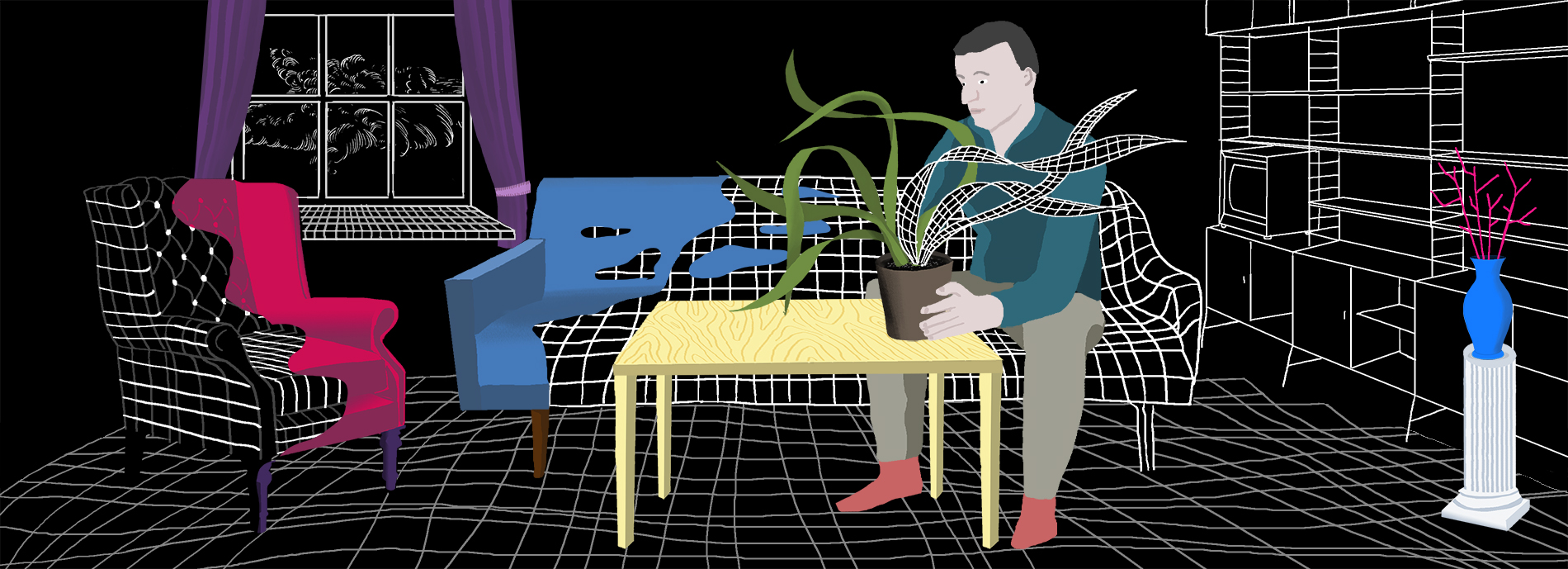
How autism may stem from problems with prediction
A ‘predictive coding’ theory of autism suggests that many of the condition’s hallmark traits occur when sensory input overrides expectation in the brain.
S
atsuki Ayaya remembers finding it hard to play with other children when she was young, as if a screen separated her from them. Sometimes she felt numb, sometimes too sensitive; sometimes sounds were muted, sometimes too sharp. As a teenager, desperate to understand herself, she began keeping a journal. “I started to write my ideas in my notebooks, like: What’s happened to me? Or: What’s wrong with me? Or: Who am I?” she says, “I wrote, wrote, wrote. I filled maybe 40 notebooks.”Today, at 43, Ayaya has a better sense of who she is: She was diagnosed with autism when she was in her early 30s. As a Ph.D. student in the history and philosophy of science at the University of Tokyo, she is using the narratives from her teen years and after to generate hypotheses and suggest experiments about autism — a form of self-analysis called Tojisha-Kenkyu, introduced nearly 20 years ago by the disability-rights movement in Japan.
In Ayaya’s telling, her autism involves a host of perceptual disconnects. For example, she feels in exquisite detail all the sensations that typical people readily identify as hunger, but she can’t piece them together. “It’s very hard for me to conclude I’m hungry,” she says. “I feel irritated, or I feel sad, or I feel something [is] wrong. This information is separated, not connected.” It takes her so long to realize she is hungry that she often feels faint and gets something to eat only after someone suggests it to her.
She has also come to attribute some of her speech difficulties to a mismatch between how her voice sounds to her and how she expects it to sound. “Just after she speaks, her own voice feeds back to her ears, and she tends to notice the difference,” says her collaborator Shin-ichiro Kumagaya, a pediatric neurologist at the University of Tokyo who studies autism using Tojisha-Kenkyu. The effect is like the awkward echo on a phone line that makes it difficult to carry on a conversation — except that for Ayaya, it’s like that almost all the time.
Ayaya’s detailed accounts of her experiences have helped build the case for an emerging idea about autism that relates it to one of the deepest challenges of perception: How does the brain decide what it should pay attention to? Novelty captures attention, but to decide what is novel, the brain needs to have in place a prior expectation that is violated. It must also assign some level of confidence to that expectation, because in a noisy world, not all violations are equal: Sometimes things happen for a reason, and sometimes they just happen.
The best guess scientists have for how the brain does this is that it goes through a process of meta-learning — of figuring out what to learn and what not to. According to this theory, biases in the meta-learning process explain the core features of autism. The theory essentially reframes autism as a perceptual condition, not a primarily social one; it casts autism’s hallmark traits, from social problems to a fondness for routine, as the result of differences in how the mind processes sensory input.
Consider what happens when we are new to a situation or a subject. Every detail — every bump on a graph, every change in a person’s tone of voice — seems meaningful. As we gain experience, though, we start to learn what the rule is and what the exception. The minutiae become less salient; the brain shifts its focus to the big picture. In this way, the brain masters one challenge and moves to the next, keeping itself at the cusp between boredom and frustration. Autism might represent a different learning curve — one that favors detail at the price of missing broader patterns.
Unlike other ‘unified theories’ of autism — those that purport to explain all aspects of the condition — this one builds on a broad account of brain function known as predictive coding. The premise is that all perception is an exercise of model-building and testing — of making predictions and seeing whether they come true. In predictive-coding terms, the brain of someone with autism puts more weight on discrepancies between expectations and sensory data. Whereas the typical brain might chalk up a stray car horn to chance variation in a city soundscape and tune it out, every beep draws conscious attention from the autism brain. “It provides a very parsimonious explanation for the cardinal features of autism,” says Karl Friston, a neuroscientist at University College London who helped develop the mathematical foundations of predictive-coding theory as it applies to the brain.
For now, the model is vague on some crucial details. “There’s many loose pieces,” says Katarzyna Chawarska, an autism researcher at Yale University. And some question whether a single model could ever account for a condition as heterogeneous as autism. Yet proponents say this very diversity argues for a unified theory. Understanding a fundamental cause might yield treatments that are equally broad in their reach. “If prediction truly is an underlying core impairment [in autism], then an intervention that targets that skill is likely to have beneficial impacts on many different other skills,” says computational neuroscientist Pawan Sinha of the Massachusetts Institute of Technology.
”"All experience is controlled hallucination. You experience, in some sense, the world that you expect to experience." Andy Clark
Predictive coding 101:
T
he basic premise of predictive coding goes back to the mid-19th century German physicist and psychologist Hermann von Helmholtz, and arguably to the philosopher Immanuel Kant, both of whom maintained that our subjective experience is not a direct reflection of external reality, but rather a construct. “All experience is controlled hallucination,” says Andy Clark, a cognitive scientist at the University of Edinburgh in Scotland. “You experience, in some sense, the world that you expect to experience.”One reason we rely so much on expectation is that our perceptions lag behind reality. Much of what we do, from playing sixteenth notes on the guitar to adjusting our stance on a jerking subway train, happens faster than the 80 milliseconds or longer it takes our conscious minds to register input, let alone act upon it. And so the brain must always be anticipating what comes next. It generates a model of the world, makes decisions on that basis, and updates the model based on sensory feedback. In the language of probability theory, the brain is a Bayesian inference engine, merging prior expectations with current conditions to assess the probability of future outcomes.
Predicting and updating needn’t be — and usually aren’t — conscious acts; the brain builds its models on multiple subconscious levels. Nearly 20 years ago, researchers showed how the visual cortex works in a hierarchical and predictive fashion. The primary visual cortex generates a prediction for small-scale image patterns such as edges. It refines its prediction to match the incoming signals from the retina, but if this localized fine-tuning is not enough, it passes the buck to the secondary cortex, which revamps its expectations of what larger-scale geometric patterns must be out there. And so it goes up the hierarchy, evoking ever more sweeping changes, until the buck stops at the highest level: consciousness. (Neuroscientists adopted the term ‘predictive coding’ from communications engineering, which in the 1950s developed the idea of transmitting discrepancies rather than raw data, to minimize the amount of information a network needs to carry.)
When the brain perceives a discrepancy, it can respond by either updating its model or deeming the discrepancy to be a chance deviation, in which case it never swims up into conscious awareness. “You want to attenuate fake news,” Friston says. Or there is a third alternative: Faced with a discrepancy between model and world, the brain might also update the world — say, by moving an arm or flexing a hand to make the prediction come true. “One can reduce prediction errors not only by updating the model but by performing actions,” says Anil Seth, a neuroscientist at the University of Sussex in the United Kingdom. In this way, predictive coding can be not just a system for perception, but also for motor control.
But which of these three responses should the brain take? In the predictive-coding model, the brain decides among them by assigning its predictions a precision — the statistical variability it expects from the input. Precision is the brain’s version of an error bar: High precision (low variance) plays up discrepancies: “This is important. Pay attention!” Low precision (high variance) downplays them: “Just a fluke, never mind.”
Suppose the brain consistently set the precision higher than conditions called for. It would be as if Google Maps understated its uncertainty about a person’s location and drew that approximate blue circle around them too small. Random variations in the signal that cause the estimated location to jump around would look like real motion. One might well watch it and wonder what could possibly be causing that person to hop around like that: Where others saw noise, you’d see signal.
That same sort of miscalculation may occur in people with autism. “Maybe autism spectrum disorder involves a kind of failure to get that Bayesian balance right, if you like, or at least to do it in the neurotypical way,” Clark says.
Extreme precision:
A
lthough the ideas underlying predictive coding date back at least 150 years, it came of age as a theory in neuroscience only in the 1990s, just as machine learning was transforming computer science — and that’s no coincidence. The two fields have cross-fertilized each other.Many machine-learning systems have a parameter called the ‘learning rate’ that plays the role of predictive precision, Friston says. An artificial neural network learns by trial and error; if it classifies a puppy as a kitten, it tweaks its internal connections to do better next time, and the learning rate dictates the amount of tweaking. The system can adjust the learning rate to optimize its training and avoid problems such as overfitting the data — recognizing every kitten and puppy it has already encountered, but failing to grasp the general features that distinguish these pets. The learning rate is often high at first but decreases over time. In the predictive-coding model, the typical brain, too, starts with a high precision and gradually dials it down, possibly by adjusting the concentrations of chemical messengers such as norepinephrine and acetylcholine. “The belief is that precision is usually encoded by neuromodulators in the brain — chemicals that change the gain on cortical responses,” says Rebecca Lawson of the University of Cambridge in the U.K. When it’s time to initiate another round of learning, the brain cranks up the precision again.
In people with autism, however, the precision may have a tendency to jump to a high level or get stuck there — for whatever reason, the brain tends to overfit. This general idea was first put forward in 2010 by Columbia University neuroscientists Ning Qian and Richard Lipkin. Inspired by machine learning, they suggested that the autism brain is biased toward rote memorization, and away from finding regularities or patterns. “We can think about the difficulties of training people with [autism] as a mismatch between the learning style and the tasks,” Qian says.
The following year, another team put forth the first Bayesian model of the condition, proposing that in individuals with autism, the brain gives too little credence to its own predictions and therefore too much to sensory input. In response, two groups — one including Friston and Lawson — suggested that predictive coding could provide the mechanism for the imbalance between predictions and sensations. And in 2014, Sinha and his colleagues proposed that in autism, the brain’s predictions aren’t underweighted but simply inaccurate, which becomes especially apparent in cases where prediction is intrinsically difficult. For example, when one event follows another only slightly more often than expected to by chance, a person with autism might not notice any connection at all. A world that seems at least somewhat predictable to typical people can strike those with autism as capricious — or, as Sinha puts it, “magical.”
Although these groups focused on different parts of the predictive process, they described much the same principle: For a person with autism, the world never stops being surprising. “That is a very common narrative in individuals with [autism],” Kumagaya says. “They tend to be surprised more frequently than neurotypicals.” In a way, this view of the world facilitates some kinds of learning. For instance, studies show that people with autism do well at tasks that involve sustained attention to detail, such as spotting the odd man out in an image and identifying musical pitches. Also, they are less likely to see visual and multisensory illusions that presume strong expectations within the perceptual system.
But hyperawareness is exhausting. “You’re forever enslaved by sensations,” Friston says. Giving too much attention to the mundane would explain the sensory overload that people with autism commonly report. Some people with autism say they remain acutely conscious of buzzing lamps and rumbling air conditioners, and studies confirm they are slow to habituate to repeated stimuli.
Also in support of the predictive-coding model, people with autism can have trouble with tasks that are predictive by nature, such as catching a ball or tracking a moving dot on a screen. The problem is amplified when dealing with the most unpredictable things of all: human beings. To predict what someone will do in a given context, you may need to make a guess based on what they or someone like them did under different circumstances. That is hard for anyone, but more so for people with autism. “It’s very common, for example, for [people with autism] to get into social interactions and have difficulty taking what they’ve learned from situation A and bringing it to situation B,” Lipkin says. A lack of predictability can lead to acute anxiety, a common problem in people on the spectrum. Many features of autism, such as a preference for routine, can be understood as coping mechanisms. “When you see most of the repetitive movements, they are actively retreating to shield complexity in the natural world,” says Sander van de Cruys of the University of Leuven in Belgium.
In addition to offering explanations for a range of autism traits, predictive coding might also make sense of the confusing links between autism and schizophrenia. The theory accounts for schizophrenia as, in some ways, autism’s mirror image. In autism, sensory data overrides the brain’s mental model; in schizophrenia, the model trumps data.
Consider schizophrenia’s distinguishing feature: having auditory verbal hallucinations (hearing voices). Last year, Philip Corlett of Yale University and his colleagues studied the origin of these hallucinations by inducing mild versions in 30 people who reported hearing voices on a daily basis (half of whom had been diagnosed with psychosis) and 29 who didn’t. To do so, the researchers borrowed a trick from Russian physiologist Ivan Pavlov. They showed the participants checkerboard images while playing a tone, so that the participants came to expect the two together. Then the researchers stopped playing the tone. The participants who hadn’t reported hearing voices quickly caught on, but those who were hallucination-prone were more likely to report that they still heard the tone. The team interpreted this difference in terms of predictive coding. “People with auditory verbal hallucinations have very, very precise expectations about the relationships between visual and auditory stimuli in our task, so much so that those beliefs sculpt new percepts from whole cloth,” Corlett says. “They make you hear things that weren’t actually presented to you.”
Autism resembles schizophrenia in some ways, Corlett says. Although hearing voices is not common, people on the spectrum have elevated rates of delusions — fixed beliefs they hold in the face of all evidence to the contrary, such as being manipulated by aliens or paranormal forces. Corlett suggests that these delusions occur when sensory data are given too much weight and install a new set of beliefs, which then become lodged in place.
”"In autism, rather than being adaptively surprised when you ought to have been surprised, it's as if there's mild surprise to everything." Rebecca Lawson
Looking ahead:
T
here is still much about autism that predictive coding doesn’t explain, such as what exactly accounts for the autism brain’s hesitancy to dial back predictive precision as the brain gains experience. Researchers are still investigating which is askew: the prediction, the sensory input, the comparison of the two or the use of a discrepancy to force a model update. And what types of predictions are involved — all kinds, or just some? Our brains make predictions on many levels and timescales. People with autism do just fine with many of them.Some researchers are skeptical that problems of prediction are the root cause of autism. Psychologist James McPartland, also at Yale, says he is partial to explanations that give primacy to the condition’s social traits. If one thing characterizes autism, he says, it’s social difficulties, suggesting that researchers should focus on the mental machinery we need to interact with other people, such as face recognition. He says he finds a social explanation no less biologically plausible than a perceptual one. “We have a really clear idea where in the brain faces are processed,” he says. He also wonders about the direction of causation: Instead of predictive problems explaining social difficulties, the relationship might work in reverse, because so much of the brain’s predictive capacities are developed through social interactions. “Is social information a critical kind of information for the normative development of predictive coding?” he says.
Predictive-coding researchers themselves acknowledge that they are just beginning to test the theory in autism. “Those initial papers, they’re sort of just-so stories, in that they are post hoc — explaining data that was already collected,” Lawson says. But she and others have been conducting experiments that probe the predictive mechanisms more specifically. Many involve associative-learning tasks, in which people have to figure out the rule that governs some series of images or other stimuli. Every so often, the experimenters change the rule in a way that’s not immediately obvious and see how quickly their participants catch on.
Last year, for example, Lawson and her colleagues brought two dozen people with autism and 25 controls into the lab. They played a high or low beep, showed a picture of a face or house, and asked participants to press a button for ‘face’ or ‘house.’ At first, a high tone presaged a house 84 percent of the time, then a low tone did, then tones had only a 50-50 relation to image type, and so on. The controls slowed down whenever a run of violated expectations convinced them that the rule must have changed, but the participants with autism responded at a more consistent rate, which was slightly slower overall. The researchers concluded that the participants with autism responded as if each deviation — a house when the tone augured a face, say — signaled a change of rule, whereas typical people were inclined to write off the first few deviations as probabilistic happenstance.
For about half the participants, the researchers also measured pupil size, because pupils dilate in response to norepinephrine, one of the chemicals thought to encode predictive precision. Interpreting these results was tricky because each person followed a slightly different learning curve and formed different expectations. To determine whether a given event would seem surprising, the researchers had to model each person’s pattern of responses individually. The upshot was that the pupils of participants with autism seemed to be on a hair trigger. “In autism, rather than being adaptively surprised when you ought to have been surprised, it’s as if there’s mild surprise to everything — so, it’s sort of saying, well, that was mildly surprising, and that was mildly surprising, and that was mildly surprising, and that was mildly surprising,” Lawson says.
One intriguing approach is to build the predictive-coding theory into computer models, even robots. Artificial neural networks that embody theories of brain function could serve as digital lab rats. Researchers could tweak the model parameters to see whether they reproduce the traits of autism, schizophrenia or other conditions. In 2012, computational scientist Jun Tani and a colleague programmed a robot to simulate schizophrenia. By adding noise to the robot controller’s calculations, they led it to miscalculate the discrepancy between its expectation and its sensory data. The spurious error — a robotic hallucination, if you will — propagated up the robot’s cognitive hierarchy and destabilized its operation. “The robot shows disorganized behaviors,” says Tani, professor at the Okinawa Institute of Science and Technology in Japan. He and others are beginning to apply predictive coding to autism in this way.
If predictive coding holds up as a model for autism, it might also suggest new directions for therapies. “Different kids with autism may show impairments in somewhat different parts of that predictive chain,” Chawarska says, which might call for a range of clinical approaches. When she meets with parents, she uses the idea of prediction to help them understand their child’s experience of the world, telling them: “Your child really has tremendous difficulties understanding what’s going to happen next,” she says. “It’s something that really comes through, particularly with these very, very young kids. Their anguish and difficulty in relating to events is that they simply don’t know where they fit.”
If nothing else, predictive coding might offer the insight some young people crave — as Ayaya did when she was a teenager. “I noticed the differences between me and other kids, and I was thinking, why was this going on?” she recalls. As an adult, she says, her anxiety has abated, not just because of the self-knowledge she has achieved, but also because of the awareness shown by her peers and friends. Often, the typical people she spends time with know about her condition, she says. “They know me. [So] I feel more free to ask, ‘I got surprised, but didn’t you?’”
Syndication
This article was republished in Science.
Recommended reading
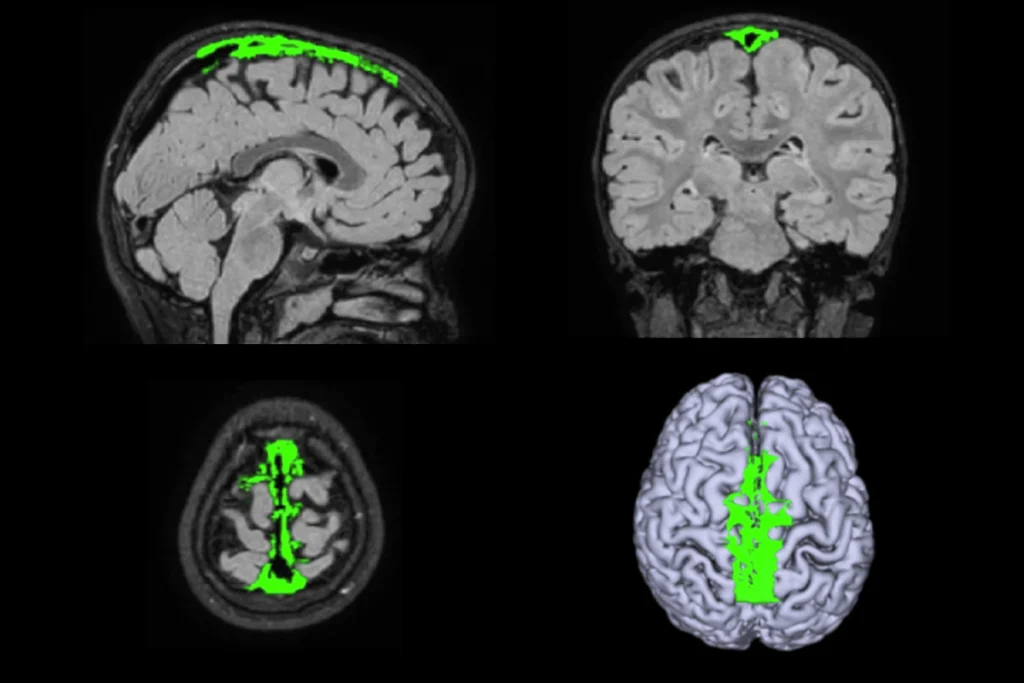
Okur-Chung neurodevelopmental syndrome; excess CSF; autistic girls
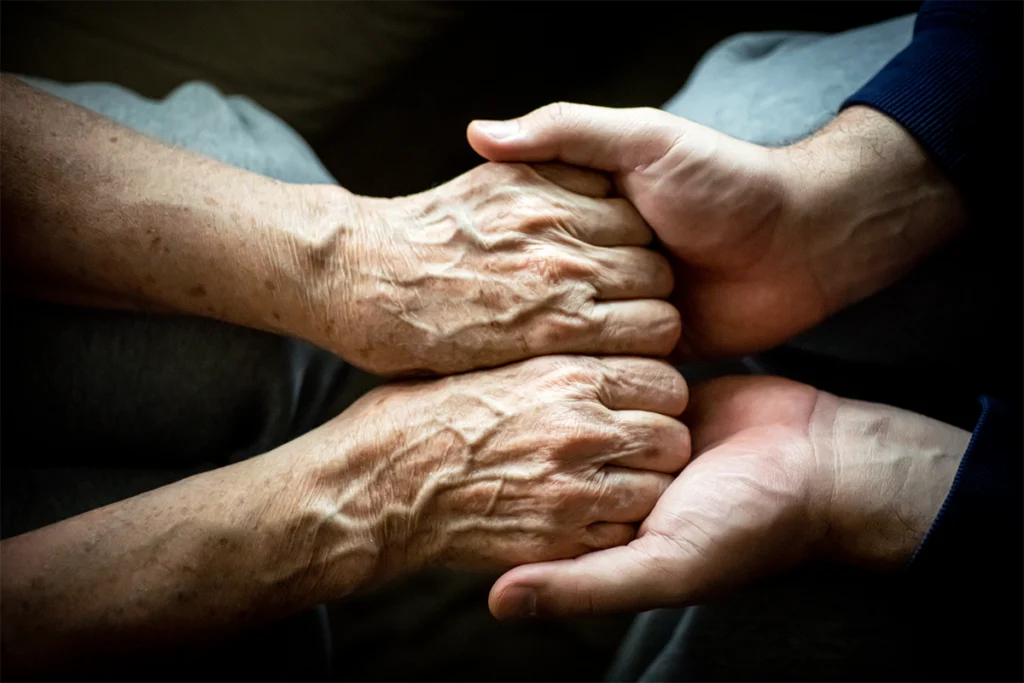
New catalog charts familial ties from autism to 90 other conditions
Explore more from The Transmitter
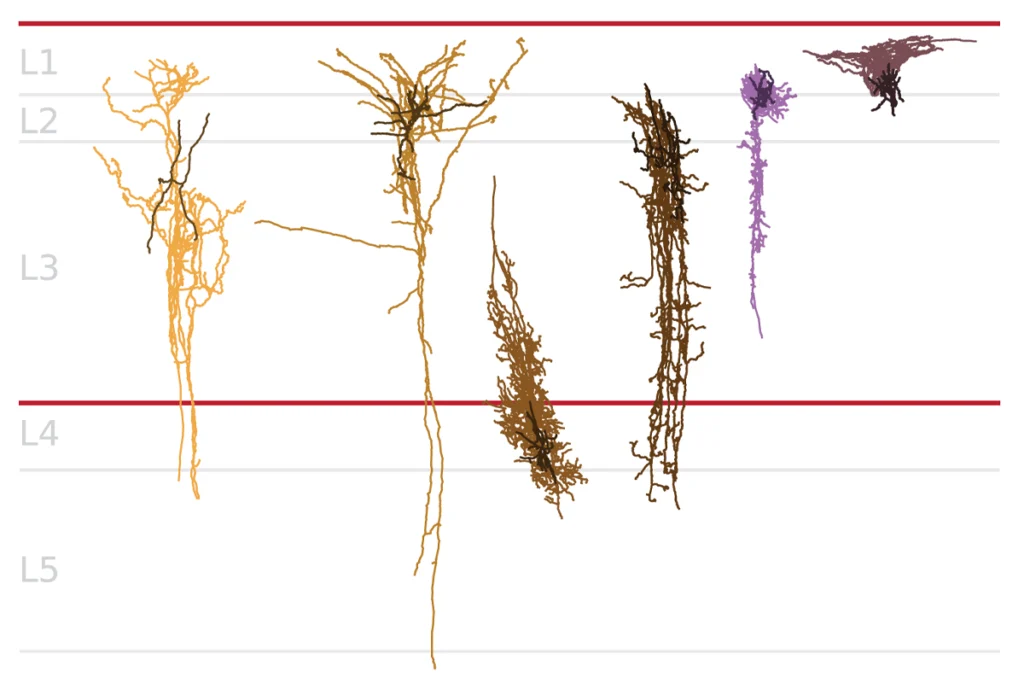