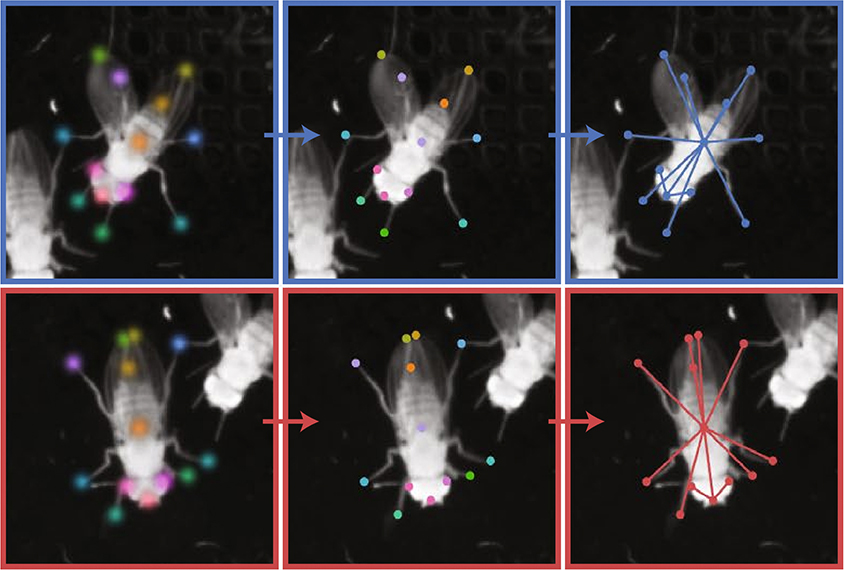
Deep-learning tool tracks interacting animals in real time
By coupling the tool — called SLEAP — with optogenetics, researchers can determine the neural circuits underlying social behaviors.
A new open-source tool enables researchers to track the movements of multiple animals in real time. Integrating the tool with optogenetics, a technique that uses light to control clusters of neurons, also makes it possible to activate brain regions in response to specific cues — social interaction or aggression, for example — and probe which neural circuits play a causal role.
The tool, called Social LEAP Estimates Animal Poses (SLEAP), tracks animals’ body parts rather than the body as a whole. That capability is important because “we can detect a lot more subtle types of key behaviors, such as the kinds of things you would see in [autism] models,” says study investigator Talmo Pereira, a Salk fellow at the Salk Institute for Biological Studies in La Jolla, California.
Scientists have used SLEAP, described in Nature Methods in April and freely available online, to study mice, gerbils, bees, fruit flies and many other life forms. “People have used SLEAP to track single cells,” Pereira says. “People have also used it all the way through to track whales. It pretty much spans the gamut there.” Pereira released an earlier version of the tool in September 2020, as a preprint.
To use the tool, researchers first manually annotate a subset of video frames, tagging each animal’s head, tail, limbs or any other body part. The algorithm learns from those annotations and automatically annotates the rest of the clip.
SLEAP is faster than other machine-learning tools that monitor groups of animals, such as Multi-Animal DeepLabCut (reported in the same issue of Nature Methods), with a delay of just 3.2 milliseconds. That’s roughly the same amount of time it takes for an action potential to ripple through a neuron.
That short delay opens up a variety of research applications, according to Pereira. “The fact that we can now do closed-loop, behavior-triggered modulation, in real time and with multiple animals, is pretty unique.”
When tracking a single animal, SLEAP is accurate for about 90 percent of the data and can process 2,194 frames of video footage per second. These metrics dip, predictably, when multiple animals are involved. For videos of interacting flies and mice, SLEAP can process more than 750 and 350 frames per second, respectively. When SLEAP ‘learns’ from just 200 manually labeled video frames, it performs about 90 percent as well as a model trained on thousands of video frames.
A
SLEAP automatically tracked when a male fly approached the female with CsChrimson. The tool delivered a pulse of light in response, action potentials flared, and the unmated flies reflexively blocked the incoming male from copulation, all in real time.
In mice that model autism and groom excessively — a proxy for repetitive behaviors in people with the condition — researchers might use the new tool to track animals and “turn off those neurons as [mice are] starting to scratch,” says Sam Golden, assistant professor of neuroscience at the University of Washington in Seattle, who was not involved in the study. If the animals “immediately stop, that suggests a very strong causal relationship between the population of neurons we’re interested in and the behavioral output.”
Researchers with no programming experience can deploy SLEAP, which was built entirely using the Python programming language. Pereira says it is already being “used in at least 70 labs in 58 universities,” and that it has been downloaded about 40,000 times, which suggests there’s a sizeable community to help resolve problems and troubleshoot errors.
Using the tool’s existing settings, without any tweaks, works for about “95 percent of applications,” Pereira says. The software package contains built-in tools to process videos, train the model and benchmark the accuracy of video annotations. And teams can export data from SLEAP and use those to train behavioral classification algorithms, such as SimBA, to predict when an animal is burying a marble, for instance, or attacking another mouse.
Last week, Pereira received an email in which a professor said their student was able to set up SLEAP and use it to track data in less than an hour. And “within another 30 minutes, they trained their undergraduate student to be able to do it themselves autonomously, which I found quite satisfactory,” Pereira says. “That’s really the thing that we’re building towards.”
Recommended reading
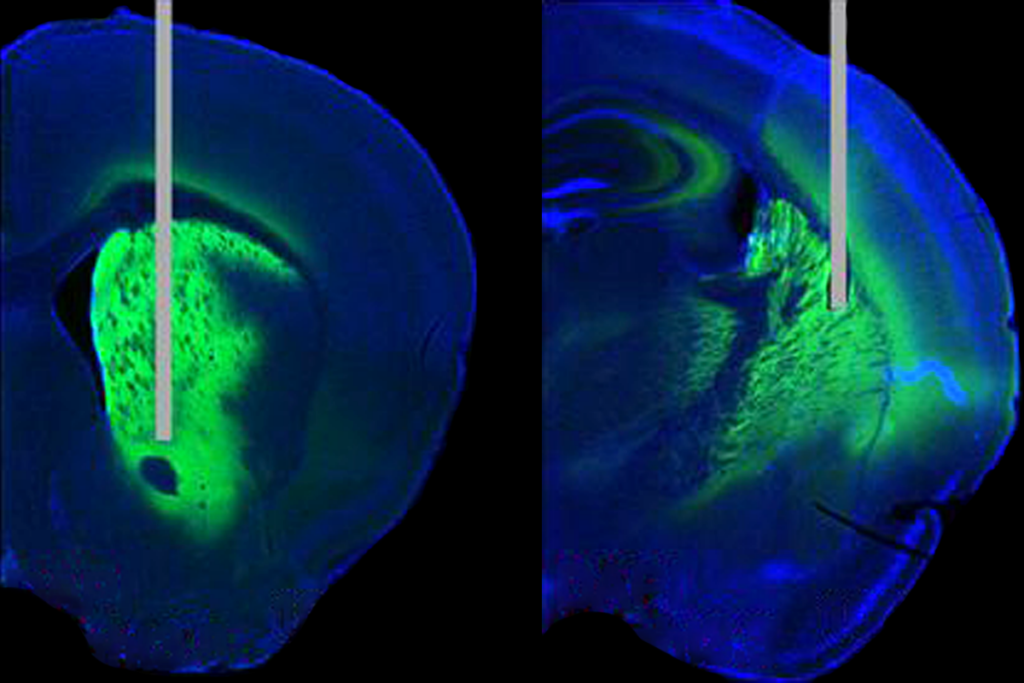
Bespoke photometry system captures variety of dopamine signals in mice
Plaque levels differ in popular Alzheimer’s mouse model depending on which parent’s variants are passed down
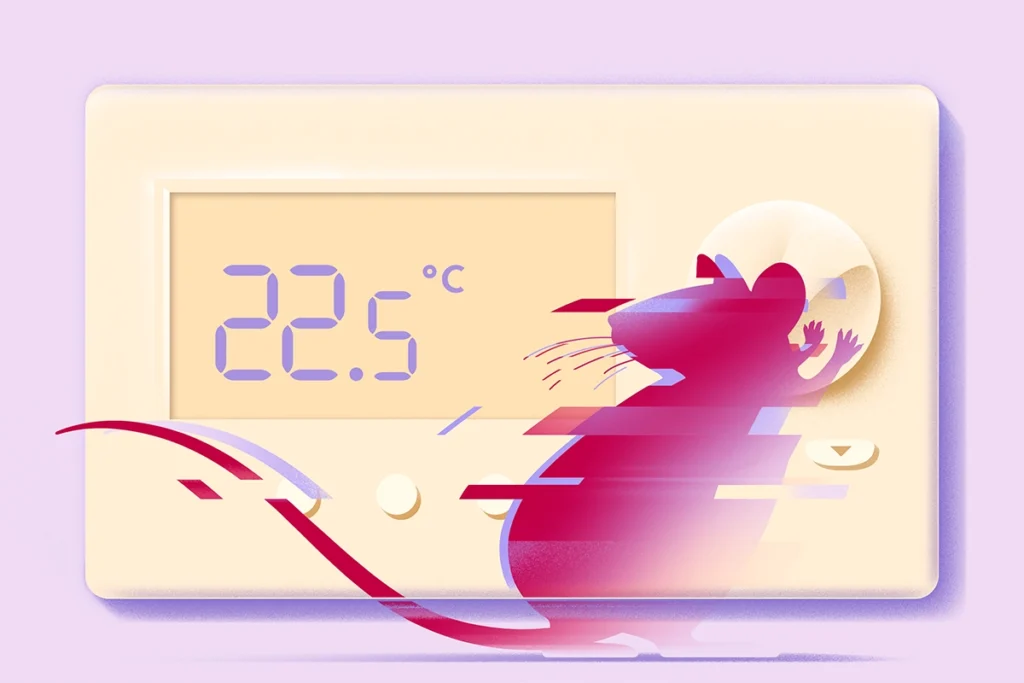
Mouse housing temperatures can cook experimental outcomes
Explore more from The Transmitter
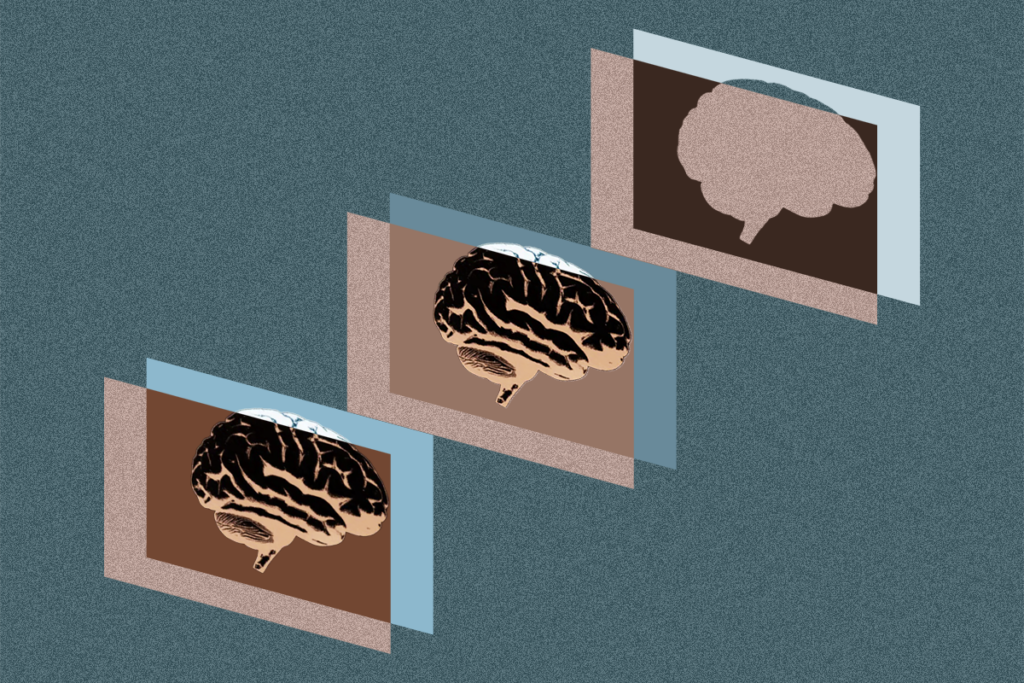
Exclusive: Recruitment issues jeopardize ambitious plan for human brain atlas
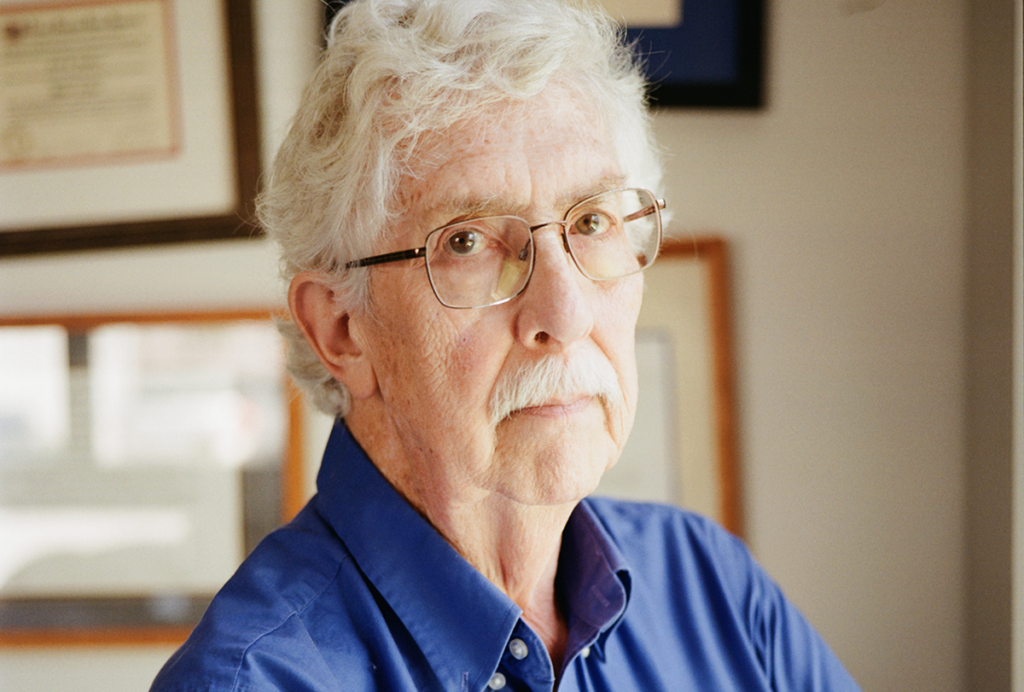