Emily S. Finn is assistant professor of psychological and brain sciences at Dartmouth College, where she directs the Functional Imaging and Naturalistic Neuroscience (FINN) Lab. Finn has pioneered techniques such as functional connectome fingerprinting and connectome-based predictive modeling for predicting individual behaviors from functional brain connectivity. Her current work is focused on how within- and between-individual variability in brain activity relates to appraisal of ambiguous information under naturalistic conditions such as watching movies or listening to stories.
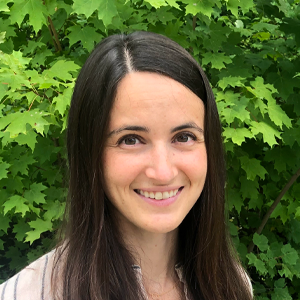
Emily S. Finn
Assistant professor of psychological and brain sciences
Dartmouth College
From this contributor
To improve big data, we need small-scale human imaging studies
By insisting that every brain-behavior association study include hundreds or even thousands of participants, we risk stifling innovation. Smaller studies are essential to test new scanning paradigms.
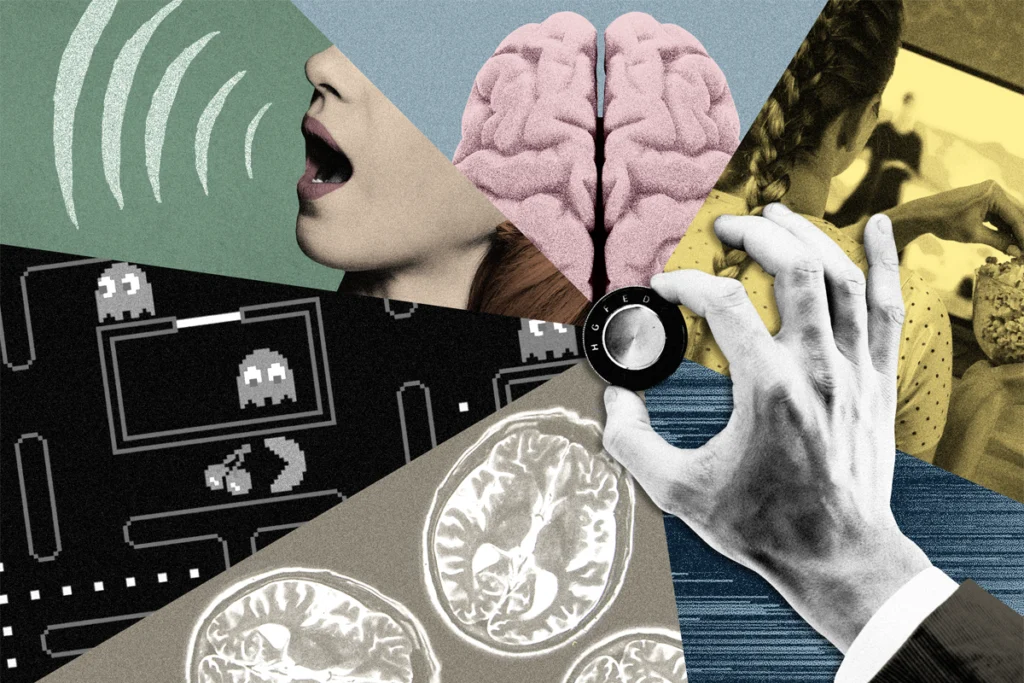
To improve big data, we need small-scale human imaging studies
Explore more from The Transmitter
More than two dozen papers by neural tube researcher come under scrutiny
One of the studies, published in 2021 in Science Advances, received an editorial expression of concern on 21 May, after the journal learned that an institutional review of alleged image problems is underway.
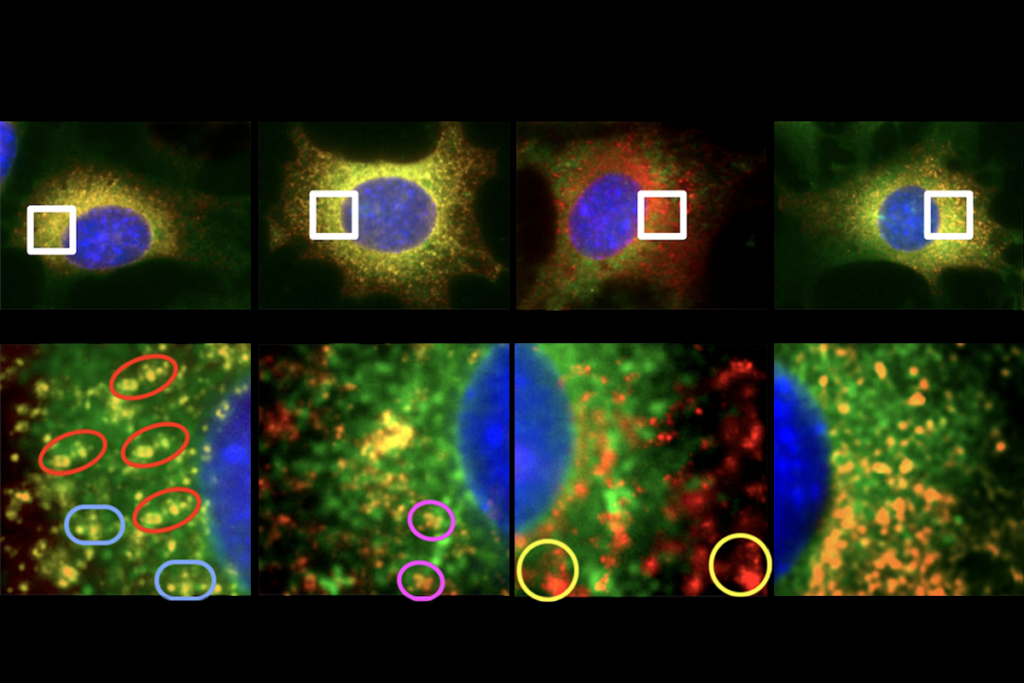
More than two dozen papers by neural tube researcher come under scrutiny
One of the studies, published in 2021 in Science Advances, received an editorial expression of concern on 21 May, after the journal learned that an institutional review of alleged image problems is underway.
On the importance of reading (just not too much)
The real fun of being a neuroscientist, and maybe the key to asking and answering new questions, is to think big and take intellectual risks.
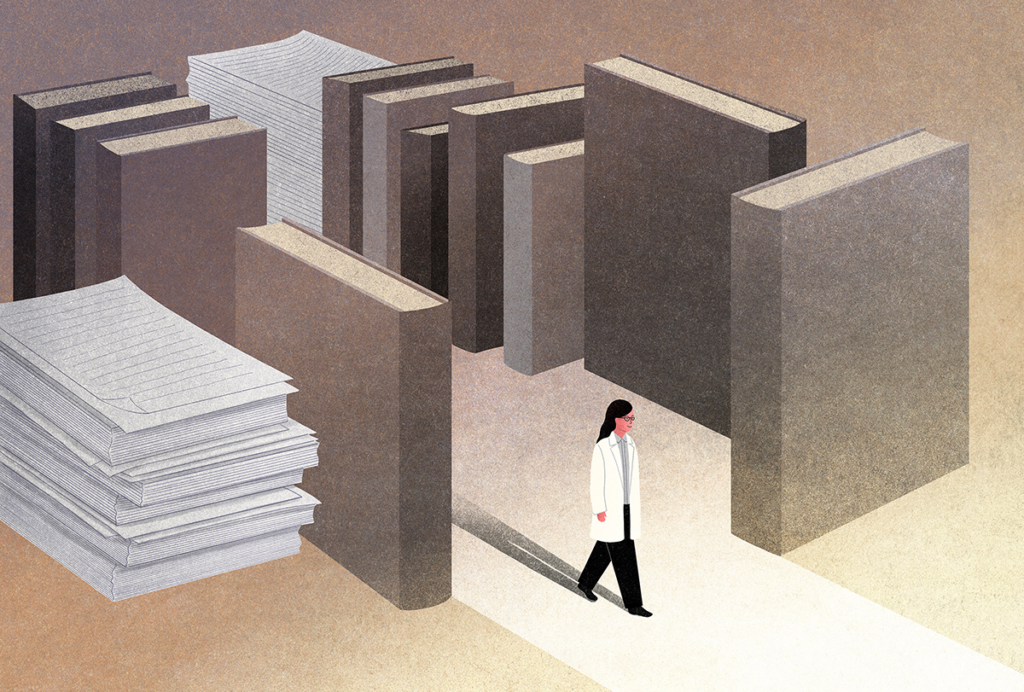
On the importance of reading (just not too much)
The real fun of being a neuroscientist, and maybe the key to asking and answering new questions, is to think big and take intellectual risks.
How developing neurons simplify their search for a synaptic mate
Streamlining the problem from 3D to 1D eases the expedition—a strategy the study investigators deployed to rewire an olfactory circuit in flies.
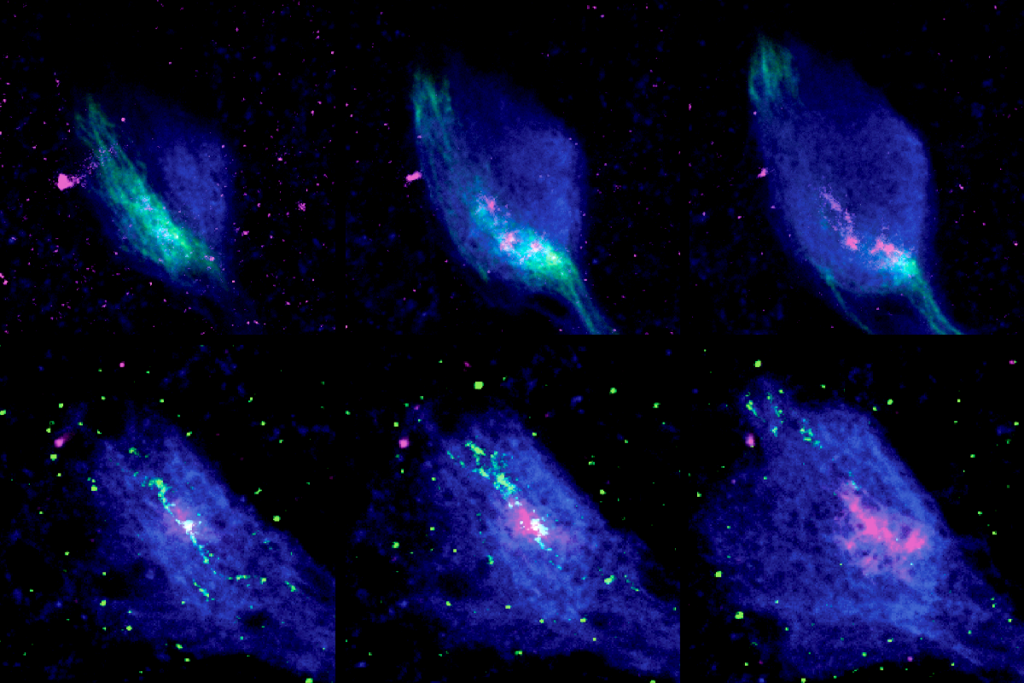
How developing neurons simplify their search for a synaptic mate
Streamlining the problem from 3D to 1D eases the expedition—a strategy the study investigators deployed to rewire an olfactory circuit in flies.