Learning in living mice defies classic synaptic plasticity rule
Donald Hebb’s theory—memorably summarized as “cells that fire together, wire together”—does not explain the shifting hippocampal connections in mice learning to navigate a virtual environment, according to a new study.
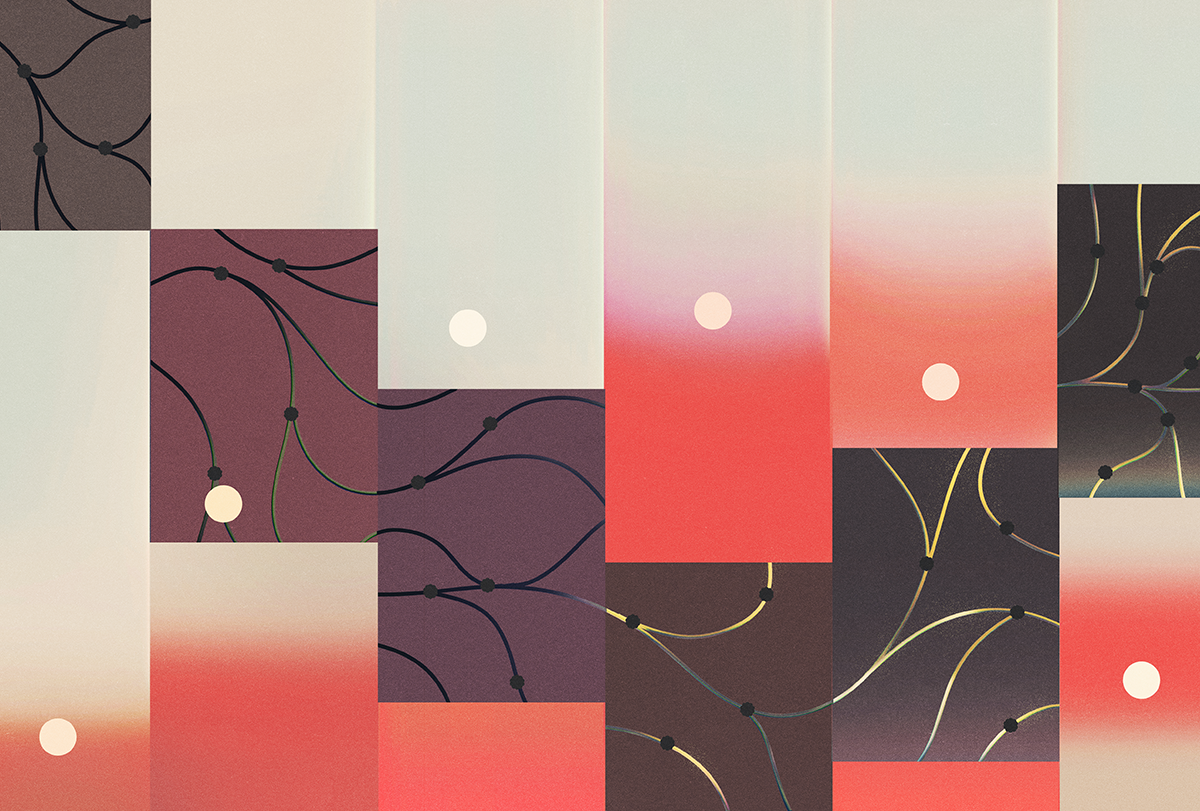
Donald Hebb famously proposed in 1949 that when neurons fire together, the synaptic connections between them strengthen, forming the basis for long-term memories. That theory—which held up in experiments in rat hippocampal slice cultures—has shaped how researchers understand synaptic plasticity ever since.
But a new computational modeling study adds to mounting evidence that Hebbian plasticity does not always explain how changing neuronal connections enable learning. Rather, behavioral timescale synaptic plasticity (BTSP), which can strengthen synapses even when neurons fire out of sync, better captures the changes seen in CA1 hippocampal cells as mice learn to navigate a new environment, the study suggests.
Hebbian spike-timing-dependent plasticity occurs when a neuron fires just ahead of one it synapses onto, leading to a stronger connection between the two cells. BTSP, on the other hand, relies on a complex spike, or a burst of action potentials, in the postsynaptic cell, which triggers a calcium signal that travels across the dendritic arbor. The signal strengthens synaptic connections with the presynaptic cell that were active within seconds of that spike, causing larger changes in synaptic strength. BTSP helps hippocampal cells establish their place fields, the positions at which they fire, previous work suggests. But it was unclear whether it also contributes to learning, says Mark Sheffield, associate professor of neurobiology at the University of Chicago, who led the new study.
The new findings suggest that it does—challenging how researchers traditionally think about plasticity mechanisms in the hippocampus, says Jason Shepherd, associate professor of neurobiology at the University of Utah, who was not involved in the research. “The classic rules of plasticity that we have been sort of thinking about for decades may not be actually how the brain works, and that’s a big deal.”
S
heffield and his colleagues built on a previous study that used calcium imaging to record neuronal activity in the hippocampus of 11 mice as the animals ran on a spherical treadmill to explore an unfamiliar virtual environment. Location-responsive cells, or place cells, in the CA1 and CA3 regions of the hippocampus fired each time an animal navigated past specific locations along the virtual linear track.As the animals became acclimated to the environment, more place cells joined in on the activity, the team found. And when that happened, a cell’s place field often changed: Most place fields shifted backward along the track, but some moved forward, and some remained stable. That movement indicates that the cells’ representation of space, and the animal’s mental map of their route, also shifts as a mouse learns about its environment, Sheffield says.
In the new work, Sheffield and his colleagues used computational modeling to understand which plasticity rules could best explain the observed shift in neurons’ place fields. The team built models of CA1 and CA3 place cells that spike in response to activation of their place fields, and they observed how those place fields changed in response to synaptic weights that strengthened according to different rules.
When the team enabled the cells to update their synapses based on Hebbian rules—when a CA3 place cell fires, it causes the CA1 place cell to fire, thus strengthening their connection—the model resulted in larger, but not shifted, place fields, the team found.
They then applied the rules of BTSP to the model: A CA1 place cell has a complex spike, which causes synaptic strengthening of CA3 synapses that were active within a few seconds of that spike. This event results in the shifting of the CA1 place field. The resulting dynamics captured the range of backward and forward place-field drift seen in vivo. The findings were published in March in Nature.
T
he results point to BTSP as an important component of how the brain learns, whereas Hebbian plasticity was nearly “useless” for modeling CA1 cells, Sheffield says.They also help explain the range of responses hippocampal cells can show during learning, says Kari Hoffman, associate professor of psychology at Vanderbilt University, who was not involved in the work. “I find that quite compelling.”
Other plasticity rules likely drive learning-induced synaptic changes too, Sheffield says. For example, although BTSP explained the CA1 place cells’ shifting fields, neither it nor Hebbian plasticity could model the activity changes observed in CA3 cells; only an altered version of BTSP worked, he and his colleagues found.
And despite the findings, it is unlikely that Hebbian plasticity is never used in learning and memory, says Timothy O’Leary, professor of information engineering and neuroscience at the University of Cambridge, who was not involved in the study. “I think anyone who’s attempted to extract some kind of consistent rule out of biological system and hasn’t been humbled by that process hasn’t really been paying attention,” he says.
As methods to observe neuronal activity in real time continue to advance, these rules become easier to test, Sheffield says. “I think we’ve been stuck in the Hebbian world for way too long, and it just is not the story. It’s not the full story.”
Recommended reading
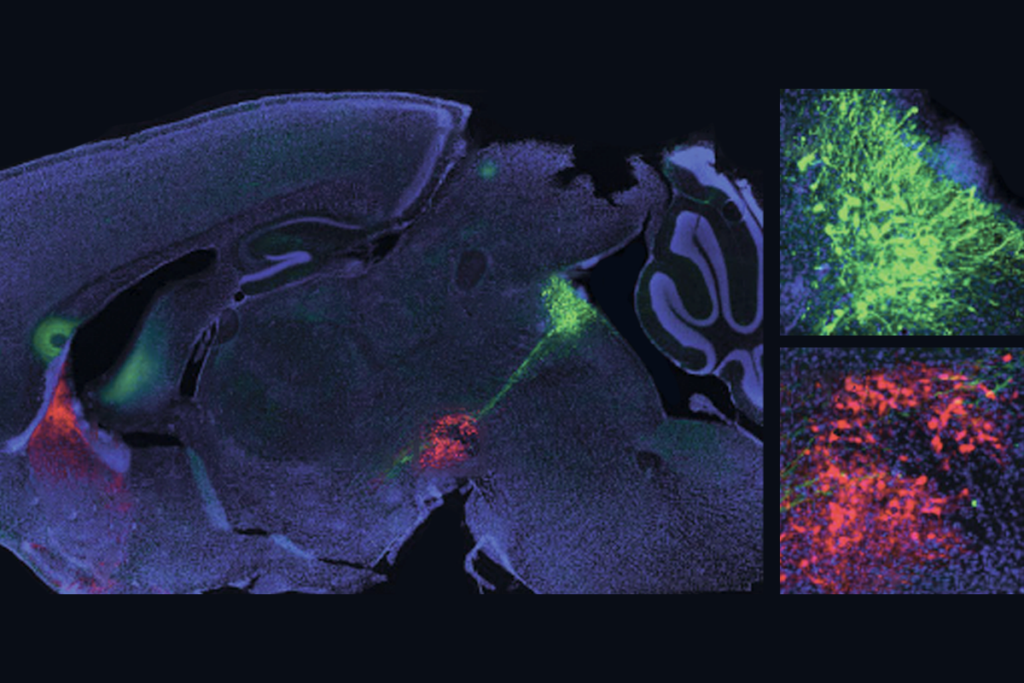
Dopamine ‘gas pedal’ and serotonin ‘brake’ team up to accelerate learning
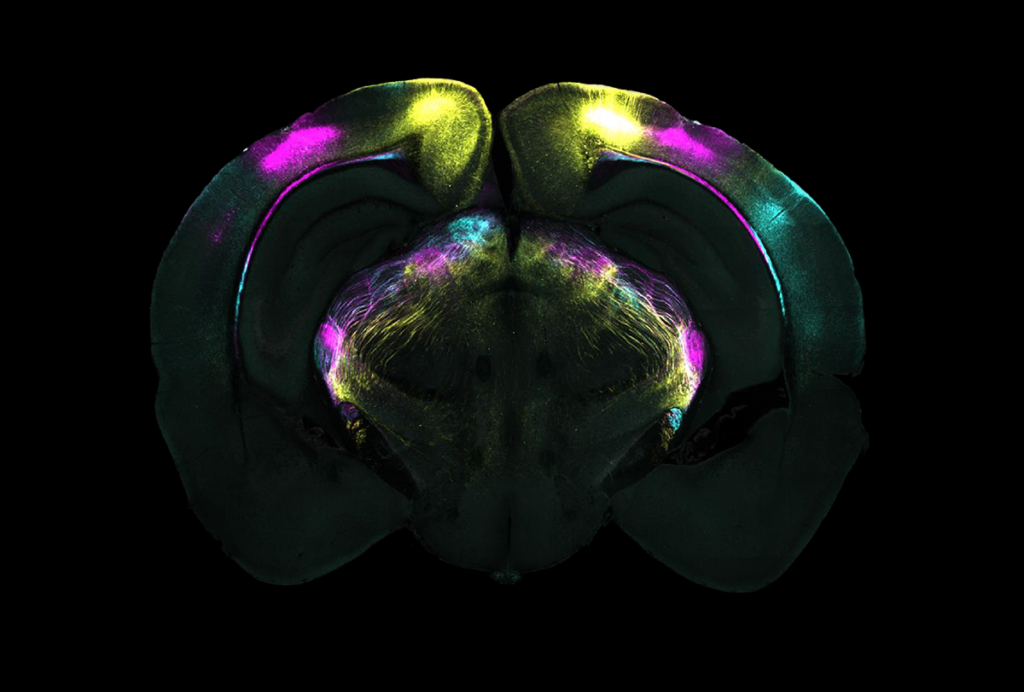
Subthalamic plasticity helps mice squelch innate fear responses
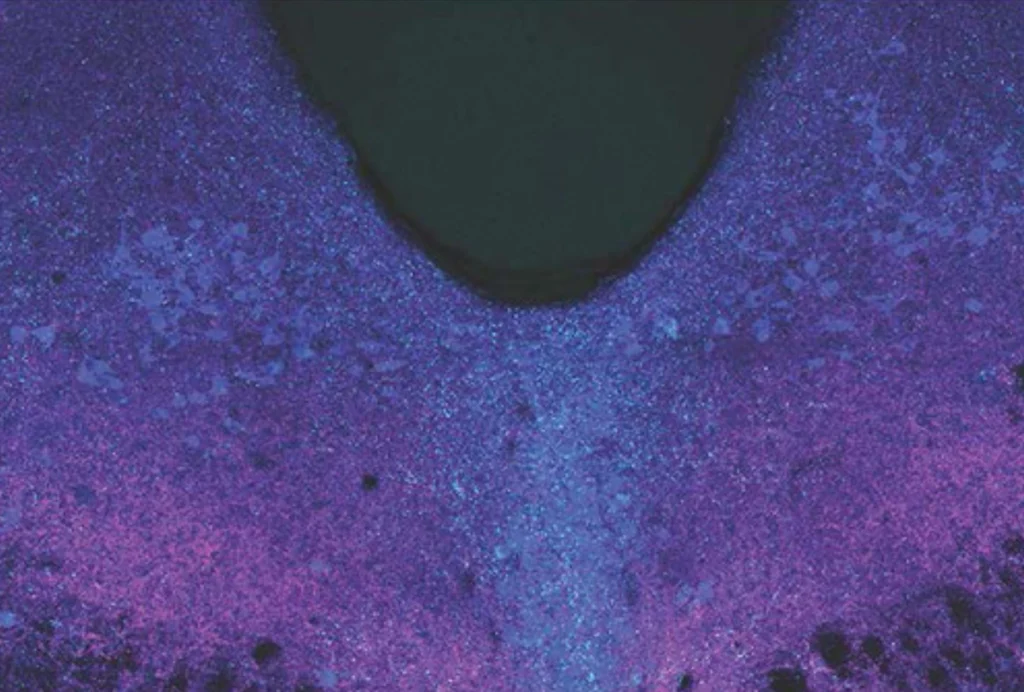
Neurotransmitter switch-up helps fan extreme stress into full-blown fear
Explore more from The Transmitter
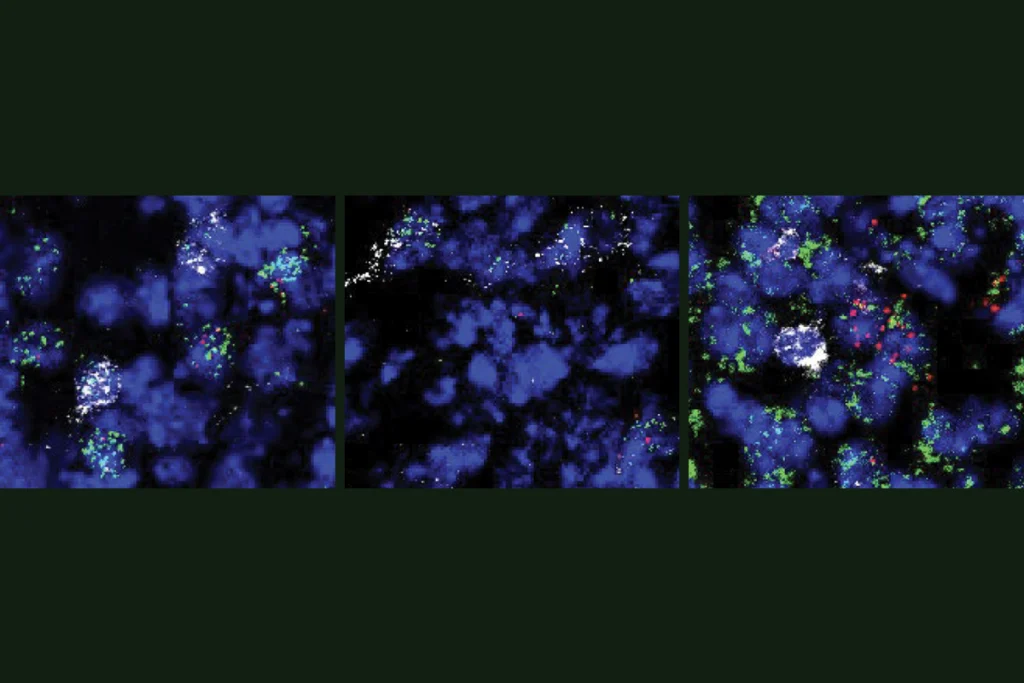
Novel neurons upend ‘yin-yang’ model of hunger, satiety in brain
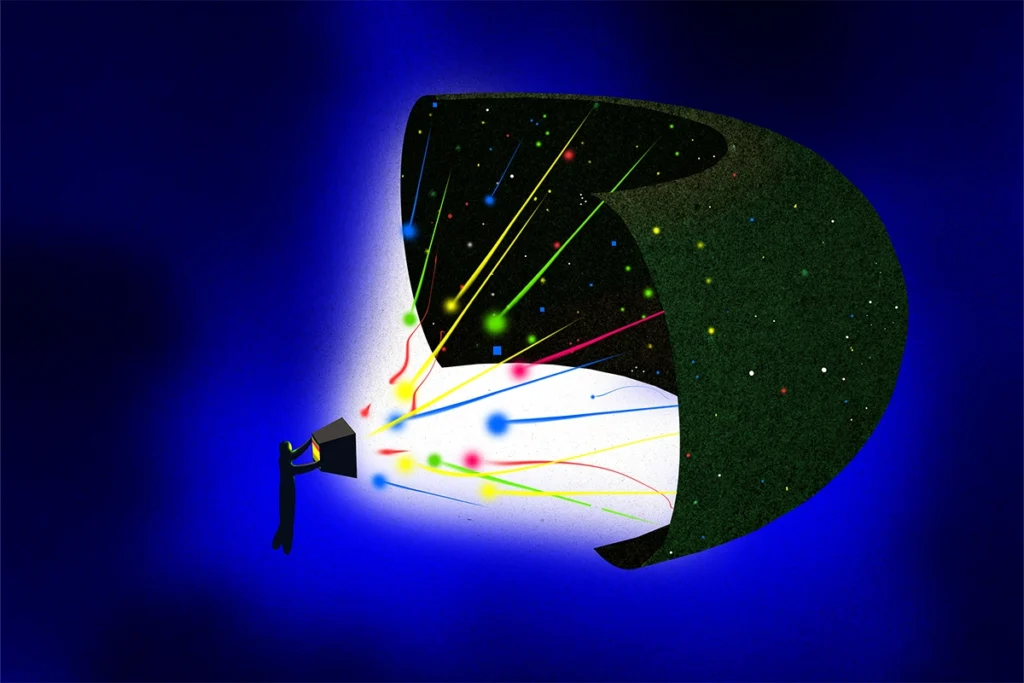
Imagining the ultimate systems neuroscience paper
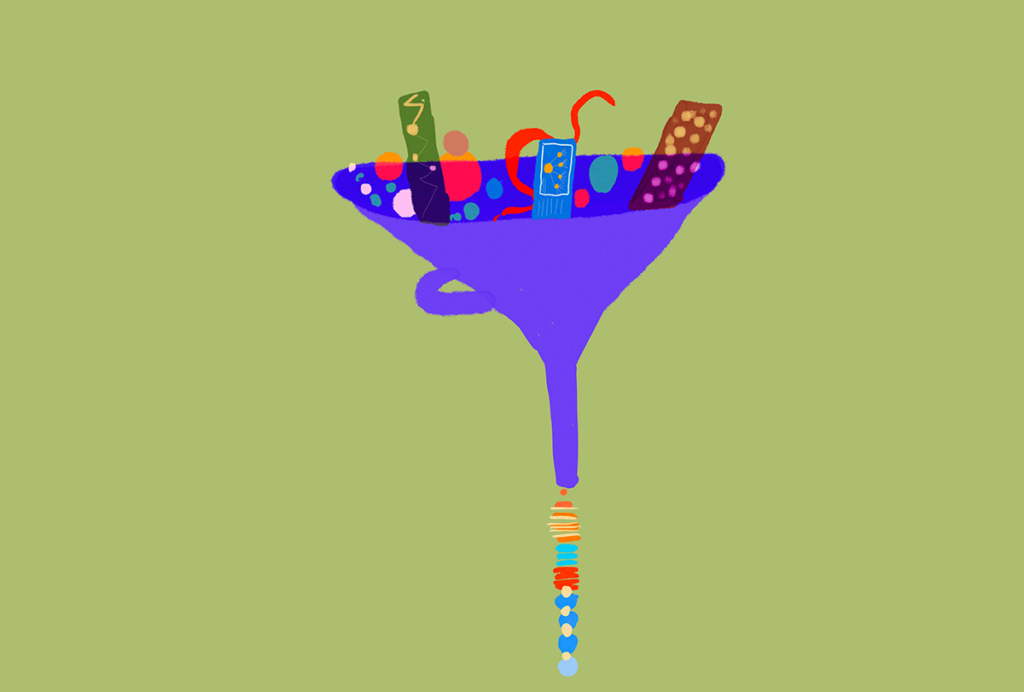