Diverse data networks point to driving force in diseases
A mathematical approach called ‘NEW biology,’ or network-enabled wisdom biology, aims to solve one of the biggest problems in disease research: isolating the key factors that drive diseases from a glut of information.
The walls of the newly launched Institute for Genomics and Multiscale Biology at Mount Sinai School of Medicine in New York still look fresh and bare, deceptively so, given the hubbub behind them. A rapidly growing sequencing center is being built down the hall, and the institute has lured a number of high-profile researchers to its ranks.
These swelling resources are largely in the service of a concept called ‘NEW biology,’ or network-enabled wisdom biology, a mathematical approach to solving one of the biggest problems in disease research: isolating the key factors that drive diseases from a glut of information1.
Research into complex disorders is generating an increasingly large amount of diverse data, be it from studies of gene expression, genetic risk variants, brain imaging or behavior.
Analyzing these data by building mathematical network models has shown promise in identifying genes involved in obesity, sleep and cancer. Researchers are beginning to apply this approach to neuropsychiatric disorders, including autism.
Eric Schadt, director of the institute and a pioneer of this approach, says he believes it will help find hidden gold in existing datasets, such as those from genome-wide association studies (GWAS).
“New technologies enable us to generate huge volumes of data, which drives a new methodology to interpret that data,” says Joshua Millstein, Schadt’s collaborator and assistant professor of biostatistics at the University of Southern California’s Keck School of Medicine in Los Angeles. “The challenge is whether we can use the data-driven approach to understand biology.”
Building networks:
Scientists have been integrating different types of large-scale data for more than a decade, and early efforts have helped to identify genes and molecular networks associated with a broad range of diseases, including autism.
But most ongoing studies analyze different types of data separately and then integrate those results, says Schadt. Newer approaches handle diverse data simultaneously to get at the best possible interpretation, he says.
Picking out the hubs in these data networks can help scientists select genetic variants that are most relevant to a particular disease, in contrast with those that have little effect. That is especially important in large-scale sequencing and other studies, which can identify hundreds or even thousands of mutations of unknown significance.
“If you’re going to war, you want to know who the general is rather than the troops fighting the war,” says Andrea Califano, professor of biomedical informatics at Columbia University in New York. Califano is also developing new methods for analyzing multilevel biological data, and is collaborating on several projects with Schadt.
In step with the growing size of datasets, Schadt’s approach, technically called ‘Bayesian probabilistic causal network reasoning,’ requires intense computation and supercomputing resources.
Researchers first build a network using one set of data, determining links between individual data points by looking for correlations, such as two genes whose expression levels appear to fluctuate in tandem.
To determine which molecular factors drive the network, they add another set of data, such as genetic variants. Because genetic variation exists before the onset of disease, researchers can measure how a specific mutation alters the network and infer the directionality of the correlation: whether factor A influences factor B or vice versa. That allows researchers to pinpoint which genes are driving changes to the network.
“Causality is critical if you want to ask which gene is responsible for the phenotype,” says Califano. “Otherwise you can get hundreds of genes and it’s almost impossible to figure out [which gene] did what.”
The idea is similar to knocking out a gene in a mouse and then observing the downstream effects, but on a larger and more complex scale.
“DNA variation serves as a source of perturbation,” says Schadt. “You can use it to start directing which way information is flowing in the network.”
The more data used to build the network, the more predictive the model.
Mapping disease:
In a study submitted for publication, Schadt and his collaborators used this approach to analyze data collected from four different studies of breast cancer, comprising about 1,000 people. They identified several sub-networks involved in molecular pathways associated with the disease, such as the cell cycle.
Because the researchers used a type of analysis that determines the direction of the correlation, they were able to identify key drivers in the network.
The researchers categorized genes as either ‘global drivers’ — those that control the network’s state — or ‘non-drivers,’ which merely respond to the drivers. (A third class of local drivers can modulate some genes and be regulated by others.)
To verify their initial findings, the researchers looked for mutations in global driver genes in a separate set of 75 individuals with breast cancer. They found that mutations in a subset of the global drivers are 100-fold more common than those in non-drivers.
Schadt’s team is applying this approach to two neurodevelopmental disorders that have proven particularly difficult to unravel: autism and schizophrenia.
“Data coming from the neuro space is now on a big enough scale to have a real shot at coming up with good models,” he says.
The researchers plan to start by analyzing tissue from the Mount Sinai brain bank and the Autism Tissue Program, as well as data from Daniel Geschwind‘s laboratory at the University of California, Los Angeles.
Geschwind’s team has analyzed gene expression in postmortem brain tissue from people with autism.
In collaboration with Raquel Gur, professor of psychiatry at the University of Pennsylvania, Schadt is also analyzing data from an ongoing study, funded by the National Institute of Mental Health, of 10,000 young people aged 8 to 21 with variety of disorders.
Participants have already had their genomes analyzed. Researchers at Children’s Hospital of Philadelphia are collecting data on attention, mood, anxiety and cognitive and emotional processing, via computerized questionnaires, and brain imaging data from 2,000 people in the study.
“All this multilevel information will enable us to look at the topography of the brain as it relates to these quantitative measures and to genomics,” says Gur.
Schadt says his approach will also help liberate valuable information hidden in GWAS studies, which search for common genetic variants linked to diseases. Many experts criticize these studies for their failure to detect a high percentage of the heritability of common diseases, despite analyzing data, in some cases, from tens of thousands of people.
Part of the problem with GWAS studies is that because a single study includes so many tests, researchers impose strict statistical criteria to correct for multiple testing errors.
Schadt says this means that genes that have a small effect are lost in the noise. He instead proposes using data from gene expression studies to narrow down the most likely suspects in GWAS studies2.
Despite the dire need for new types of analyses, NEW biology is still a work in progress and many caveats remain.
“The technology is still way ahead of the methodology,” says Millstein. “Because the methodology is young, there is a lot of discussion and a lot of development in the area. I don’t think there is consensus yet on the right or wrong way.”
References:
1: Schadt E.E. and J.L. Björkegren Sci. Transl. Med. 4, 115rv1 (2012) PubMed
2: Zhong H. et al. PLoS Genet. 6, e1000932 (2010) PubMed
Recommended reading
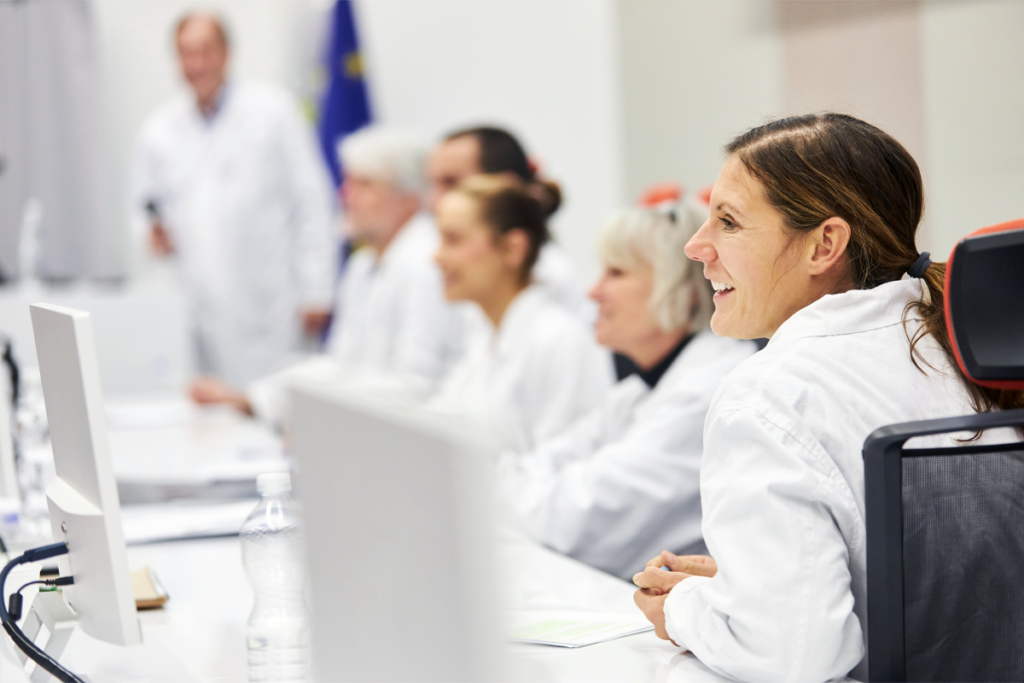
INSAR takes ‘intentional break’ from annual summer webinar series
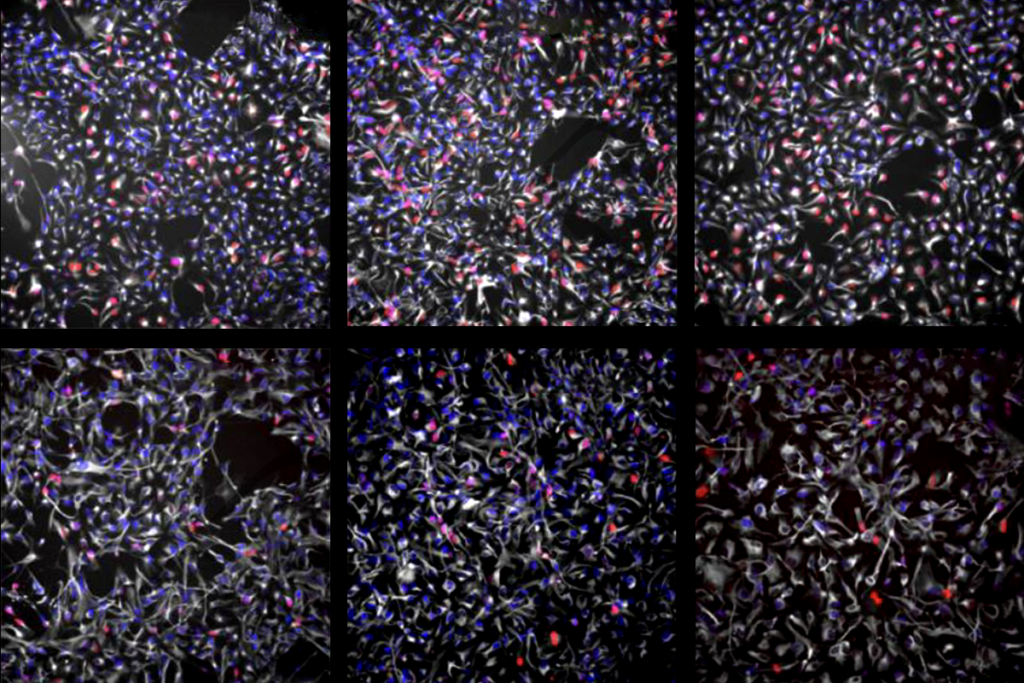
Dosage of X or Y chromosome relates to distinct outcomes; and more
Explore more from The Transmitter
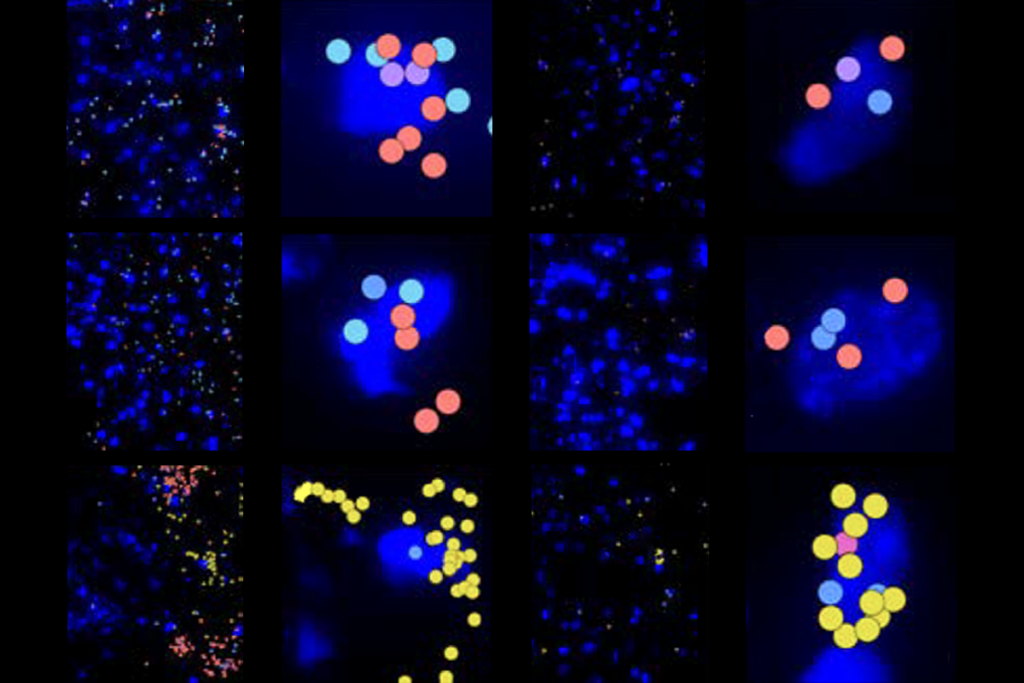
Machine learning spots neural progenitors in adult human brains
Xiao-Jing Wang outlines the future of theoretical neuroscience
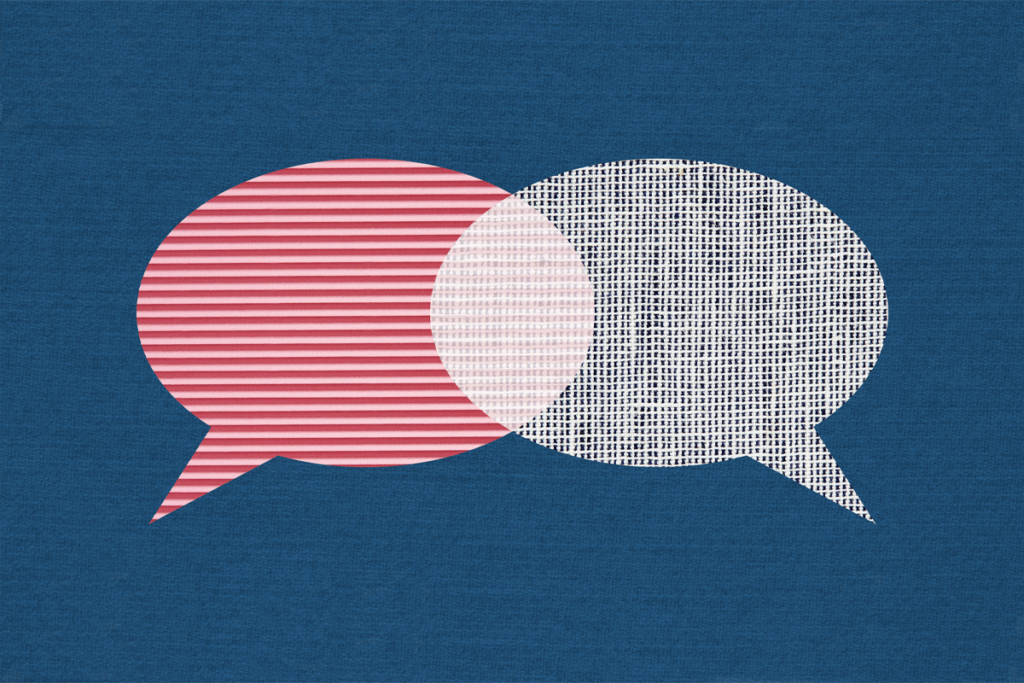