Simply making data publicly available isn’t enough. We need to make it easy — that requires community buy-in.
I helped create a standard to make it easy to upload, analyze and compare functional MRI data. An ecosystem of tools has since grown up around it, boosting reproducibility and speeding up research.
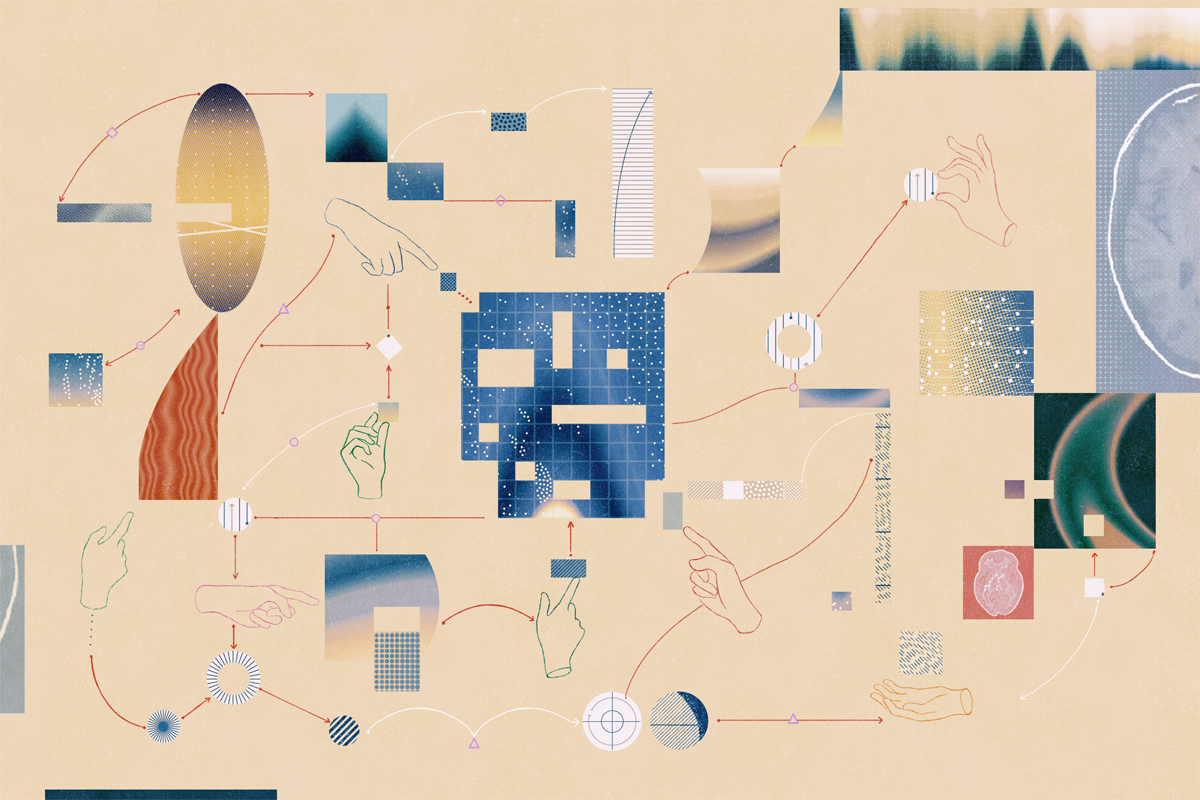
The sharing of data collected in scientific studies is increasingly viewed as an important way to make science better. It helps maximize the benefits that accrue from the data (which are often collected using taxpayer funds); it enables larger studies by aggregating across many smaller datasets; and it enables researchers to check the results from published papers to see if they hold up, using the same or different analysis methods.
But shared data provide such benefits only if they are organized in such a way that other researchers can use them effectively. A humorous YouTube video, “Data Sharing and Management Snafu in 3 Short Acts,” by the NYU Health Sciences Library in New York City, demonstrates how data-sharing can go wrong. In this animated video, one researcher (played by a sad panda) requests data from another researcher from their paper recently published in the journal Science. After much back and forth, the researcher finally provides the data, leading to the following exchange:
– I received the data, but when I opened it up it was in hexadecimal [an indecipherable digital format].
– Yes, that is right.
– I cannot read hexadecimal.
– You asked for my data, and I gave it to you. I have done what you asked.
My group has experienced firsthand the importance of data organization. About a decade ago, inspired by Michael Milham and his colleagues in the 1000 Functional Connectomes Project, we started sharing brain imaging data through a project called OpenfMRI. In that early project, researchers sent us datasets to be shared, and a data curator in our group had to reorganize their data to match our in-house organization scheme. This reorganization often required extensive back-and-forth between the curator and the owner of the data.
In 2015, we received funding from the Laura and John Arnold Foundation to expand this project, which ultimately became the OpenNeuro data archive, an open platform for sharing magnetic resonance imaging (MRI), positron emission tomography (PET), magnetoencephalography (MEG) and electroencephalogram (EEG) data. Because we wanted to be able to accept data broadly without requiring a large team of curators, we decided to develop a data organization standard that any researcher could use, enabling them to upload their data without the need for human curation. We realized that this would be successful only if we got many researchers in our community on board, so we worked with a large number of people to develop a new framework that we called the Brain Imaging Data Structure (BIDS). It took about a year to develop the first version of BIDS, which was published in the journal Scientific Data in 2016.
So what is BIDS, exactly? It’s really two things. First, it’s a scheme for naming and organizing the many files that make up a brain imaging dataset. My lab uses MRI. BIDS tells us how to name the files that are generated during brain imaging experiments and how to set up the folders that the different kinds of data will go into. BIDS also provides a scheme for how to organize the metadata that describe how the data were collected. Each image file in a BIDS dataset has an associated file that contains detailed information about how the image was collected.
Importantly, BIDS specifies the vocabulary that can be used to name each parameter. For example, “repetition time” is an important variable in MRI experiments, and in the literature it is referred to in many different ways — “Repetition Time,” “RT” and “TR,” for example — and can be expressed in seconds or milliseconds. BIDS dictates the specific term used to define this value in the metadata (“RepetitionTime”), as well as the units (seconds). To anyone who isn’t an MRI aficionado, this level of detail probably sounds immensely boring, but it turns out to be essential if humans or machines are to read MRI datasets without any ambiguity about how the data were generated.
P
art of BIDS’ success stems from its strong community-driven character: Decision-making is led by an elected steering group, and its ongoing development and maintenance is supported by a group of nine volunteers, along with many other contributors. This kind of community organization requires a lot of time and effort and a willingness to compromise for the greater good.The BIDS community has grown to be very large, with several hundred researchers having contributed to the effort in some way. By various estimates, there are hundreds of thousands of datasets in the wild that have been converted into the BIDS format, with data from more than 30,000 people available via the OpenNeuro archive alone. The ease of reusing a BIDS dataset has led to many published reuses, helping to maximize the benefits of those data for the community and the world.
In one notable study designed to assess reproducibility, 70 groups of researchers analyzed the same large functional MRI (fMRI) dataset distributed in the BIDS format. The results varied widely depending on analysis workflows, highlighting the need to understand how analytic variability affects scientific results. BIDS made it possible for each of the participating groups to take the dataset and immediately understand how to process it; without BIDS, the degree of communication required to explain the data would have been overwhelming for such a large number of groups.
Yet another great benefit of BIDS is the ecosystem of tools that has grown around it. This suite of community-generated “BIDS Apps” makes it easy to process the data in various ways. These apps enable users to take commonly used imaging analysis software, such as the FreeSurfer tool for anatomical processing, and easily apply it to their BIDS dataset, rather than having to reformat the data to meet the distinct requirements of each software package. One such BIDS App for the preprocessing of fMRI data, fMRIPrep, has become remarkably popular, with several thousand uses each week over the past year. Because BIDS Apps are packaged with all of the required additional software libraries, they also provide a greater degree of reproducibility across different computer platforms.
BIDS also has a defined process for extensions into new data types, which supports growth into new communities. Community members can propose extensions that can then be developed through discussion with the maintainers and the steering group. This process has led to support for many new data types, ensuring the continued growth and relevance of the standard, and demonstrates the strength of the community model for data standards.
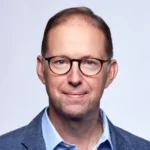
Explore more from The Transmitter
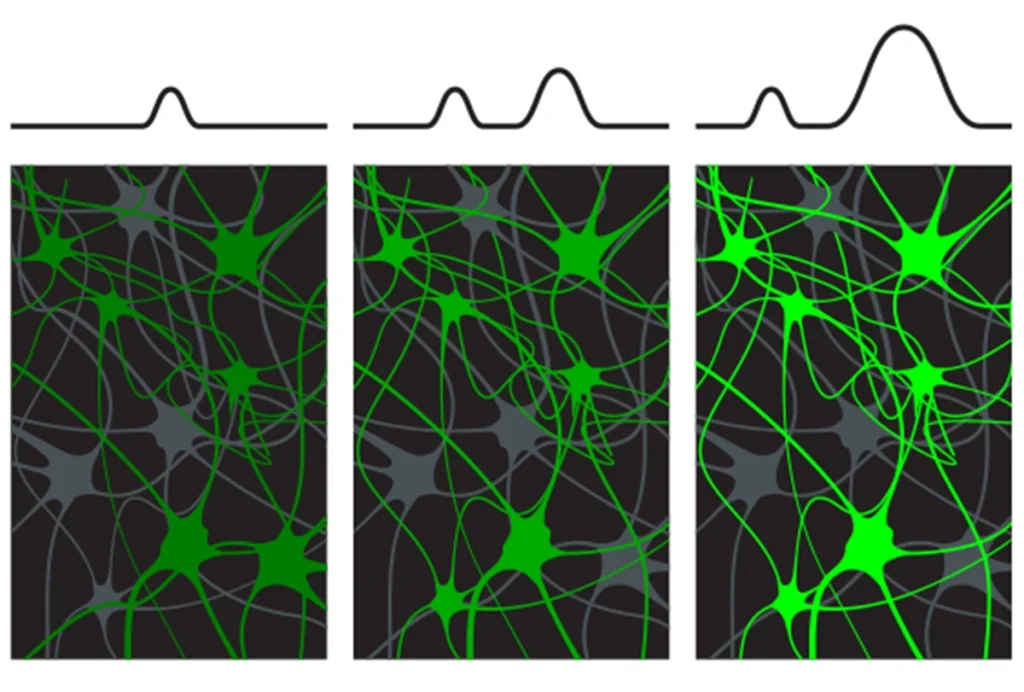
Cocaine, morphine commandeer neurons normally activated by food, water in mice
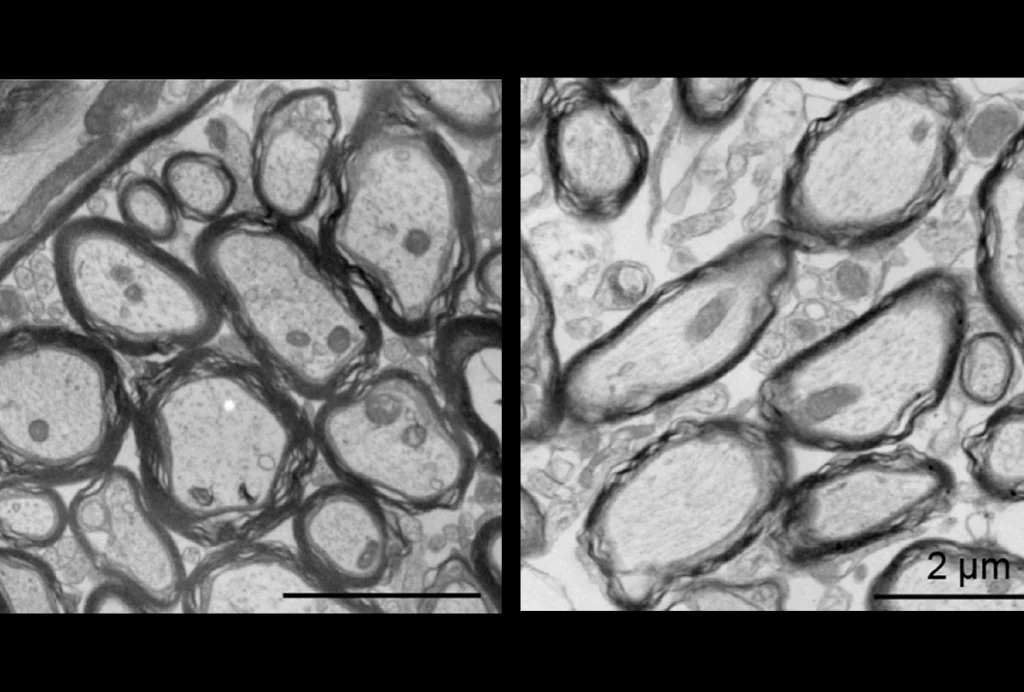
X chromosome inactivation; motor difficulties in 16p11.2 duplication and deletion; oligodendroglia
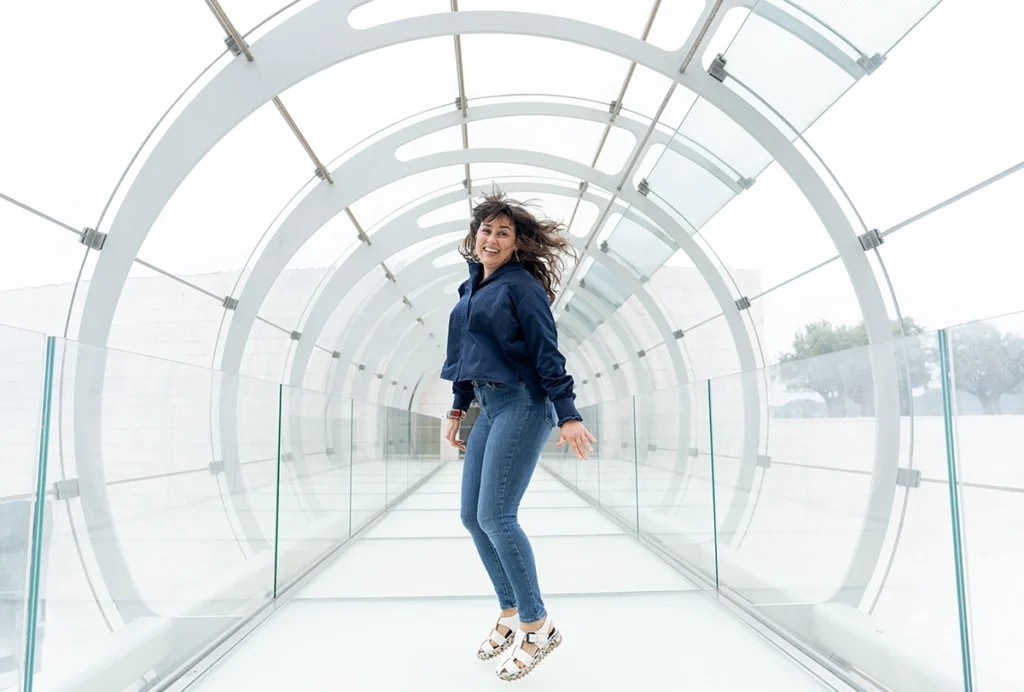