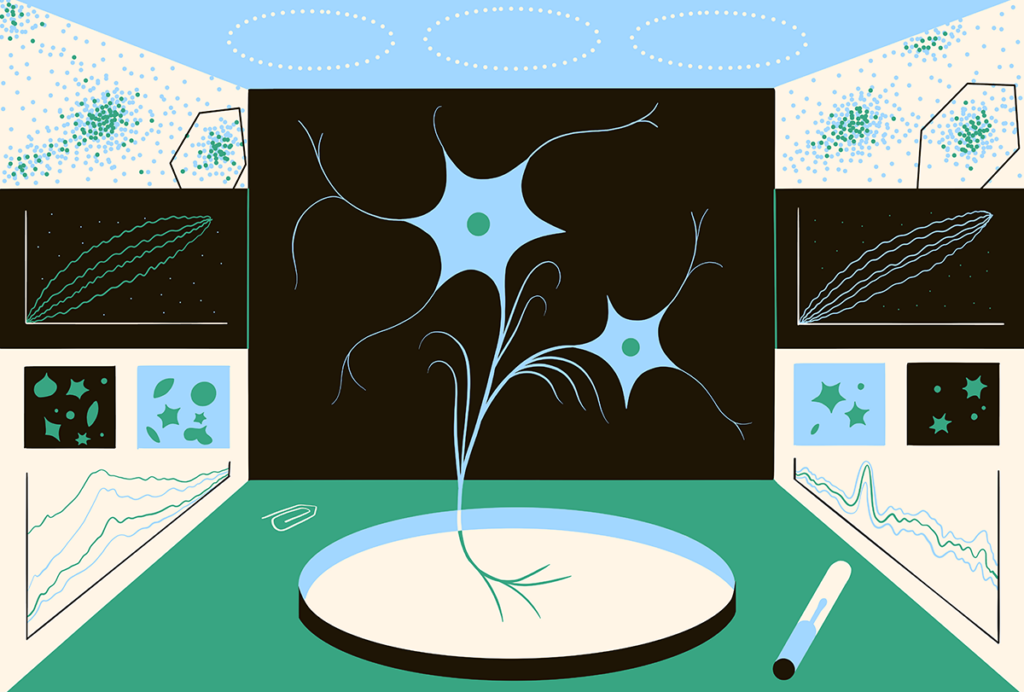
This paper changed my life: Shane Liddelow on two papers that upended astrocyte research
Explore more from The Transmitter
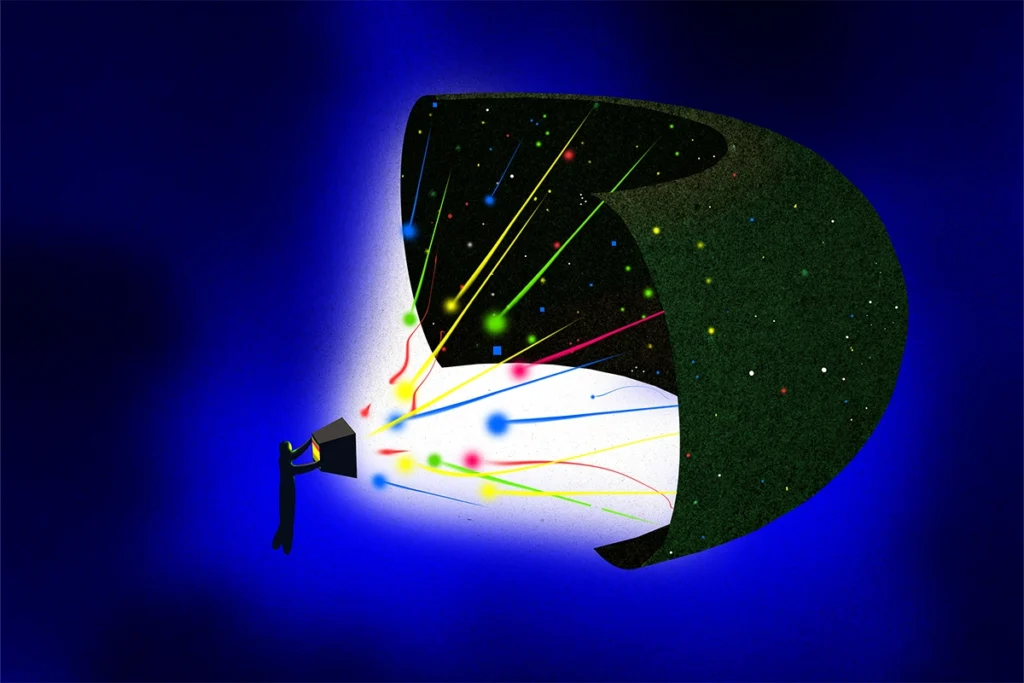