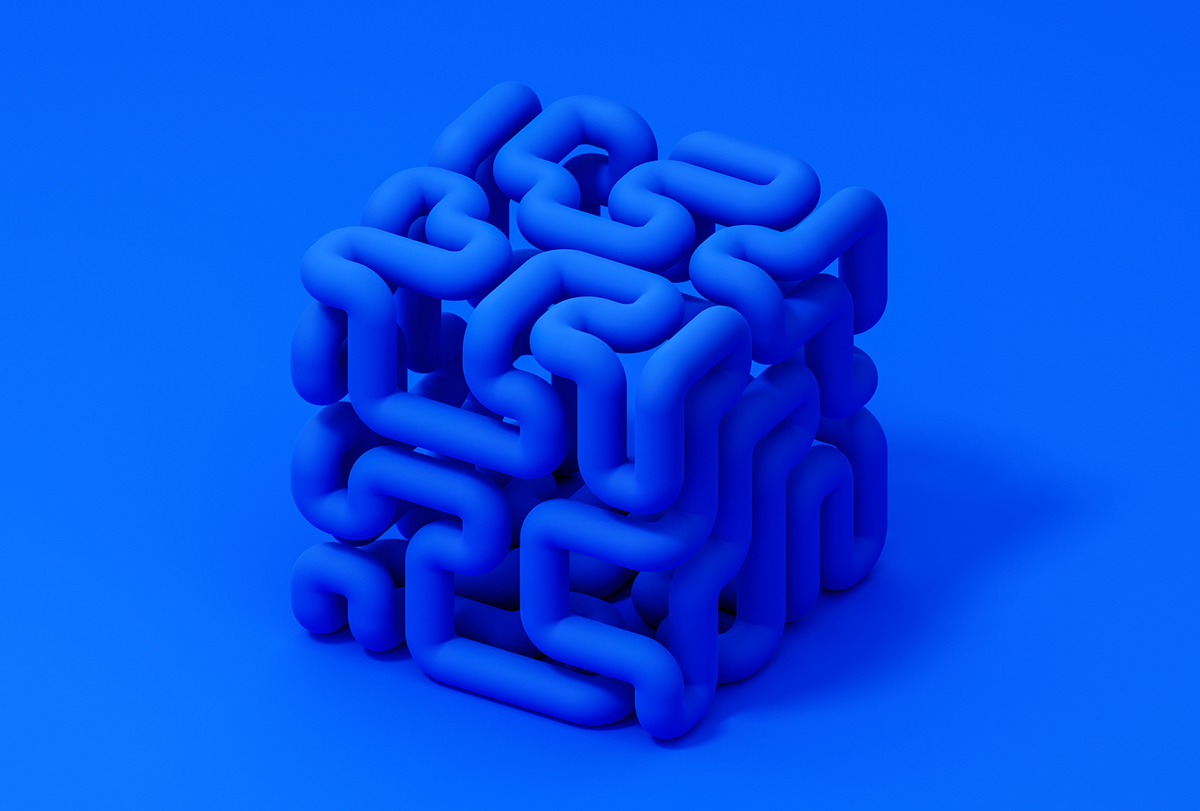
Thinking about thinking: AI offers theoretical insights into human memory
We need a new conceptual framework for understanding cognitive functions—particularly how globally distributed brain states are formed and maintained for hours.
Reading and language, those most human of cognitive functions, have long been the domain of cognitive science. But new technologies for high-dimensional recording directly from the human brain are making it possible to study the neural coding of language and other human-specific cognitive functions. Making sense of the complex activity tied to these processes requires new theoretical approaches—and insights from artificial intelligence.
As you read this article, your eyes make fast, saccadic movements across the page, taking in small groups of words in your fovea three times per second. Each saccade is a snapshot that must be integrated with all the previous words, building up a conceptual understanding of what is being conveyed. After reading this article, your brain will think about it in the context of experiences and thoughts previously stored in long-term memory. Thinking is generative. Thinking underlies planning future actions. Thinking is fleeting, constantly coming and going.
The timescale for these cognitive functions is minutes to hours, much longer than well-studied sensorimotor actions that last seconds. Researchers have developed conceptual frameworks for interpreting neural activity for fast automatized actions, correlating firing rates of sensory and motor neurons with sensory perception and behavior. But this approach doesn’t work when analyzing neural population activity over much longer timescales that are not directly related to behavior. As a field, we know much less about the fundamental neural mechanisms that underlie thinking, planning and reasoning. We need a new conceptual framework for how globally distributed brain states are formed and maintained for hours.
Linking written words across many sentences or spoken words during a long lecture, for example, requires temporal context to relate a new word to previous words. How do brains encode temporal context over hours? Long-term working memory, which supports longer-term cognitive functions, likely plays a role. Long-term working memory receives and maintains sensory inputs, using them for cognitive processing over hours, intermediate between short-term and long-term memory.
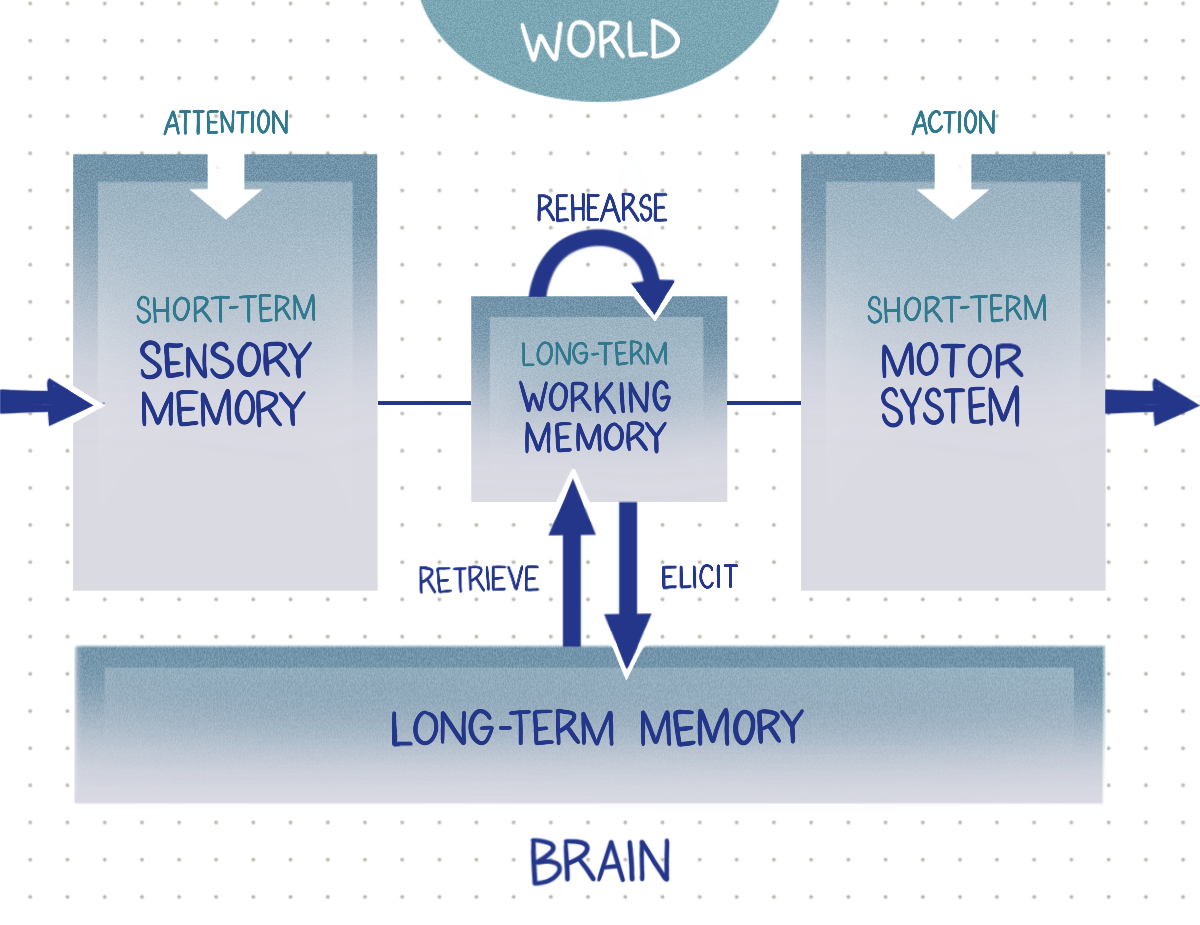
How does long-term working memory implement temporal context? How is long-term working memory used to generate cognitive behaviors such as thinking and planning? How are fleeting thoughts and temporary plans converted to overt behaviors?
AI may offer insight into temporal context. Like humans, large language models track information about the sequence of words across many sentences and paragraphs and use temporal context to link these words semantically. I argue here that generative transformers, a key architectural feature of many large language models, demonstrate how neural networks can create temporal context and that a similar process is at work in biological brains. I will make the case that temporal context, implemented by transformers in a spatially static way, is implemented dynamically in brains.
T
ransformers are feedforward neural networks that comprise an encoder, which receives queries, and a decoder, which outputs words in English, one word at a time. Each output word is looped back to the input of the decoder. As each word is produced on the output layer, it is added to a long input vector, providing a comprehensive temporal context for predicting the next word. Transformers, therefore, convert temporal sequences into a spatial sequence that gives the feedforward network access to all the words at the same time. Traveling waves of sparse cortical activity offer a candidate dynamical mechanism for temporal context in brains. Like the transformers in large language models, a traveling wave recodes input sequences into spatial patterns and extends working memory across the cortex.Traveling waves were first observed 100 years ago and are especially prominent in the cerebral cortex, but we know little about what they do. Cortical traveling waves, which can be triggered by sensory inputs, are sparse, with only a few percent of the cortical neurons activated by a passing wave. Scientists can visualize them with voltage-sensitive dyes. Unlike simulations of dense traveling waves in small network models, in which all the neurons spike as the wave goes by, sparse traveling waves require large recurrent neural network models with 100,000 spiking neurons with the same connectivity as the cortex.
Traveling wave electrical activity in recurrent networks can extend working memory with rehearsal up to a minute. But some other mechanism is needed to extend timescales from a minute to hours. A promising candidate is spike-timing-dependent plasticity (STDP), a form of plasticity that requires repetitive, precisely timed pairings of pre- and postsynaptic spikes within 10 milliseconds of each other at frequencies above 10 hertz. In cortical slice experiments, spike pairings repeated 50 or more times induce enduring changes in synaptic strength. With fewer pairings, however, the change in strength fades away over minutes and hours, depending on the number and frequency of pairings. STDP is generally considered a synaptic mechanism for forming long-term memories. Instead, I propose that STDP mainly supports temporary working memories.
STDP can rapidly change the strength of cortical synapses, the vast majority of which are small and labile. These changes could support a temporary working memory complementary to the fewer but larger, more stable synapses that store long-term memories and are used for fast sensorimotor processing. Although synaptic plasticity in these small synapses may be temporary, their capacity is immense. The induction phase of synaptic plasticity triggered by waves traveling across the cortex is like a palimpsest that can be rewritten many times and could support a “global workspace,” a leading model for conscious awareness that posits that information is shared across cortical regions. Traveling waves could accomplish this by rapidly assembling a second tier of global connectivity on top of the first tier, which supports more automatized sensorimotor behaviors. These two tiers are complementary, and their relationship is somewhat analogous to classical mechanics and quantum mechanics, in which a collapse of a quantum wave packet corresponds to a decision to recruit the motor system.
I suggest that together, these two aspects of cortical physiology—traveling waves, which are ubiquitous but lack a well-established function, and STDP, which is well established but thought to be the basis of long-term memory—are responsible for long-term working memory and underlie some aspects of thinking and cognitive processing. These two neural mechanisms can interact because the frequencies of cortical traveling waves match the frequencies of pairing required for STDP. In this new conceptual framework, STDP is induced temporarily by precisely timed wavefronts. Spontaneous traveling waves are also ubiquitous and could be used to recall long-term memories and rehearse fading working memories.
The grid of large synapses supporting long-term memories for the first tier provides high-speed highways for cortical activity called mathematical manifolds in the second tier. The vehicles on the road are not single passengers but trucks that carry bundles of associated items called a schema, or a structured plan.
These are a few key pieces of the thinking puzzle. The Humpty Dumpty challenge is to put all the pieces together again, including pieces still left out. We still need to incorporate the roles of inhibitory neurons and neuromodulators, for example. But these potential insights from transformers demonstrate NeuroAI’s new conceptual framework for understanding cortical function.
Recommended reading
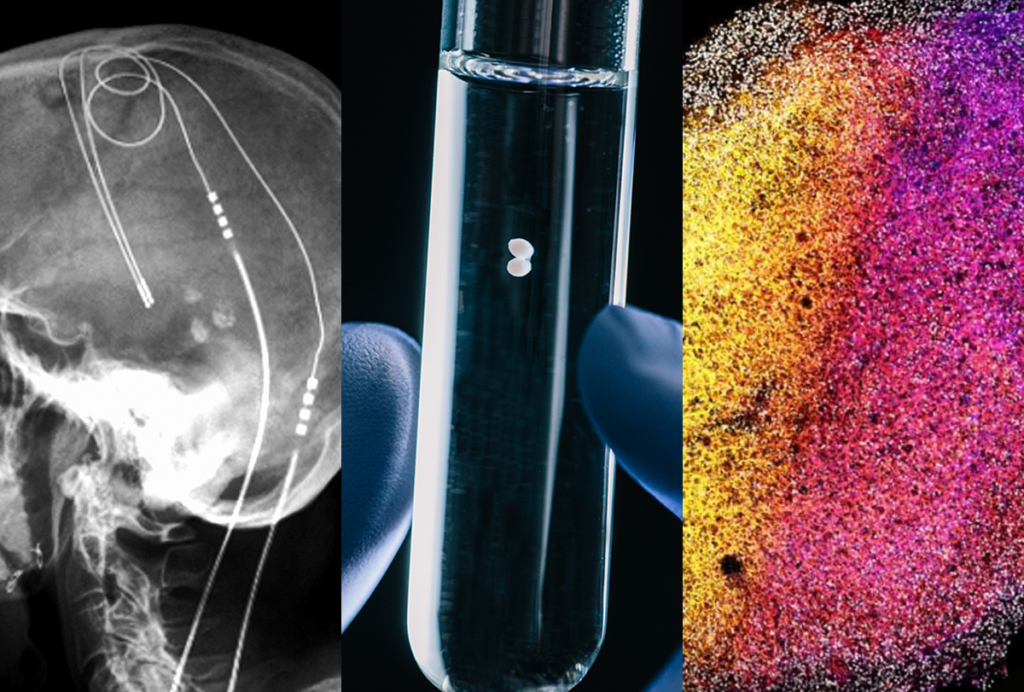
Why the 21st-century neuroscientist needs to be neuroethically engaged
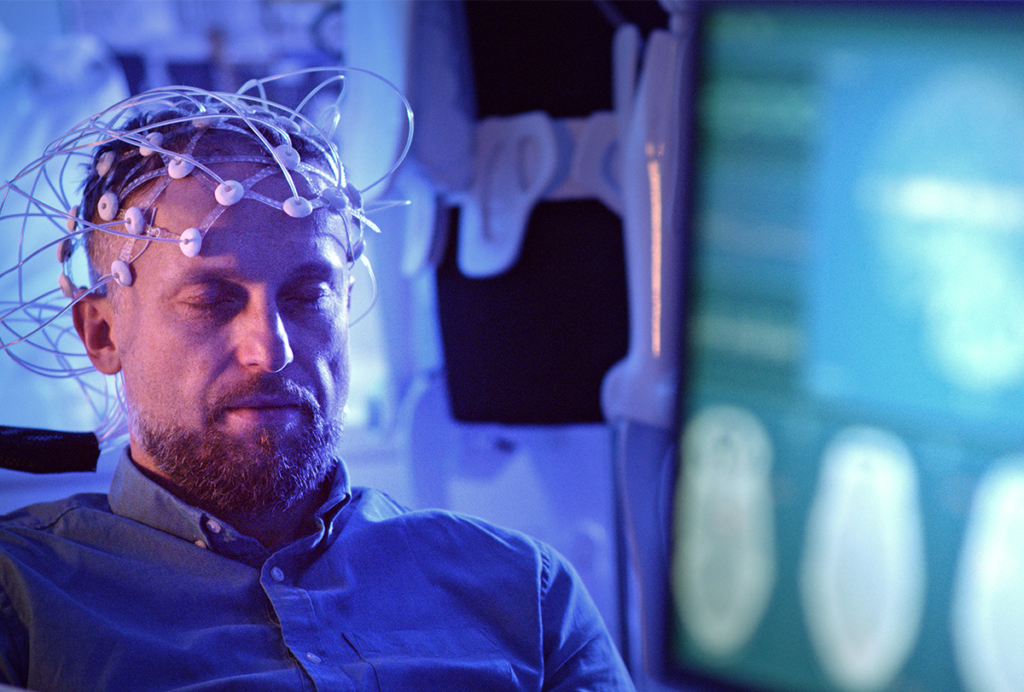
Noninvasive technologies can map and target human brain with unprecedented precision
Explore more from The Transmitter
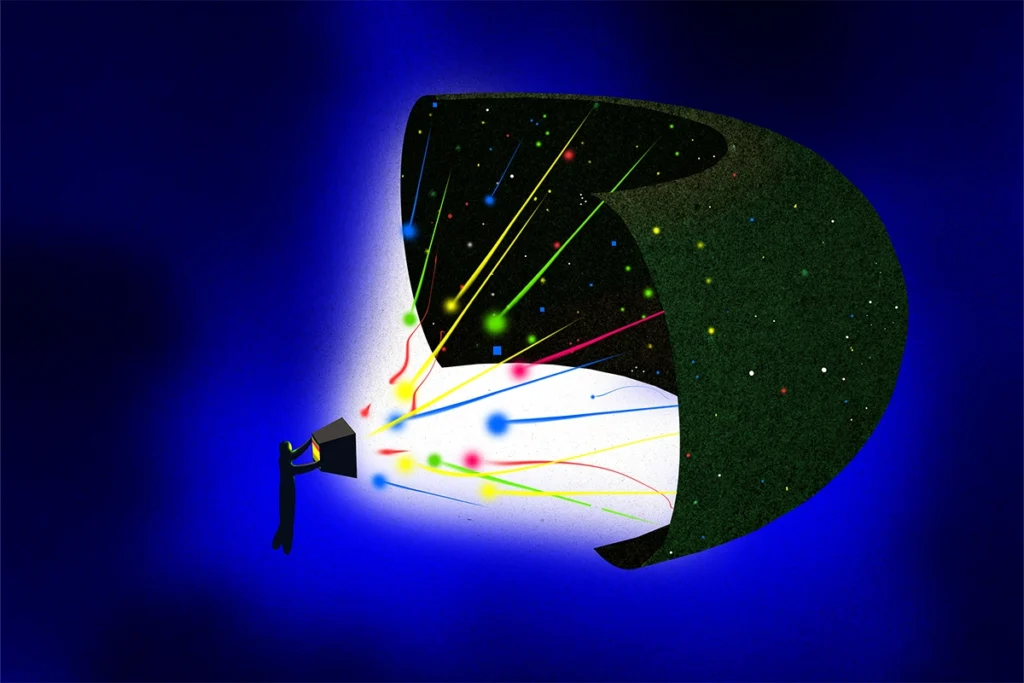
Imagining the ultimate systems neuroscience paper
Grace Hwang and Joe Monaco discuss the future of NeuroAI
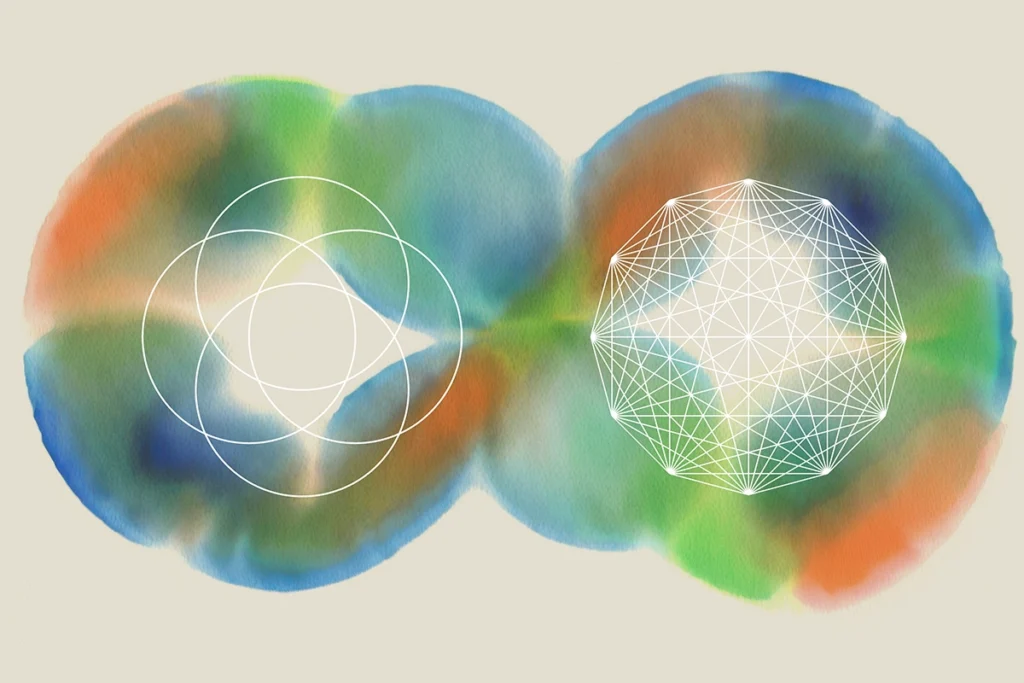