
Study seeks autism biomarkers in brain-imaging database
A large, multisite dataset of brain scans identifies autism with 60 percent accuracy, much lower than the numbers cited by single-site studies. The study, published 25 September in Frontiers in Human Neuroscience, highlights the vast differences in equipment, quality and methods across sites.
A large, multisite dataset of brain scans identifies autism with 60 percent accuracy1, much lower than the 80 percent accuracy or higher cited by single-site studies2, 3. The study, published 25 September in Frontiers in Human Neuroscience, highlights the vast differences in equipment, quality and methods across sites.
It also points to the limitations of pooled datasets, which, despite their large size, must account for these differences.
“It’s a much more difficult problem when you expand to multisite data,” says lead investigator Jeffrey Anderson, assistant professor of neuroradiology at the University of Utah.
Still, Anderson and others say they are optimistic about the potential of multisite datasets to power large studies, and to define subgroups of autism.
The new study, the first attempt at identifying autism using such a dataset, offers intriguing hints about which brain areas may be particularly important in identifying the disorder. Learning from its limitations may also help researchers improve multisite studies, he says.
The study drew its data from the Autism Brain Imaging Database Exchange (ABIDE), an open-access database fed by more than 16 labs worldwide. Together, the labs contribute functional magnetic resonance imaging (fMRI) scans and diagnostic and demographic data from more than 1,000 people with autism and controls, aged 7 to 64 years.
For this study, the researchers used data from 964 individuals whose brain images meet specific criteria, including 517 typically developing people and 447 people with autism. They analyzed the brain scans, accounting for head motion, heartbeat and other potential sources of error.
The team focused their attention on 7,266 separate regions in the cortex for each brain scan. They traced a total of 26.4 million connections between pairs of these regions, aiming to test the functional connectivity theory of autism. This theory holds that connections in the brains of people with autism differ from those of controls.
Sorting scans:
The researchers compared each individual’s connectivity with that of the other participants. This, along with data about age and handedness, generated an individual’s classification score: The higher the score, the greater the likelihood of autism.
Averaged across all of the ABIDE sites, the researchers were able to correctly identify individuals as having autism 60 percent of the time.
“I was hoping it would be higher,” says Anderson. He says he had anticipated that having more data to weed out poor connections might do more to outweigh the noise in the data than it did.
“I find [the study] an exciting initial step,” says Adriana Di Martino, assistant professor of child and adolescent psychiatry at New York University’s Langone Medical Center. Di Martino co-founded the ABIDE database but was not directly involved in the new study. “It’s highlighting that there’s much work that needs to be done to use fMRI to classify individuals with autism,” she says.
In addition to looking at accuracy, the scientists pinpointed connections that were particularly important in distinguishing the brain of someone with autism. “We could start to get a feel for what kinds of connections or what places were abnormal,” Anderson says.
For example, the researchers found that the default network, the insula and the fusiform gyrus, which have all been implicated in autism, differ between the two groups. This finding corroborates the results of an earlier ABIDE study, as well as those from some single-site studies.
To improve the usefulness of multisite studies, researchers must first address the variability across the sites, Anderson says. For instance, the classification accuracy at a given ABIDE site is strongly related to how long the scans last at that site.
“Most of these scans were only five or ten minutes,” says Anderson. Longer imaging times — up to an hour — could yield much better results, even if split over multiple sessions, he says.
Other sources of variation must also be addressed, experts say.
For example, studies should take into account the wide range in participants’ ages and the different models and magnet strengths of MRI machines, says Vinod Menon, professor of psychiatry and behavioral sciences at Stanford University in California, who was not involved in the study. “Those are very much open questions.”
Despite these limitations, Menon says, “This is the first study that has done the analysis across data from all the available sites.” It makes progress, he says, particularly in identifying “hotspots” of difference between autism and controls, such as the insula.
Multisite brain-imaging datasets such as ABIDE provide far greater statistical power than single-lab studies. They also offer the ability to focus on subgroups of the population or subtypes of autism that might not be well represented in smaller samples, notes Anderson.
Because of this, researchers are trying to find ways to overcome the limitations of such datasets, Anderson says.
“I think most of the interesting work is going to come out of these large datasets, because there’s so much you can do with them,” Anderson says. “Despite the limitations, I really prefer working with more data.”
References:
1: Nielsen J.A. et al. Front. Hum. Neurosci. 7, 599 (2013) PubMed
2: Anderson J.S. et al. Brain 134, 3742-3754 (2011) PubMed
3: Uddin L.Q. et al. Biol. Psychiatry 70, 833-841 (2011) PubMed
Recommended reading
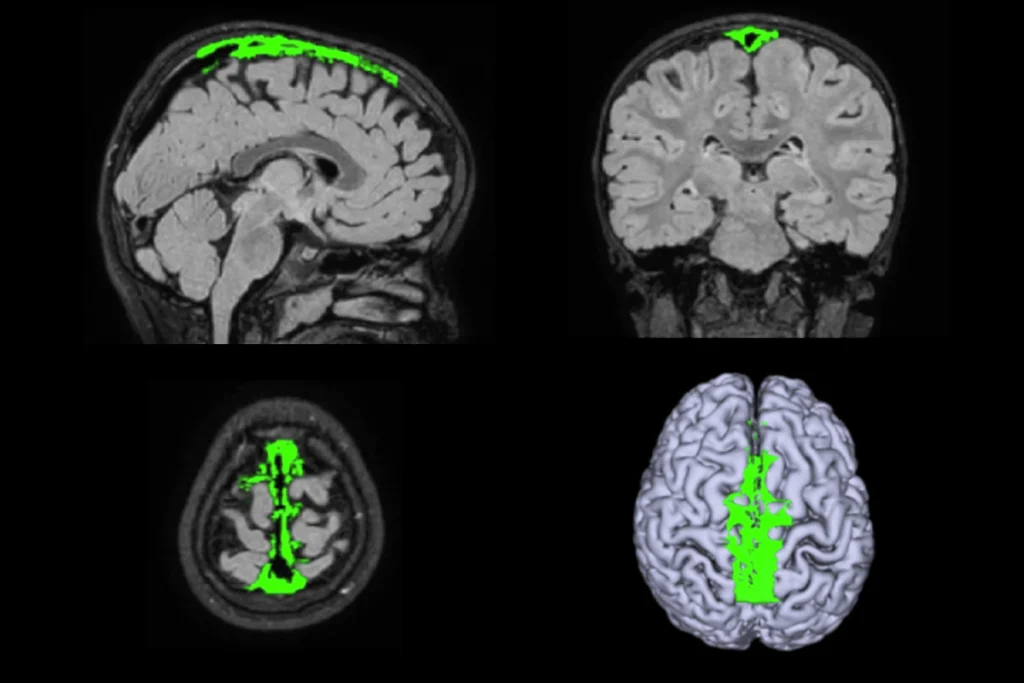
Okur-Chung neurodevelopmental syndrome; excess CSF; autistic girls
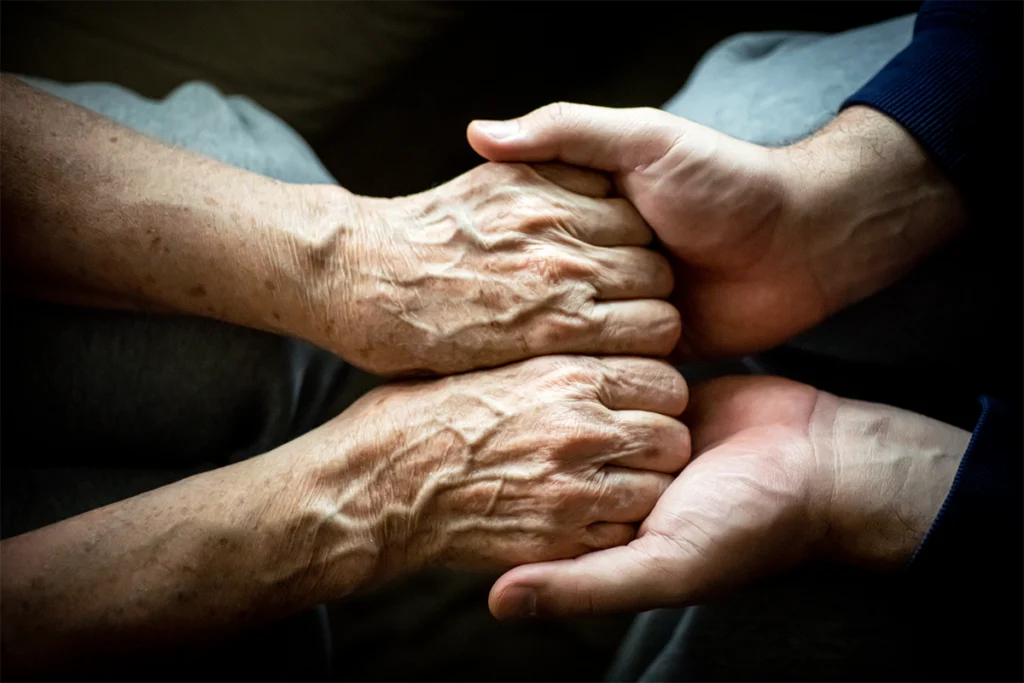
New catalog charts familial ties from autism to 90 other conditions
Explore more from The Transmitter
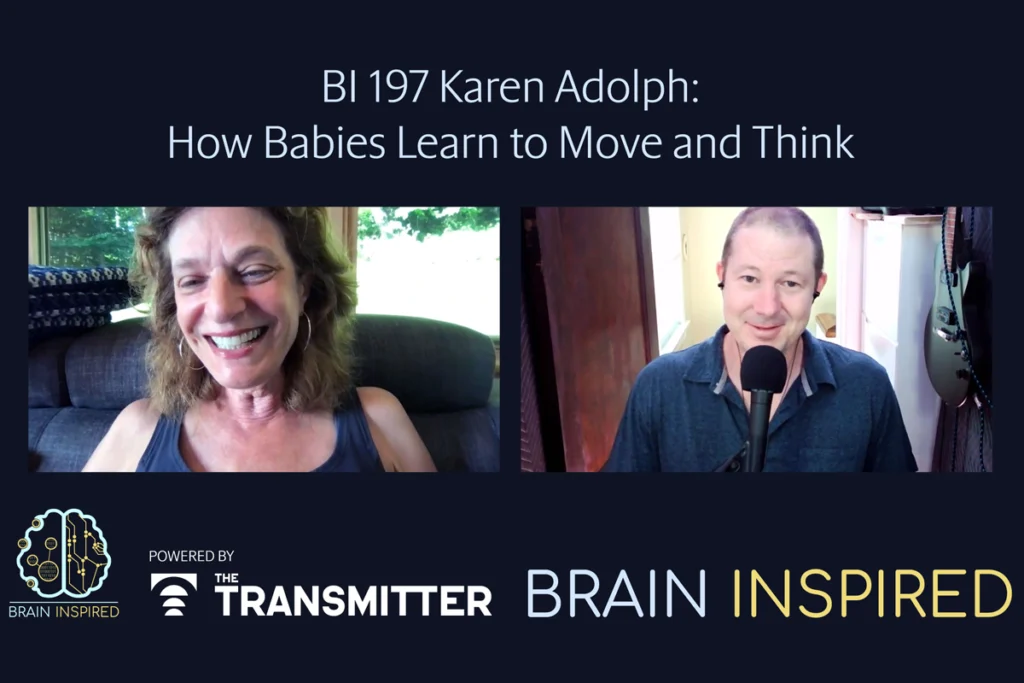
Karen Adolph explains how we develop our ability to move through the world
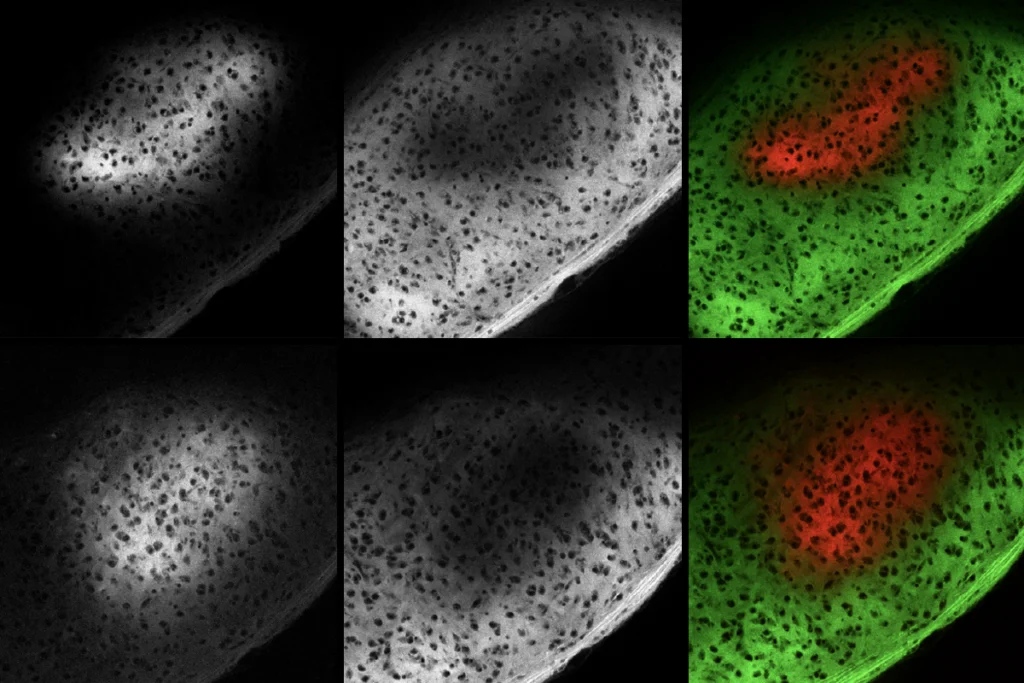
Microglia’s pruning function called into question
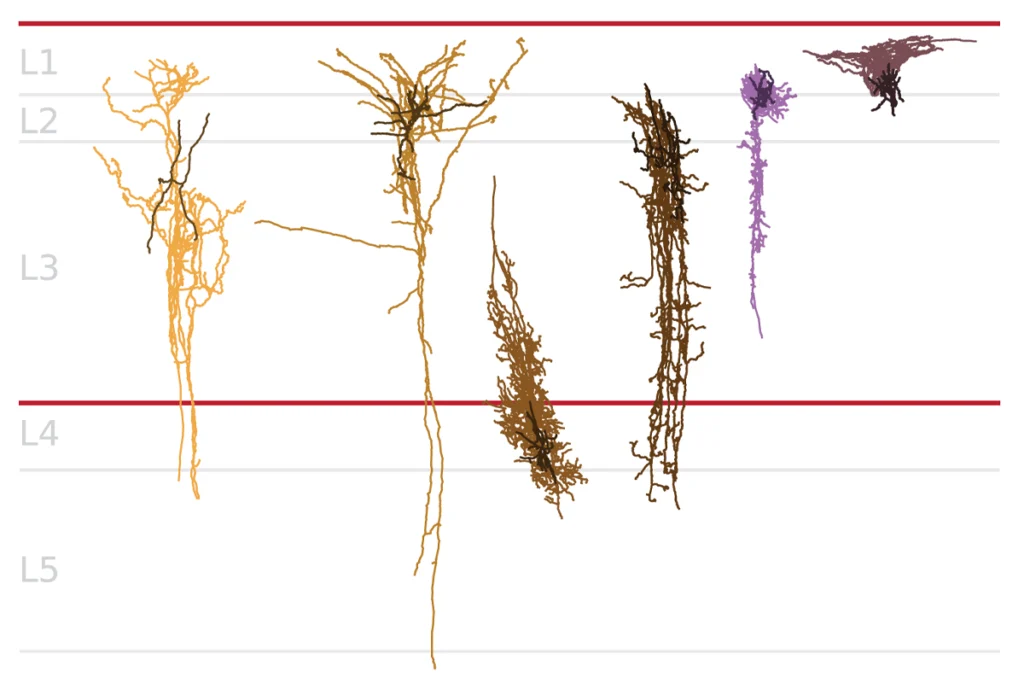